Neural Constraint Inference: Inferring Energy Constraints in Interacting Systems
ICLR 2023(2023)
摘要
Systems consisting of interacting agents are prevalent in the world, ranging from dynamical systems in physics to complex biological networks. To build systems which can interact robustly in the real world, it is thus important to be able to infer the precise interactions governing such systems. Existing approaches typically discover such interactions by explicitly modeling the feedforward dynamics of the trajectories. In this work, we propose Neural Constraint Inference (NCI) model as an alternative approach to discover such interactions: it discovers a set of relational constraints, represented as energy functions, which when optimized reconstruct the original trajectory. We illustrate how NCI can faithfully predict future trajectory dynamics, achieving more consistent long-rollouts than existing approaches. We show that the constraints discovered by NCI are disentangled and may be intermixed with constraints from other trajectories. Finally, we illustrate how those constraints enable the incorporation of external test-time constraints.
更多查看译文
关键词
relational inference,energy-based models,energy constraints,trajectory prediction,graph neural networks
AI 理解论文
溯源树
样例
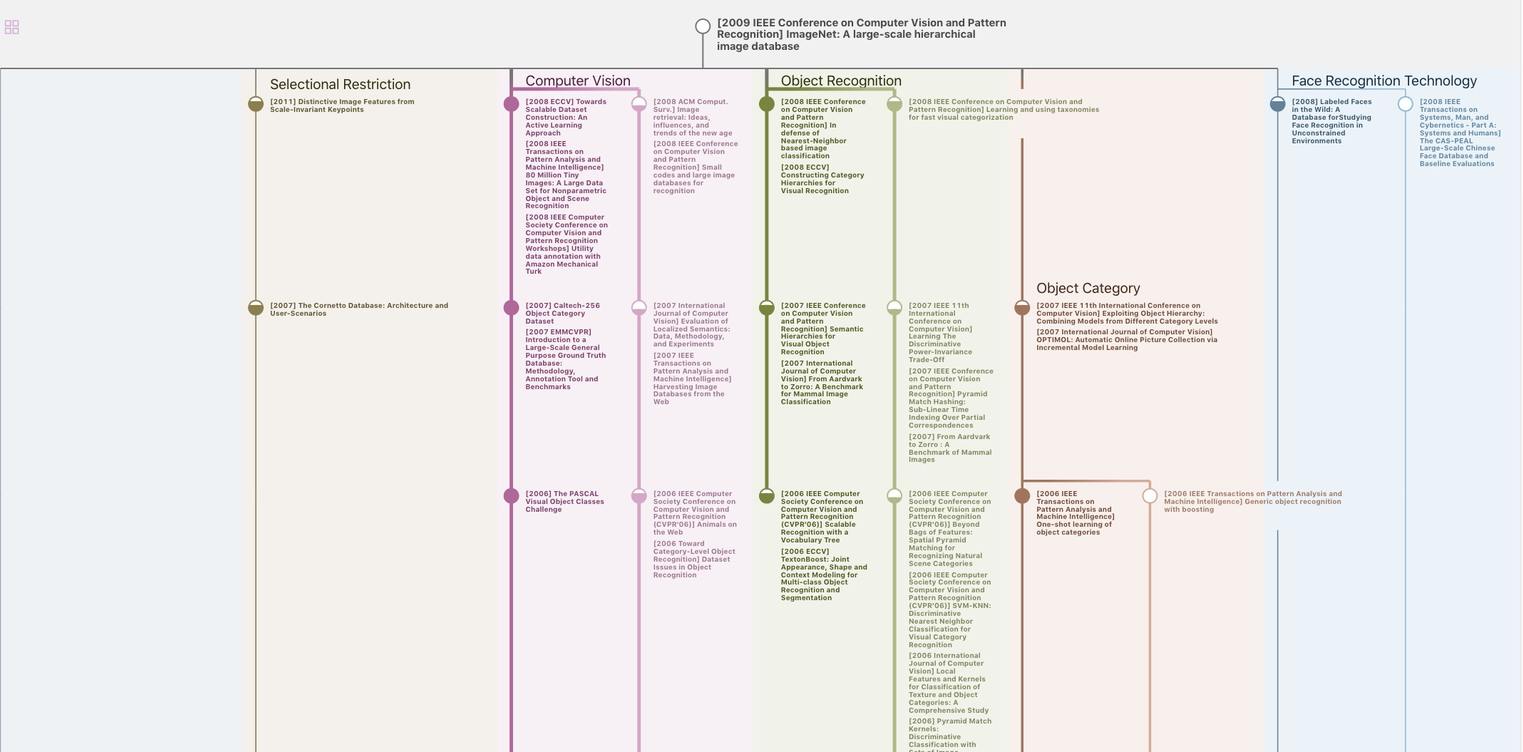
生成溯源树,研究论文发展脉络
Chat Paper
正在生成论文摘要