How Weakly Supervised Information helps Contrastive Learning
ICLR 2023(2023)
摘要
Contrastive learning has shown outstanding performances in both supervised and unsupervised learning. However, little is known about when and how weakly supervised information helps improve contrastive learning, especially from the theoretical perspective. The major challenge is that the existing theory of contrastive learning based on supervised learning frameworks failed to distinguish between supervised and unsupervised contrastive learning. Therefore, we turn to the unsupervised learning frameworks, and based on the posterior probability of labels, we translate the weakly supervised information into a similarity graph under the framework of spectral clustering. In this paper, we investigate two typical weakly supervised learning problems, noisy label learning, and semi-supervised learning, and analyze their influence on contrastive learning within a unified framework. Specifically, we analyze the effect of weakly supervised information on the augmentation graph of unsupervised contrastive learning, and consequently on its corresponding error bound. Numerical experiments are carried out to verify the theoretical findings.
更多查看译文
AI 理解论文
溯源树
样例
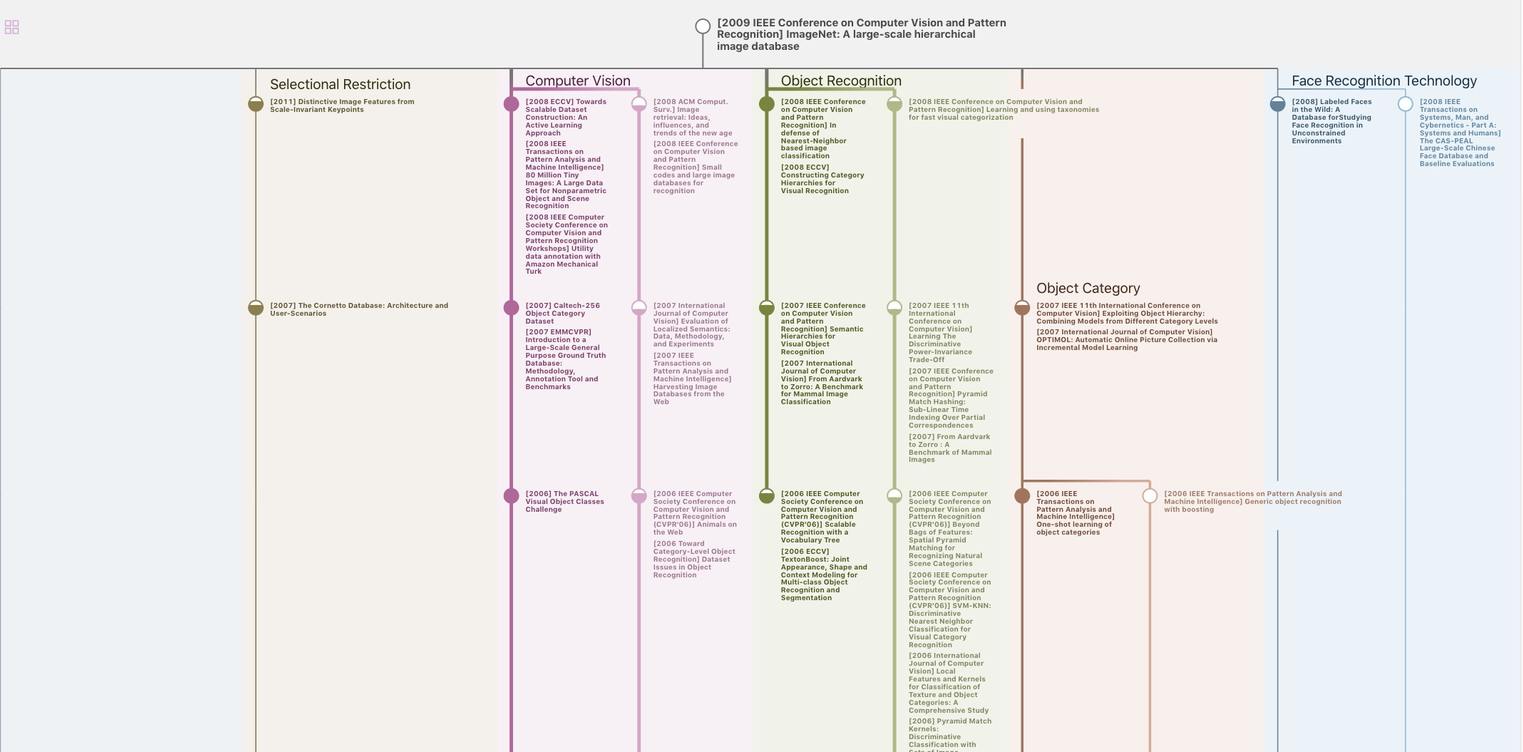
生成溯源树,研究论文发展脉络
Chat Paper
正在生成论文摘要