Bayesian Optimal Experimental Design for the Survey Bandit Setting
ICLR 2023(2023)
摘要
The contextual bandit is a classic problem in sequential decision making under uncertainty that finds broad application to tasks in precision medicine, personalized education, and drug discovery. Here, a decision maker repeatedly receives a context, takes an action, and then observes an associated outcome, with the goal of choosing actions that achieve a minimal regret. However, in many settings, the context is not given, and the decision maker must instead collect some information to infer a context before proceeding. For example, when a doctor does not have prior information about a patient, they might ask a sequence of questions before recommending a medical treatment. In this paper, we aim to develop methods for this setting—which we refer to as the \emph{survey bandit}—where the decision maker is not given access to the context but can ask a finite sequence of questions to gain information about the context before taking an action and observing an outcome. Using insights from Bayesian optimal experimental design (BOED) and decision-theoretic information theory, we view the interaction with each user as a BOED task, where the goal is to ask a sequence of questions that elicit the most information about the optimal action for this user. Our procedure is agnostic to the choice of probabilistic model, and we demonstrate its usefulness in a few common classes of distributions. Our algorithm achieves significantly better performance on both synthetic and real data relative to existing baseline methods while remaining statistically efficient, interpretable, and computationally friendly.
更多查看译文
关键词
Bayesian optimal experimental design,contextual bandit,survey
AI 理解论文
溯源树
样例
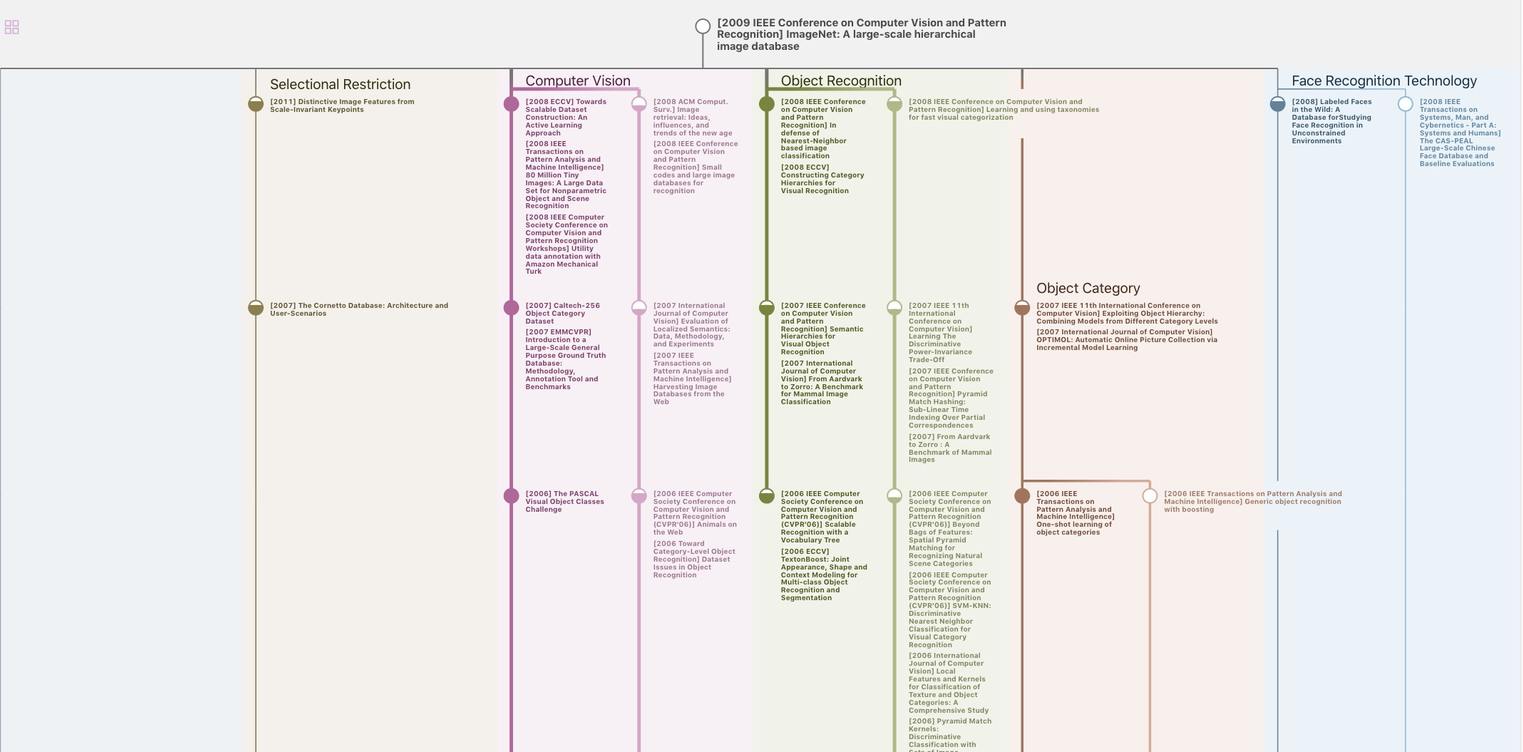
生成溯源树,研究论文发展脉络
Chat Paper
正在生成论文摘要