Improving Continual Learning by Accurate Gradient Reconstructions of the Past
ICLR 2023(2023)
摘要
Knowledge reuse is essential for continual learning, and current methods attempt to realize it through regularization or experience replay. These two strategies have complementary strengths, e.g., regularization methods are compact, but replay methods can mimic batch training more accurately. At present, little has been done to find principled ways to combine the two methods and current heuristics can give suboptimal performance. Here, we provide a principled approach to combine and improve them by using a recently proposed principle of adaptation, where the goal is to reconstruct the “gradients of the past”, i.e., to mimic batch training by estimating gradients from past data. Using this principle, we design a prior that provably gives better gradient reconstructions by utilizing two types of replay and a quadratic weight-regularizer. This improves performance on standard benchmarks such as Split CIFAR, Split TinyImageNet, and ImageNet-1000. Our work shows that a good combination of replay and regularizer-based methods can be very effective in reducing forgetting, and can sometimes even completely eliminate it.
更多查看译文
AI 理解论文
溯源树
样例
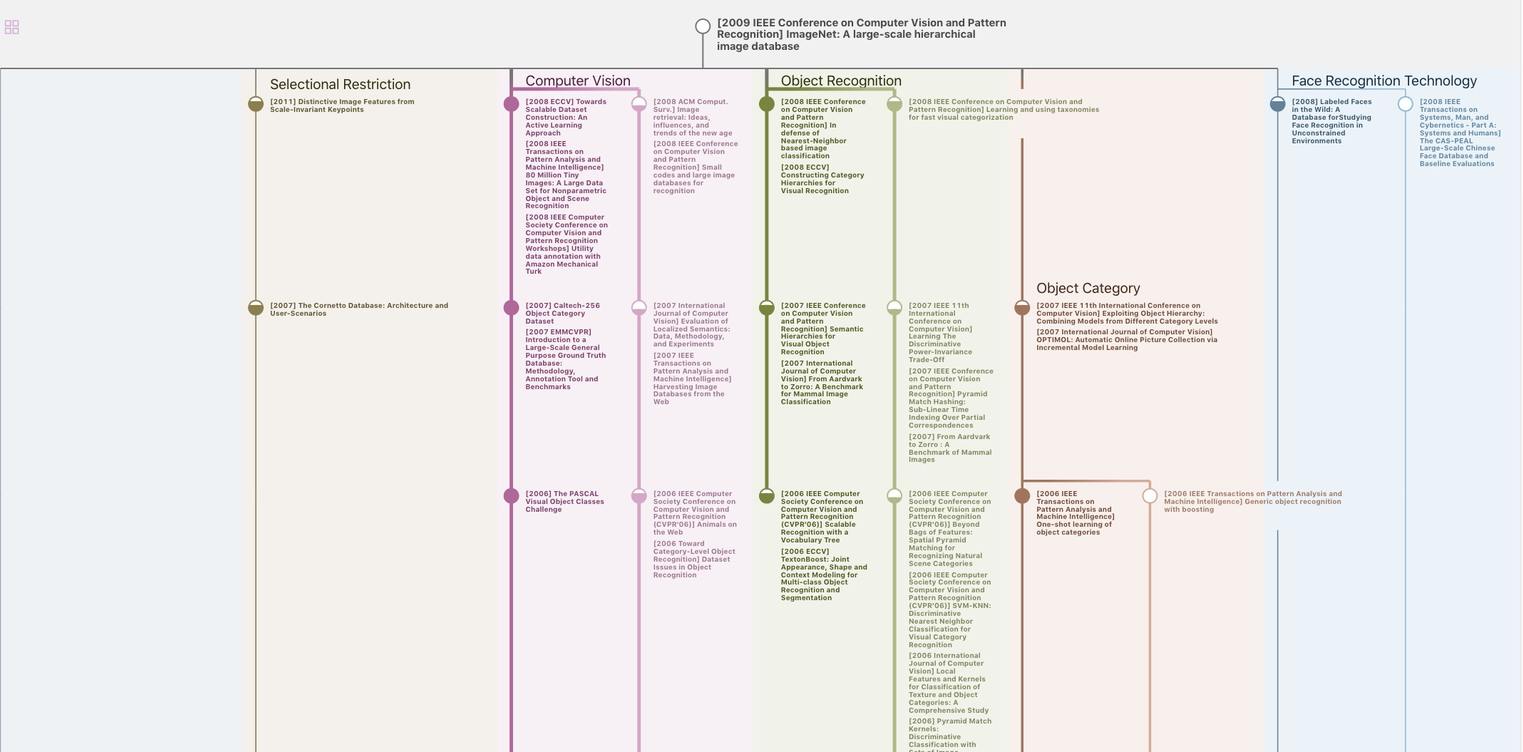
生成溯源树,研究论文发展脉络
Chat Paper
正在生成论文摘要