On the Expressive Power of Geometric Graph Neural Networks
ICLR 2023(2023)
摘要
The expressive power of Graph Neural Networks (GNNs) has been studied extensively through the lens of the Weisfeiler-Leman (WL) graph isomorphism test. Yet, many graphs arising in real-world applications come embedded in Euclidean space with an additional notion of geometric isomorphism, which is not covered by the WL framework. In this work, we propose a geometric version of the WL test (GWL) for discriminating geometric graphs while respecting the underlying physical symmetries: permutation, rotation, reflection, and translation. We use GWL to characterise the expressive power of GNNs that are invariant or equivariant to physical symmetries by studying the classes of geometric graphs that can or cannot be distinguished by these architectures. This allows us to formalise the advantages equivariant GNN layers have over their invariant counterparts in the Geometric Deep Learning blueprint. Finally, we connect our discrimination-based perspective with the universal approximation properties of geometric GNNs and prove they are two sides of the same coin.
更多查看译文
关键词
Graph Neural Networks,Geometric Deep Learning,Equivariance,Expressive Power,Graph Isomorphism
AI 理解论文
溯源树
样例
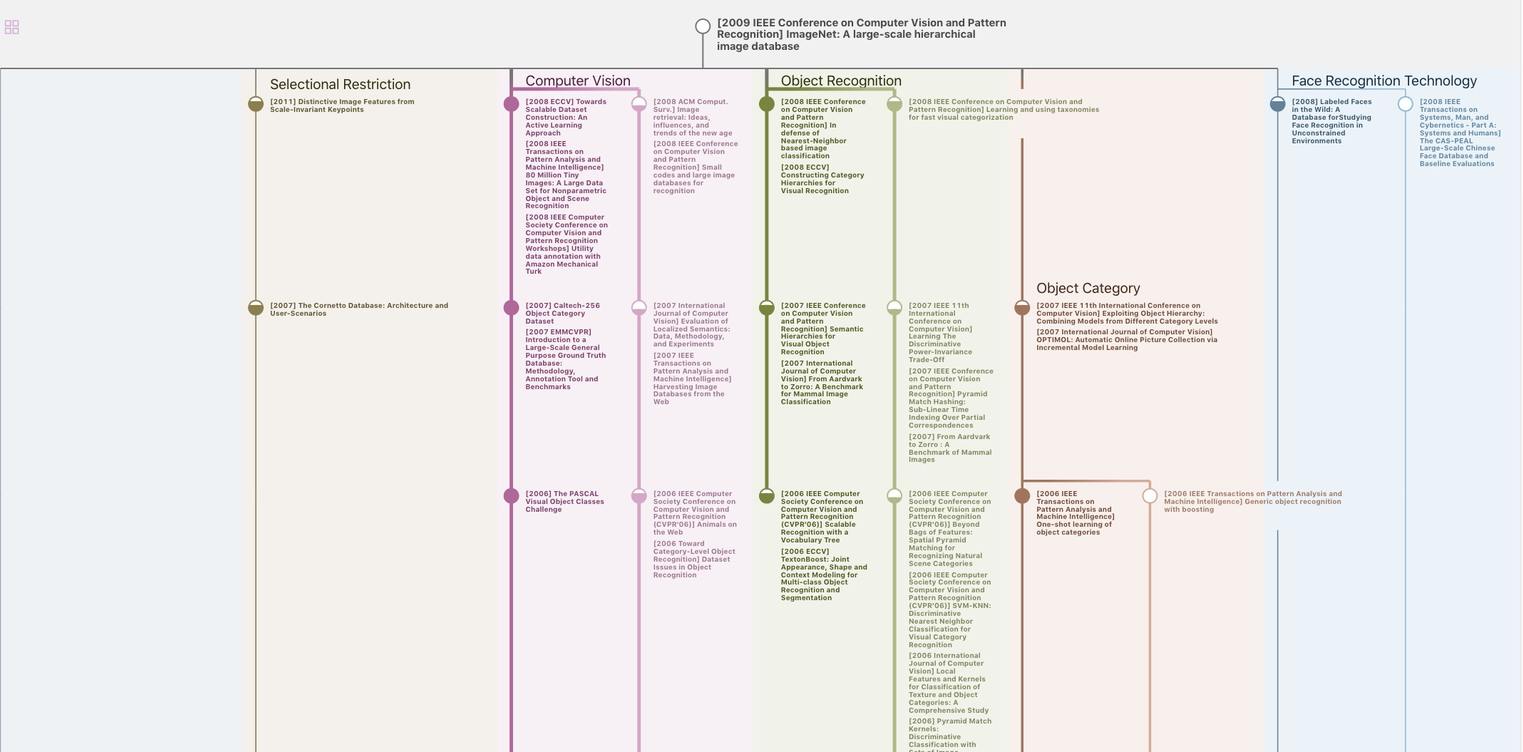
生成溯源树,研究论文发展脉络
Chat Paper
正在生成论文摘要