Meta-Learning via Classifier(-free) Guidance
ICLR 2023(2023)
摘要
State-of-the-art meta-learning techniques do not optimize for zero-shot adaptation to unseen tasks, a setting in which humans excel. On the contrary, meta-learning algorithms learn hyperparameters and weight initializations that explicitly optimize for few-shot learning performance. In this work, we take inspiration from recent advances in generative modeling and language-conditioned image synthesis to propose meta-learning techniques that use natural language guidance to achieve higher zero-shot performance compared to the state-of-the-art. We do so by recasting the meta-learning problem as a multi-modal generative modeling problem: given a task, we consider its adapted neural network weights and its natural language description as equivalent multi-modal task representations. We first train an unconditional generative hypernetwork model to produce neural network weights; then we train a second "guidance" model that, given a natural language task description, traverses the hypernetwork latent space to find high-performance task-adapted weights in a zero-shot manner. We explore two alternative approaches for latent space guidance: "HyperCLIP"-based classifier guidance and a conditional Hypernetwork Latent Diffusion Model ("HyperLDM"), which we show to benefit from the classifier-free guidance technique common in image generation. Finally, we demonstrate that our approaches outperform existing meta-learning methods with zero-shot learning experiments on our Meta-VQA dataset, which we specifically constructed to reflect the multi-modal meta-learning setting.
更多查看译文
关键词
deep leaning,meta learning,hypernetworks,generative models,classifier guidance,contrastive learning,clip,classifier-free guidance,latent diffusion,diffusion models
AI 理解论文
溯源树
样例
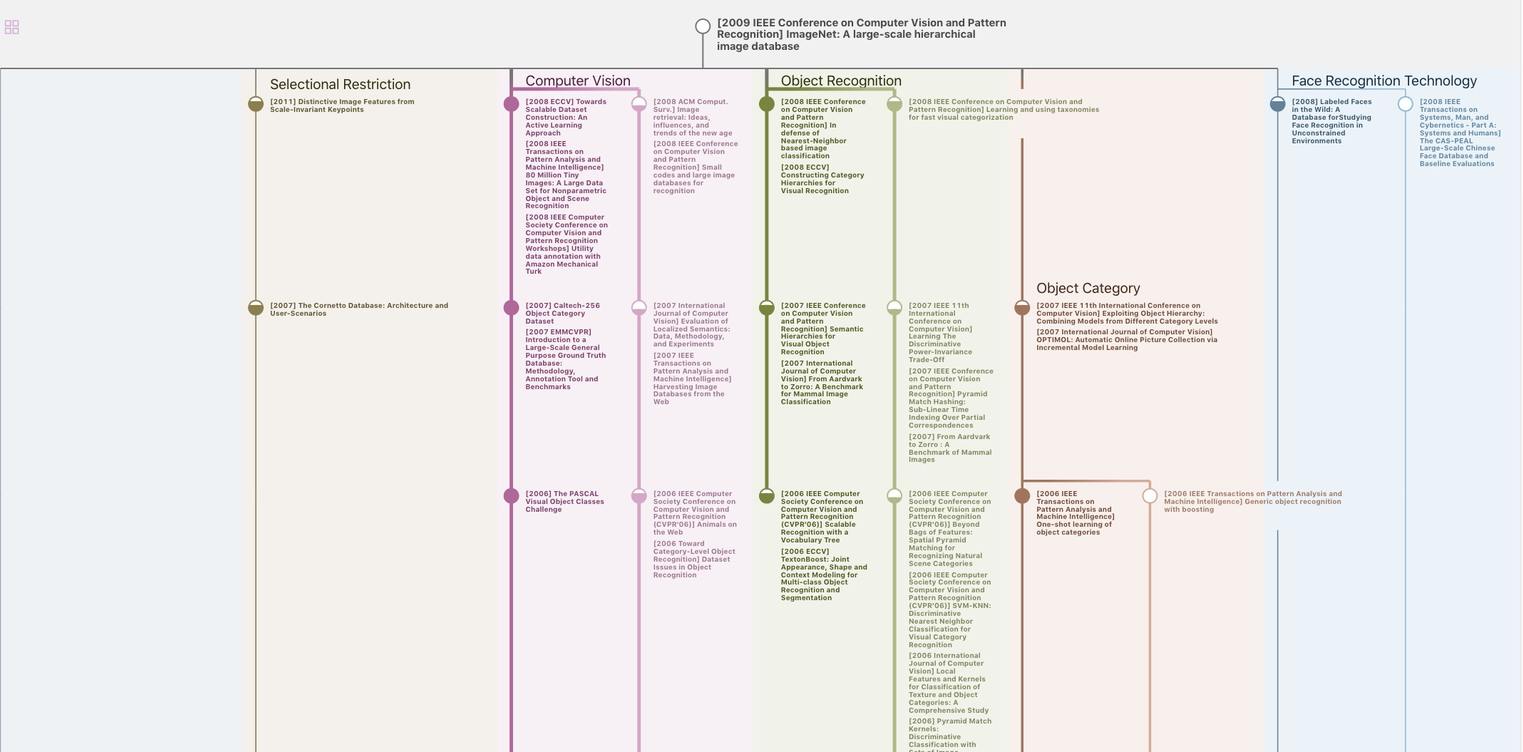
生成溯源树,研究论文发展脉络
Chat Paper
正在生成论文摘要