Applying Second Order Optimization to Deep Transformers with Parameter-Efficient Tuning
ICLR 2023(2023)
摘要
Despite the theoretical superiority in convergence issues, second-order optimizers are generally not among the top choices for training large-scale neural networks due to their high computational and memory cost. Nevertheless, introduced in recent progress of parameter-efficient tuning is a new paradigm that large-scale pre-trained models (PTMs) can be adapted to specific tasks by optimizing a tiny proportion of parameters, which might hopefully change the game. We associate this new paradigm with the computational tractability of second-order optimizers and succeed in applying them to large PTMs that are from hundreds of millions to billions in scale. Beyond verifying their tractability, we further investigate the stability-influencing factors in the optimization process and propose accordingly a Newton-step-clipping approach in which we clip the update tensors rather than the gradients. This approach stabilizes the convergence by gating the magnitude of Newton steps along the optimization trajectories through the rugged landscapes of deep transformers.
We conduct extensive experiments across different downstream tasks, demonstrating that, when equipped with our Newton-step-clipping strategy, second-order optimizers, especially Kronecker-factored curvature approximation (K-FAC), can attain comparable and even superior results and faster convergence to those state-of-the-art bars implemented with AdamW. Furthermore, we scale the model up to 3 billion parameters and validate the tractability and effectiveness of our method. This work is not only the first successful application of second-order optimization on such large-scale models but also sheds light on the possibility of further optimization-wise analysis on large-scale models in the future.
更多查看译文
关键词
Pre-trained Models,NLP,Model Adaptation
AI 理解论文
溯源树
样例
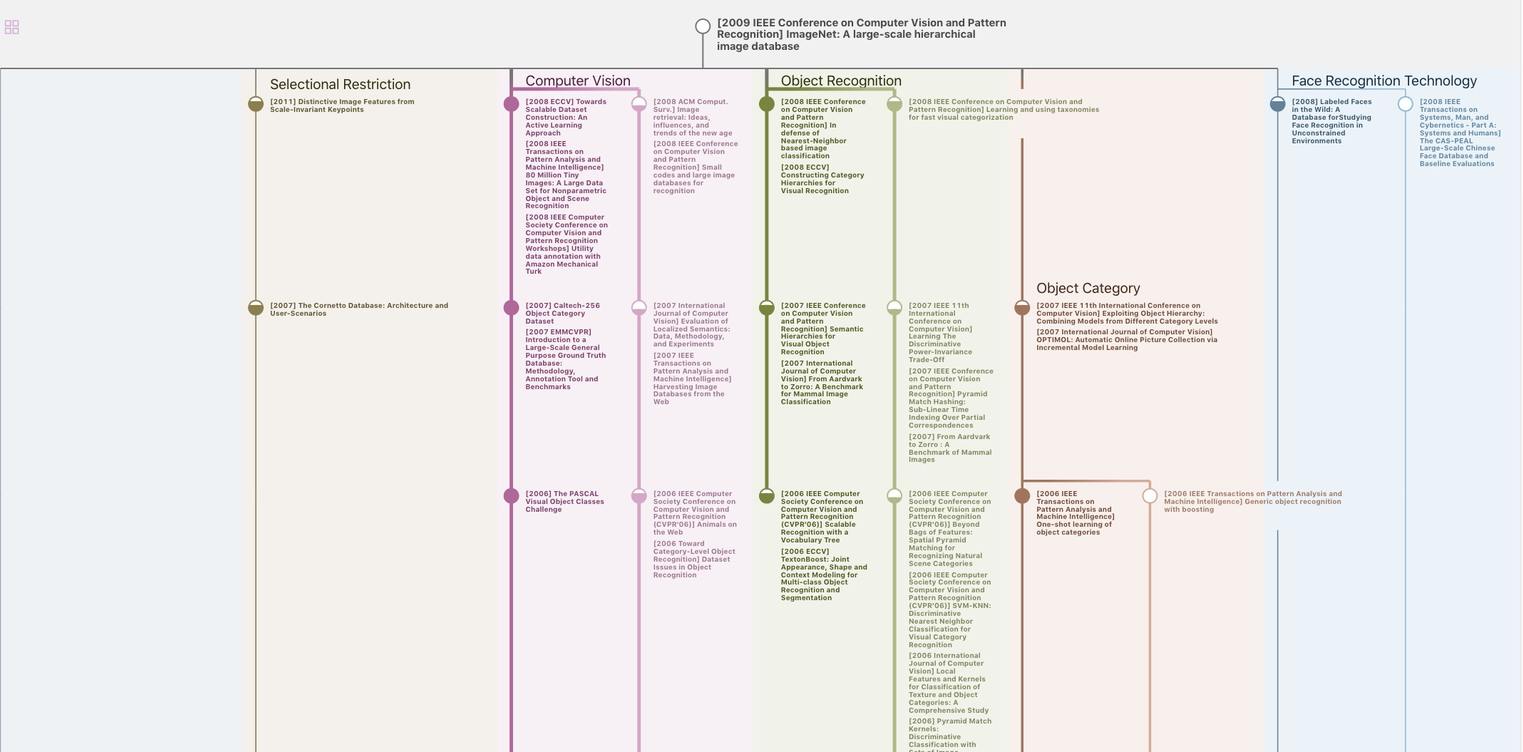
生成溯源树,研究论文发展脉络
Chat Paper
正在生成论文摘要