Discerning Hydroclimatic Behavior with a Deep Convolutional Residual Regressive Neural Network
ICLR 2023(2023)
摘要
Water impacts the globe daily in new and familiar ways such as the ongoing western United States drought and the 2022 Pakistan flood. These events sustain uncertainty, risk, and loss forces to the global ecosystem. Better forecasting tools are mandatory to calibrate our response in an effort to mitigate such natural hazards in our watersheds and adapt to the planet’s dynamic environment. Here, we present a Deep Convolutional Residual Regressive Neural Net (DCRRNN - pronounced “discern”) platform for obtaining, visualizing, and analyzing the basin response of watersheds to water cycle fluxes. We examine four very large basins, simulating river response to the hydroclimatic fluxes they face. Experiments modulating the lever of time lag between remotely sensed and ground truth measurements are performed to assess the metrological limits of this forecasting device. The resultant grand mean Nash Sutcliffe and Kling Gupta efficiency values are both of greater value than 90\%. Our results show that DCRRNN can become a powerful resource to simulate and forecast the impacts of hydroclimatic events as they relate to watershed response in a globally changing climate.
更多查看译文
关键词
water,climate,sustainability,supervised representation learning,societal considerations
AI 理解论文
溯源树
样例
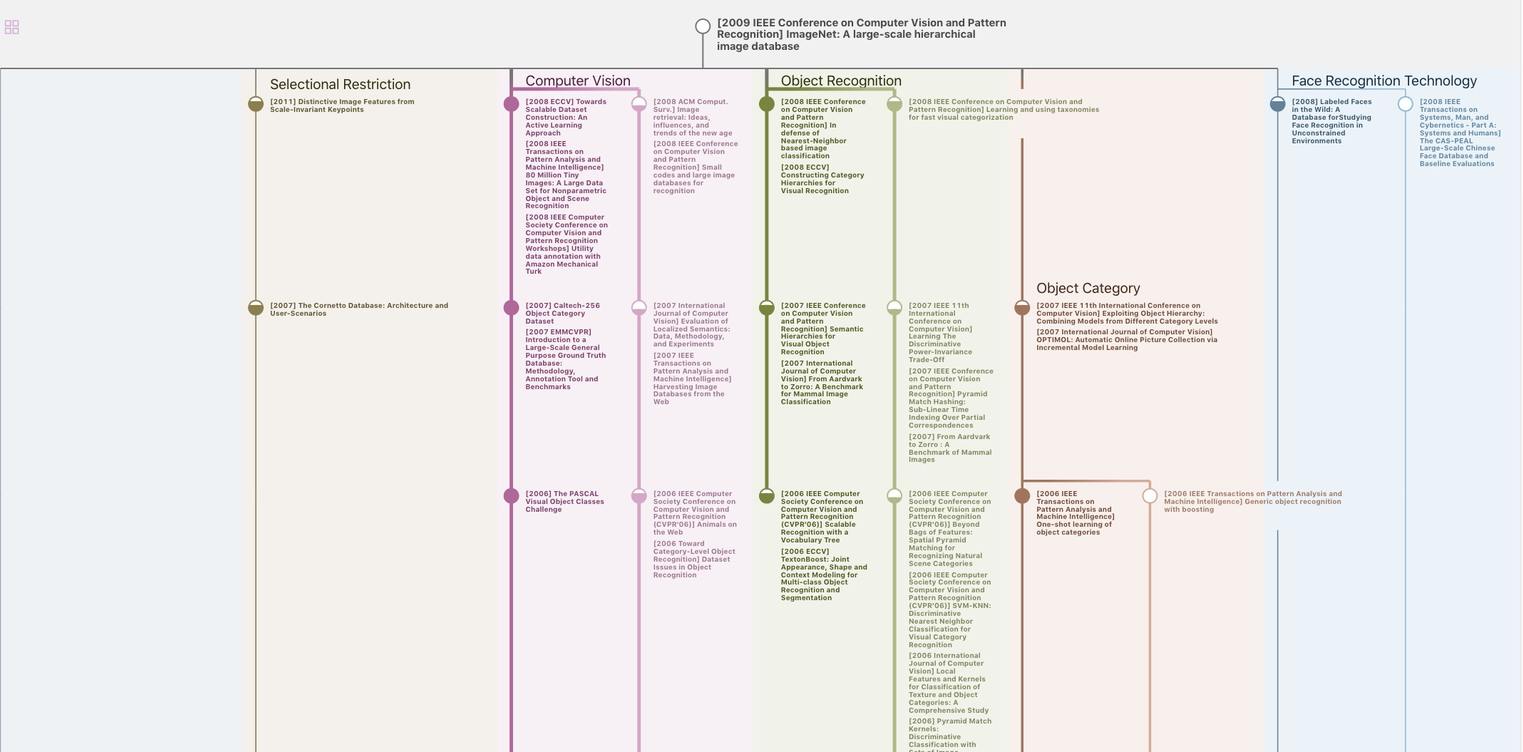
生成溯源树,研究论文发展脉络
Chat Paper
正在生成论文摘要