When Rigid Coherency Hurts: Distributional Coherency Regularization for Probabilistic Hierarchical Time Series Forecasting
ICLR 2023(2023)
摘要
Probabilistic hierarchical time-series forecasting is an important variant of time-series forecasting, where the goal is to model and forecast multivariate time-series that have hierarchical relations. Previous works all assume rigid consistency over the given hierarchies and do not adapt to real-world data that show deviation from this assumption. Moreover, recent state-of-art neural probabilistic methods also impose hierarchical relations on point predictions and samples of distribution. This does not account for full forecast distributions being coherent with the hierarchy and leads to poorly calibrated forecasts. We close both these gaps and propose PROFHIT, a probabilistic hierarchical forecasting model that jointly models forecast distributions over the entire hierarchy. PROFHIT (1) uses a flexible probabilistic Bayesian approach and (2) introduces soft distributional coherency regularization that enables end-to-end learning of the entire forecast distribution leveraging information from the underlying hierarchy. This enables robust and calibrated forecasts as well as adaptation to real-life data with varied hierarchical consistency. PROFHIT provides 41-88% better performance in accuracy and 23-33% better calibration over a wide range of dataset consistency. Furthermore, PROFHIT can robustly provide reliable forecasts even if up to 10% of input time-series data is missing, whereas other methods’ performance severely degrade by over 70%.
更多查看译文
关键词
Hierarchical Forecasting,Time Series Forecasting,Deep Probabilistic Models
AI 理解论文
溯源树
样例
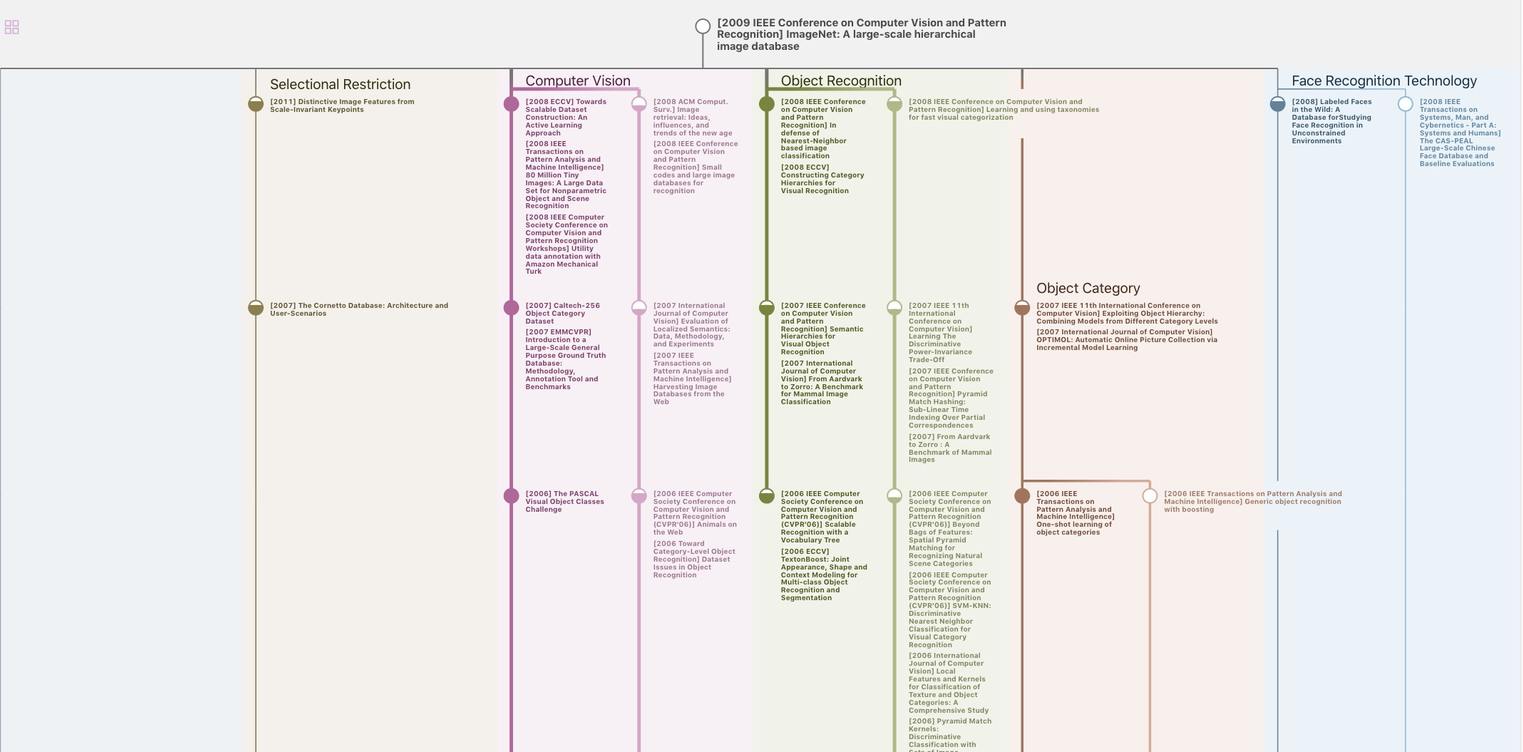
生成溯源树,研究论文发展脉络
Chat Paper
正在生成论文摘要