Robust Federated Learning with Majority Adversaries via Projection-based Re-weighting
ICLR 2023(2023)
摘要
Most robust aggregators for distributed or federated learning assume that adversarial clients are the minority in the system. In contrast, this paper considers the majority adversary setting. We first show that a filtering method using a few trusted clients can defend against many standard attacks. However, a new attack called Mimic-Shift can circumvent simple filtering. To this end, we develop a re-weighting strategy that identifies and down-weights the potential adversaries under the majority adversary regime. We show that our aggregator converges to a neighborhood around the optimum under the Mimic-Shift attack. Empirical results further show that our aggregator achieves negligible accuracy loss with a majority of adversarial clients, outperforming strong baselines.
更多查看译文
关键词
Federated learning,robustness,adversarial attack,majority adversary
AI 理解论文
溯源树
样例
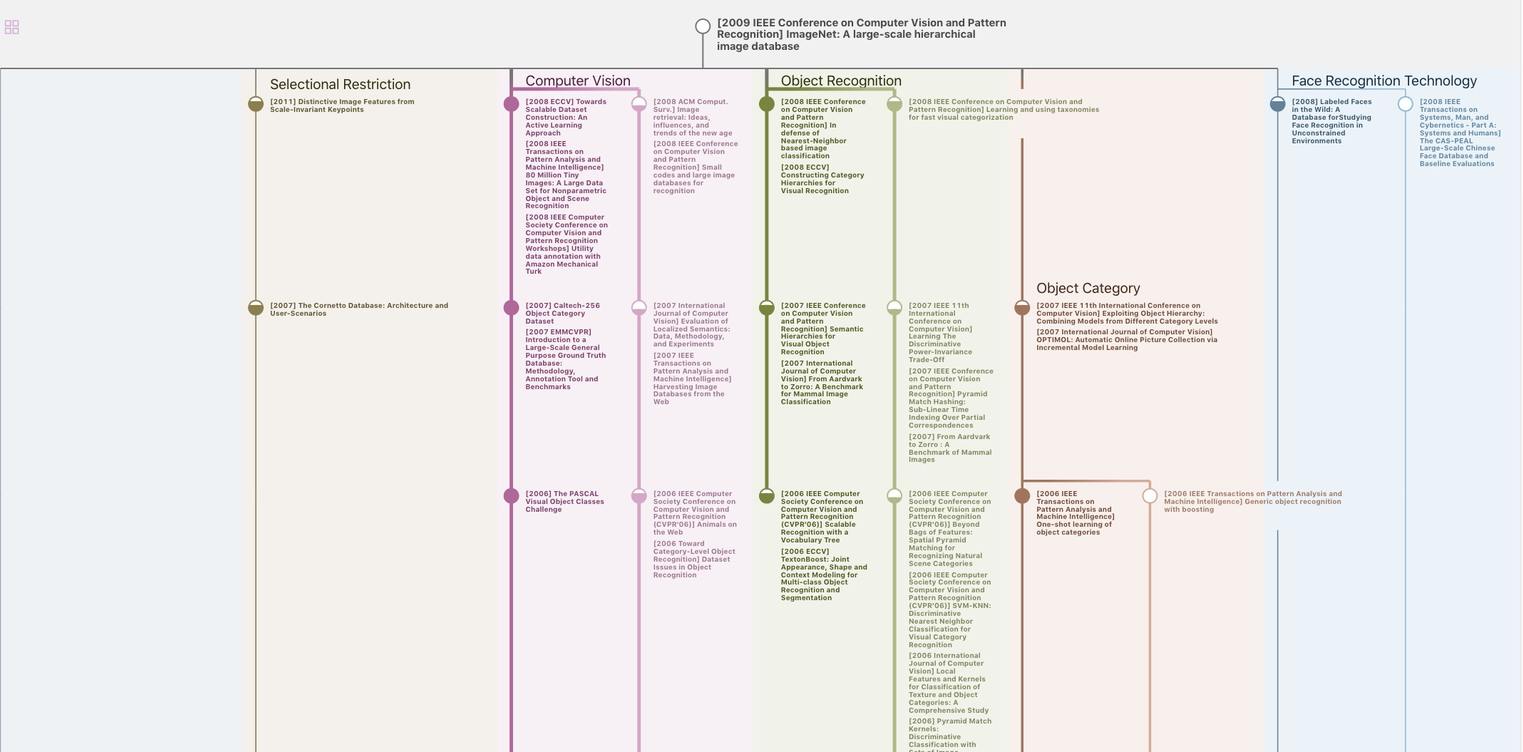
生成溯源树,研究论文发展脉络
Chat Paper
正在生成论文摘要