DIGEST: FAST AND COMMUNICATION EFFICIENT DECENTRALIZED LEARNING WITH LOCAL UPDATES
ICLR 2023(2023)
摘要
Decentralized learning advocates the elimination of centralized parameter servers
(aggregation points) for potentially better utilization of underlying resources, de-
lay reduction, and resiliency against parameter server unavailability and catas-
trophic failures. Gossip based decentralized algorithms, where each node in a net-
work has its own locally kept model on which it effectuates the learning by talking
to its neighbors, received a lot of attention recently. Despite their potential, Gossip
algorithms introduce huge communication costs. In this work, we show that nodes
do not need to communicate as frequently as in Gossip for fast convergence; in
fact, a sporadic exchange of a digest of a trained model is sufficient. Thus, we
design a fast and communication-efficient decentralized learning mechanism; DI-
GEST by particularly focusing on stochastic gradient descent (SGD). DIGEST is
a decentralized algorithm building on local-SGD algorithms, which are originally
designed for communication efficient centralized learning. We show through anal-
ysis and experiments that DIGEST significantly reduces the communication cost
without hurting convergence time for both iid and non-iid data.
更多查看译文
关键词
Decentralized Learning,Distributed Optimization,Communication Efficient Learning,Local SGD,Federated Learning
AI 理解论文
溯源树
样例
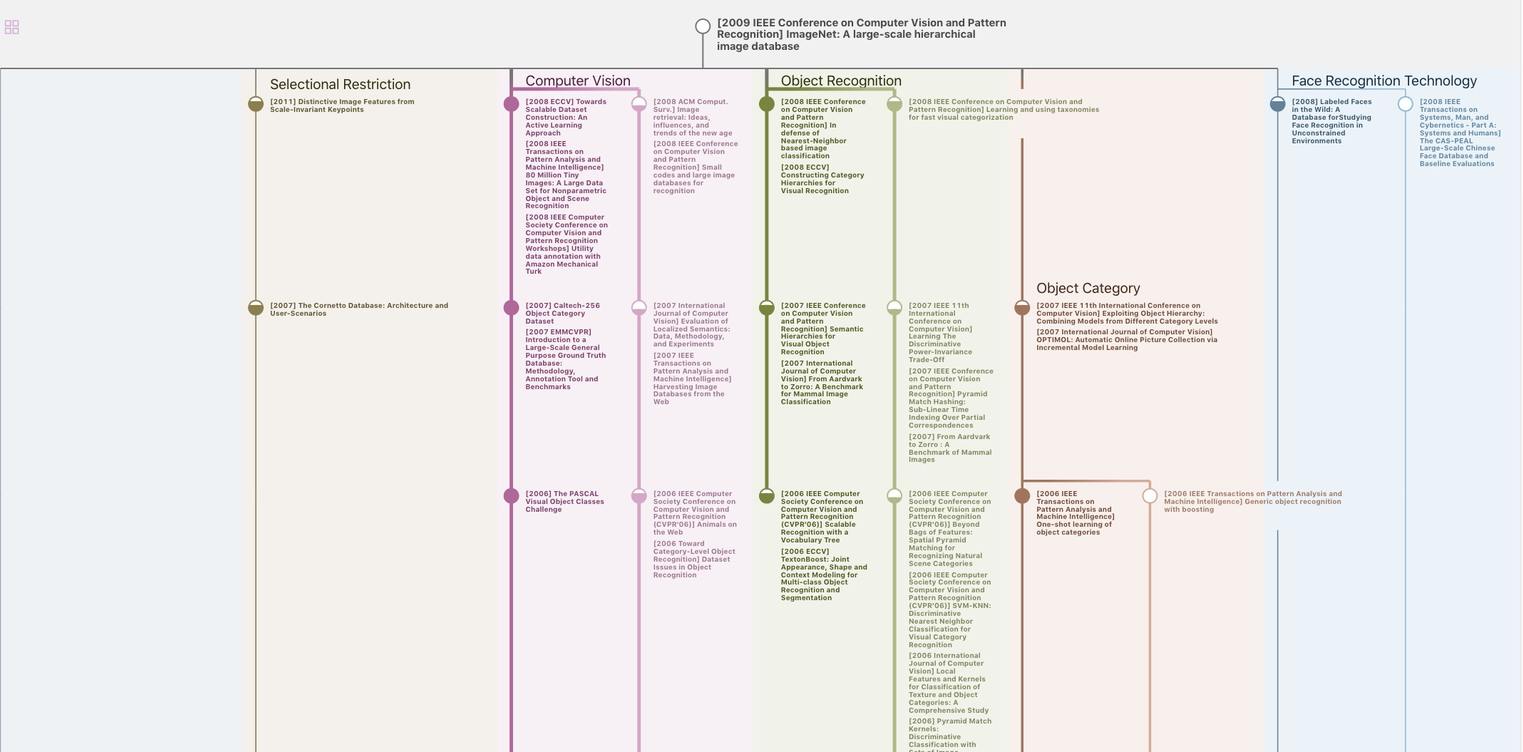
生成溯源树,研究论文发展脉络
Chat Paper
正在生成论文摘要