Matrix factorization under the constraint of connectivity between observed and source data ~ Muscle synergy analysis based on connectivity between muscle and brain activities ~
ICLR 2023(2023)
Abstract
Matrix factorization is a popular method to investigate the hidden elements in observed data for tasks such as speech separation and muscle synergy analysis. The hidden elements may be closely related to the source phenomenon that cause the observed phenomenon.
However, conventional methods do not always factorize the observed phenomenon elements with the connectivity between the observed and source phenomena because they only use the observed phenomenon. This paper proposes a matrix decomposition method that constrains the connectivity between observed and source data by using the representations from a decoding model from source data to observed data. We applied our method to the corticomuscular system, which is made up of corticospinal pathways between the primary motor cortex and muscles in the body and creates muscle synergies that enable efficient connections between the brain and muscles. In this context, muscle activities are the observed phenomenon and brain activities are the source. Many previous studies have analyzed muscle synergies using only observed muscle activity, but there may be unrevealed muscle synergies under the constraint of the connectivity between brain and muscle activity. We therefore simultaneously recorded the brain activity from multiple regions of an extensive cortical area and the activity of multiple muscles of a monkey's forelimb while it performed a reach and grasp task throughout the course of recovery from a partial spinal cord injury (SCI). Analysis from a dataset of the monkey before SCI showed that some of the muscle synergies calculated from the proposed method using brain and muscle activities, did not exhibit a high degree of similarity to synergies obtained from the conventional method. The proposed method results obtained from the monkey after SCI showed an adaptive change in the number of muscle synergies associated with the degree of functional recovery. Specifically, the numbers of muscle synergies obtained by the proposed method initially increased immediately after SCI and then gradually decreased, while those obtained by a conventional method maintained the same number before and after SCI. These results suggest that our method is able to capture the unrevealed connectivity in the corticomuscular system that contributes to functional recovery: in other words, that it can factorize the observed data under the constraint of the connectivity between the observed and source data. Our work thus demonstrates the importance of using not only observed data but also source data to reveal unknown hidden elements.
MoreTranslated text
Key words
Matrix factorization,Muscle synergy
AI Read Science
Must-Reading Tree
Example
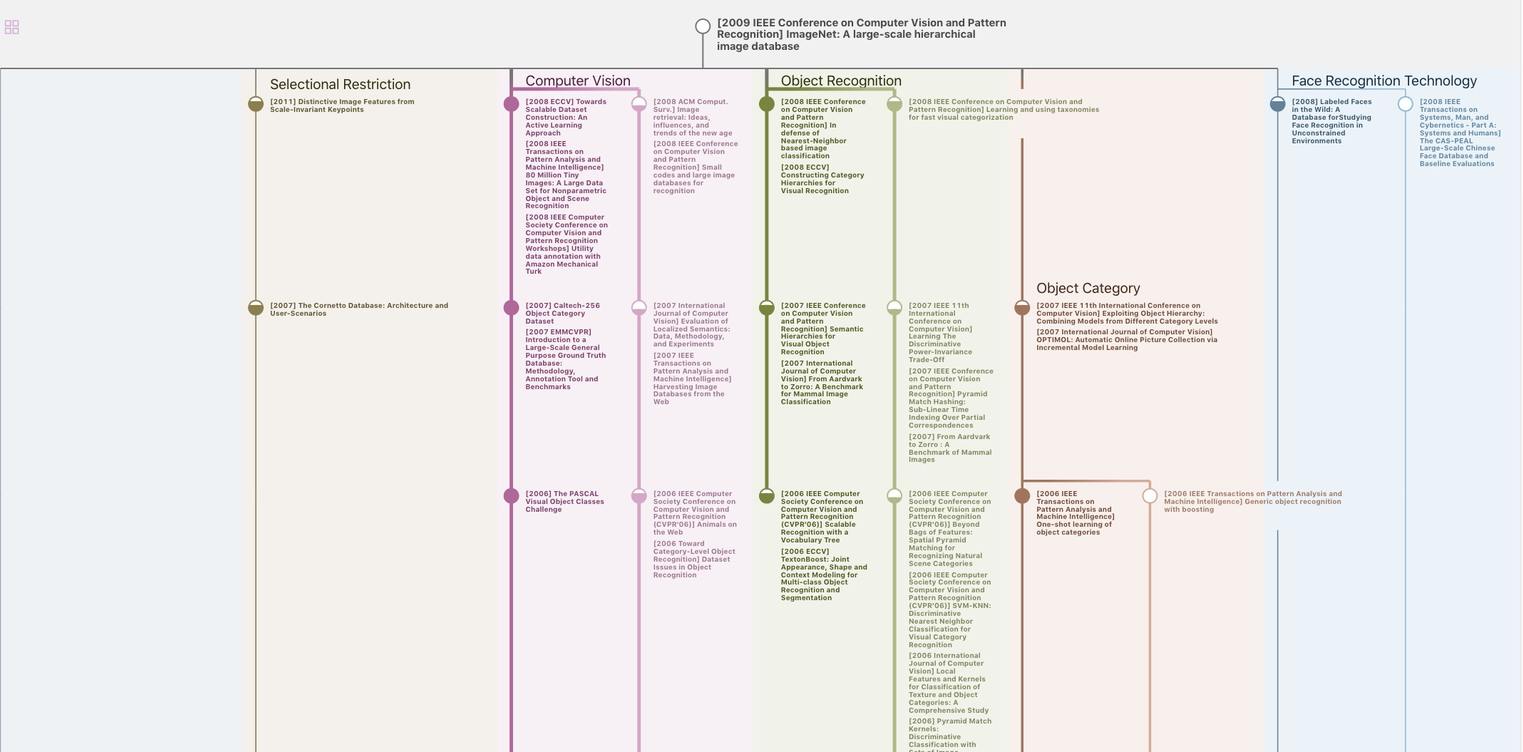
Generate MRT to find the research sequence of this paper
Chat Paper
Summary is being generated by the instructions you defined