On The Impact of Machine Learning Randomness on Group Fairness
ICLR 2023(2023)
摘要
Statistical measures for group fairness in machine learning reflect the gap in performance of algorithms across different groups. These measures, however, exhibit a high variance, between different training instances, that makes them unreliable for empirical evaluation of fairness. What is the cause of this variance, and how can we reduce it? We investigate the impact of different sources of randomness in machine learning on group fairness. We show that the variance in group fairness measures is mainly due to the high volatility of the learning process on under-represented groups, which itself is largely caused by the stochasticity of data order during training. Based on these findings, we show how to manipulate group level accuracy (i.e. model fairness), with high efficiency and negligible impact on the overall predictive power of the model, by changing the data order.
更多查看译文
关键词
neural networks, fairness, randomness in training, evaluation
AI 理解论文
溯源树
样例
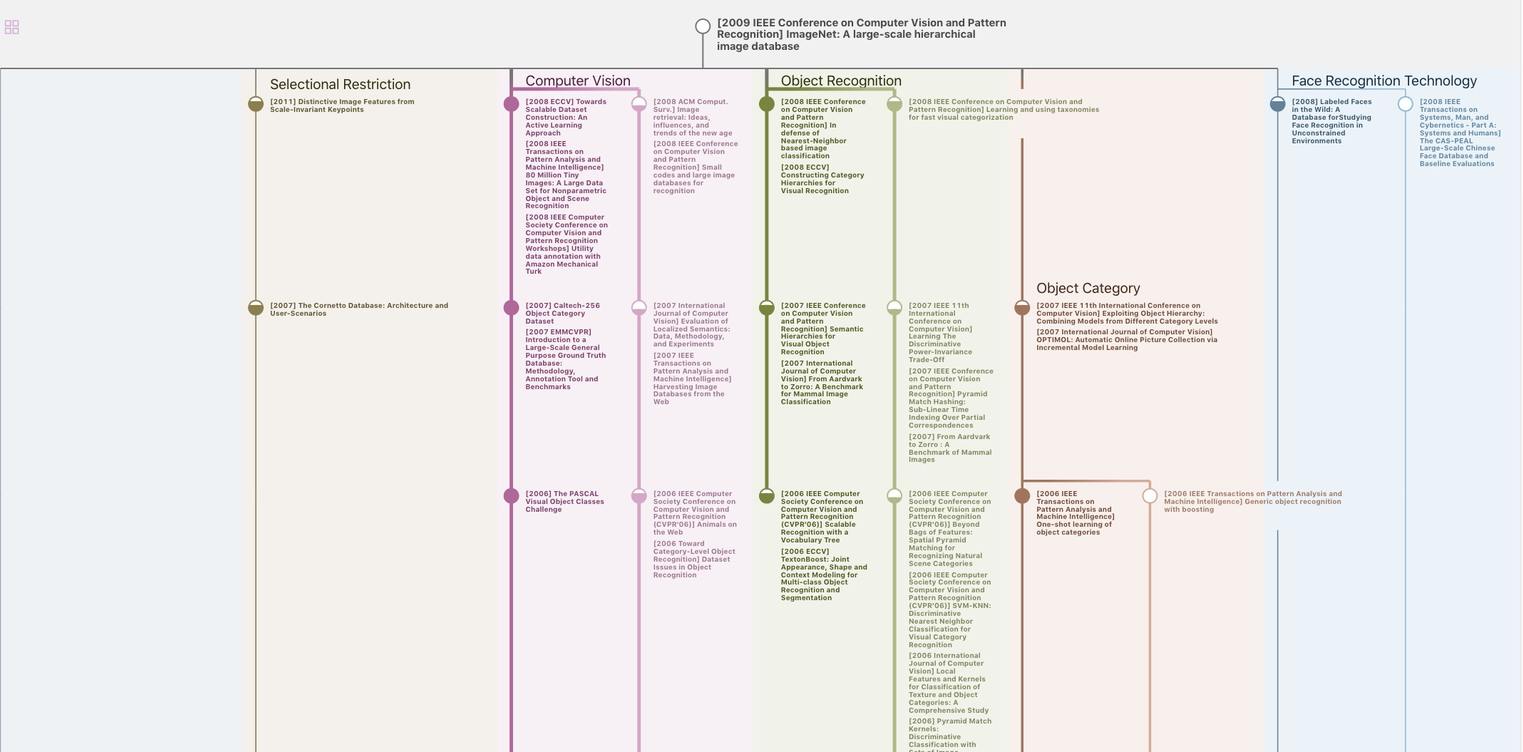
生成溯源树,研究论文发展脉络
Chat Paper
正在生成论文摘要