Continual Learning Based on Sub-Networks and Task Similarity
ICLR 2023(2023)
摘要
Continual learning (CL) has two main objectives: preventing catastrophic forgetting (CF) and encouraging knowledge transfer (KT) across tasks. The existing literature mainly tries to overcome CF. Although some papers have focused on both CF and KT, they may still suffer from CF because of their ineffective handling of previous tasks and/or poor task similarity detection mechanisms to achieve KT. This work presents a new CL method that addresses the above issues. First, it overcomes CF by isolating the knowledge of each task via a learned mask that indicates a sub-network. Second, it proposes a novel technique to compute how important each mask is to the new task, which indicates how the new task is similar to an underlying old task. Similar tasks can share the same mask/subnetwork for KT, while dissimilar tasks use different masks/sub-networks for CF prevention. Comprehensive experiments have been conducted using a range of NLP problems, including classification, generation, and extraction to show that the proposed method consistently outperforms prior state-of-the-art baselines.
更多查看译文
关键词
Continual learning,NLP tasks,Task Similarity,Sub-network
AI 理解论文
溯源树
样例
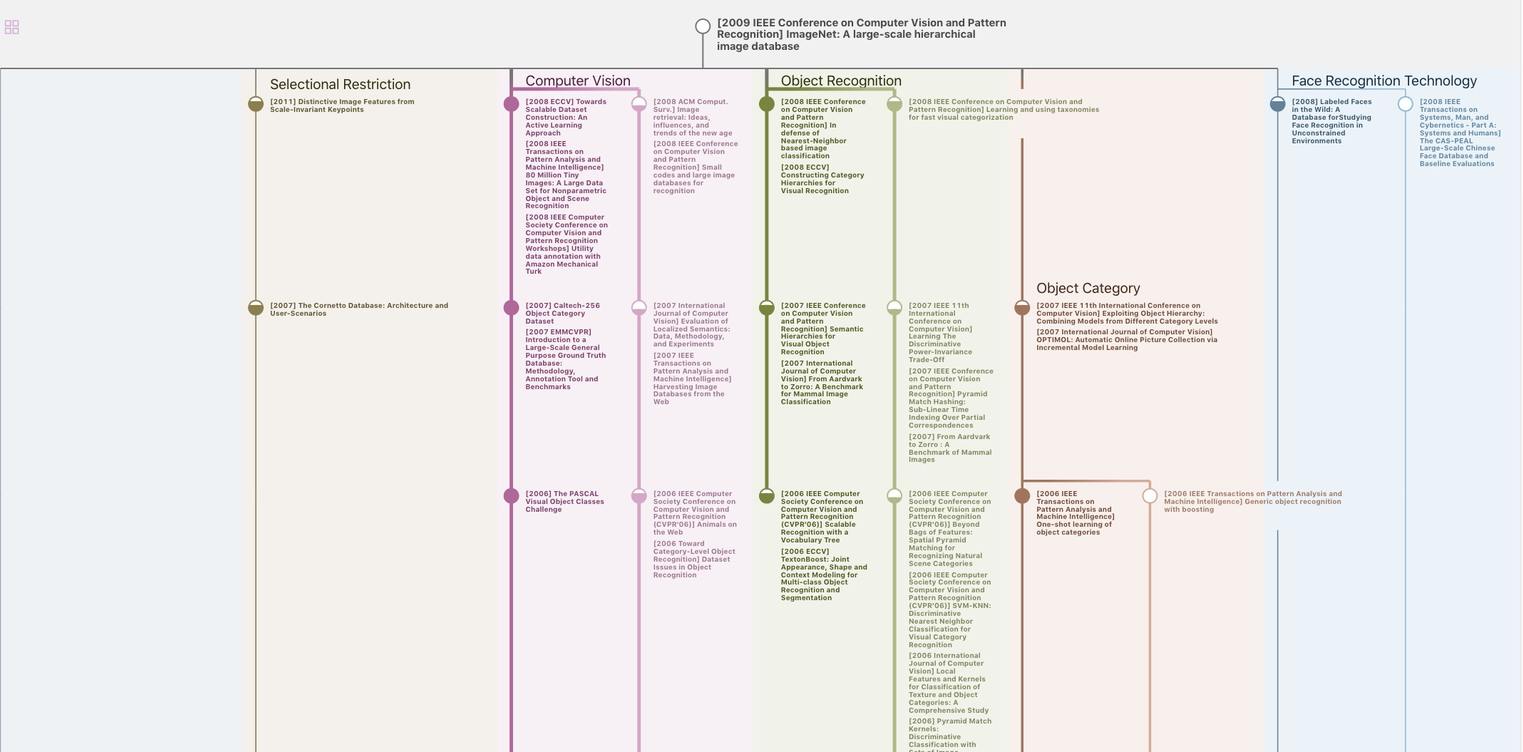
生成溯源树,研究论文发展脉络
Chat Paper
正在生成论文摘要