A Picture of the Space of Typical Learning Tasks
ICLR 2023(2023)
摘要
We develop a technique to analyze representations learned by deep networks when they are trained on different tasks using supervised, multi-task, meta- and contrastive learning. We develop a technique to visualize such representations using an isometric embedding of the space of probabilistic models into a lower-dimensional space, i.e., one that preserves pairwise distances. We discover the following surprising phenomena that shed light upon the structure in the space of learning tasks: (1) the manifold of probabilistic models trained on different tasks using different representation learning methods is effectively low-dimensional; (2) supervised learning on one task results in a surprising amount of progress on seemingly dissimilar tasks; progress on other tasks is larger if the training task has diverse classes; (3) the structure of the space of tasks indicated by our visualization technique is consistent with parts of the Wordnet phylogenetic tree; (4) fine-tuning a model upon a sub-task does not change the representation much if the model was trained for a large number of epochs; (5) episodic meta-learning algorithms fit similar models eventually as that of supervised learning, even if the two traverse different trajectories during training; (6) contrastive learning methods trained on different datasets learn similar representations. We use classification tasks constructed from the CIFAR-10 and Imagenet datasets to study these phenomena.
更多查看译文
关键词
Information Geometry,Space of learning tasks
AI 理解论文
溯源树
样例
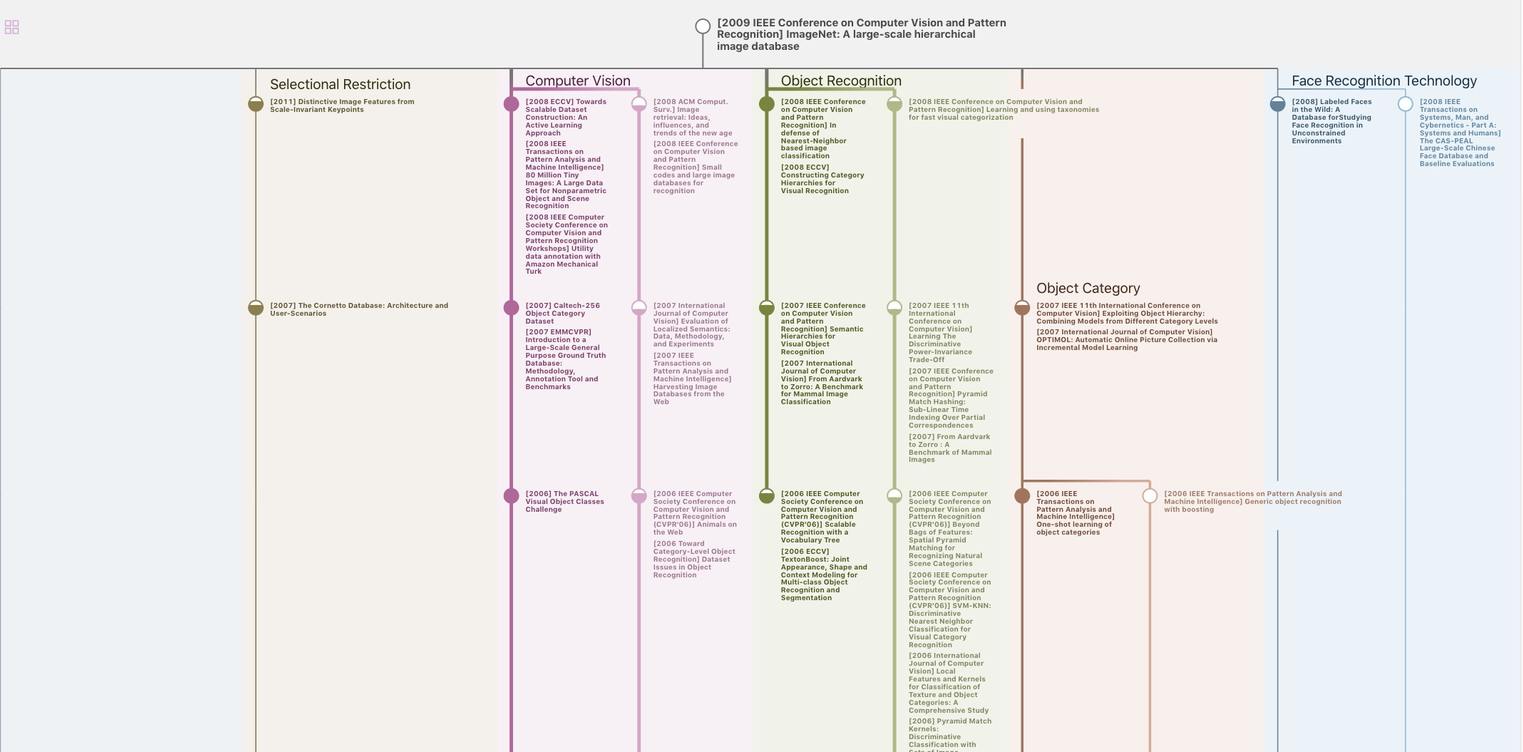
生成溯源树,研究论文发展脉络
Chat Paper
正在生成论文摘要