WebBrain: Learning to Generate Factually Correct Articles for Queries by Grounding on Large Web Corpus
ICLR 2023(2023)
摘要
In this paper, we introduce a new NLP task – generating short factual articles for queries by mining supporting evidence from the Web. In this task, called WebBrain, the ultimate goal is to generate a fluent, informative, and factually-correct short article (e.g., Wiki article) for a factual query unseen in Wikipedia. To enable experiments on WebBrain, we construct a large-scale dataset WebBrain-Raw by extracting English Wikipedia articles and their crawlable Wiki references. WebBrain-Raw is ten times larger than the previous biggest peer dataset, which can greatly benefit the research community. Besides, we empirically analyze the performances of the current state-of-the-art NLP techniques on WebBrain and introduce a new framework ReGen, which enhances the generation factualness by improved evidence retrieval and task-specific pre-training for generation. Experiment results show that ReGen outperforms all baselines in both automatic and human evaluations.
更多查看译文
关键词
factual generation,retrieval-augmented generation,new large-scale dataset
AI 理解论文
溯源树
样例
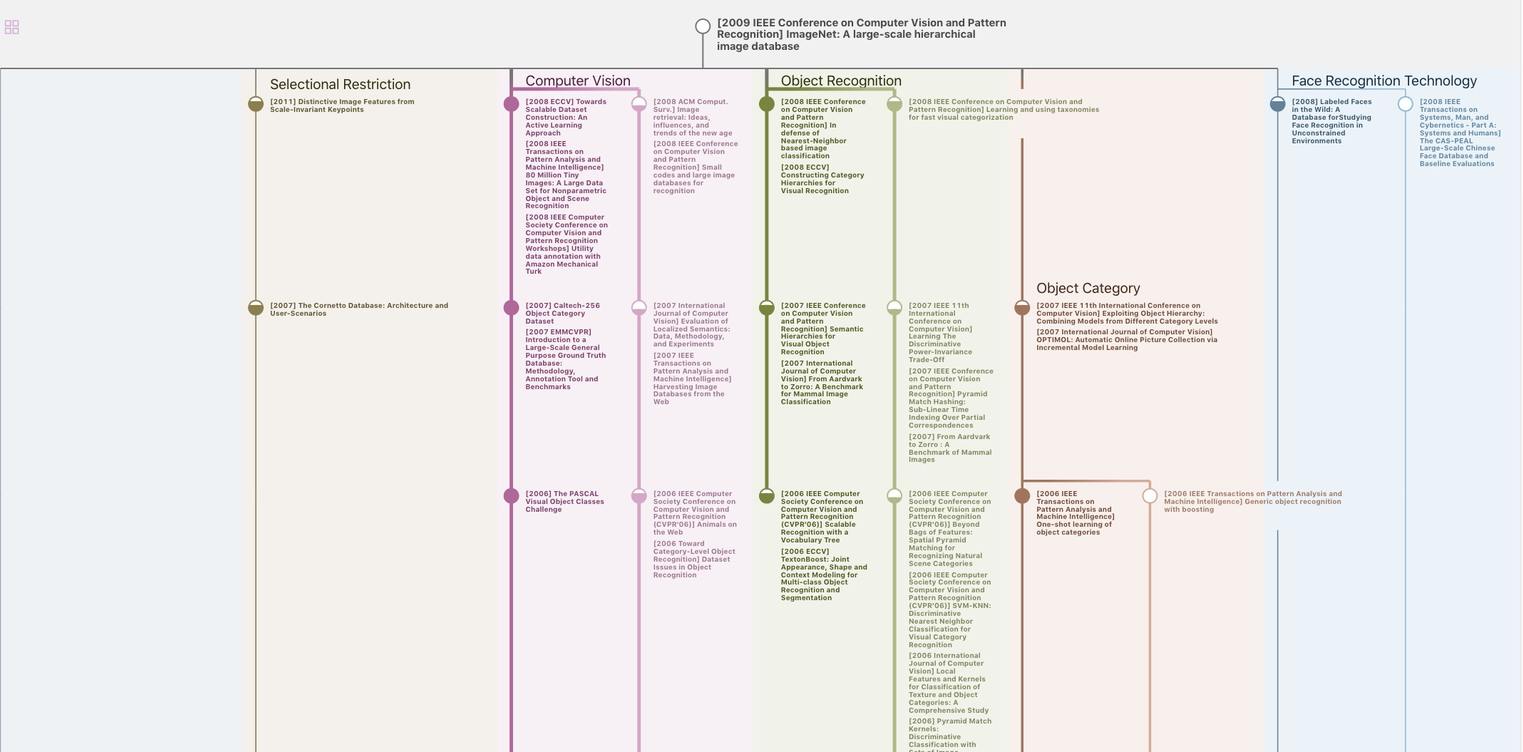
生成溯源树,研究论文发展脉络
Chat Paper
正在生成论文摘要