Distributed Least Square Ranking with Random Features
ICLR 2023(2023)
摘要
In this paper, we study the statistical properties of pairwise ranking using distributed learning and random features (called DRank-RF) and establish its convergence analysis in probability. Theoretical analysis shows that DRank-RF remarkably reduces the computational requirements while preserving a satisfactory convergence rate. An extensive experiment verifies the effectiveness of DRank-RF. Furthermore, to improve the learning performance of DRank-RF, we propose an effective communication strategy for it and demonstrate the power of communications via theoretical assessments and numerical experiments.
更多查看译文
关键词
least square ranking,distributed learning,learning theory,random features
AI 理解论文
溯源树
样例
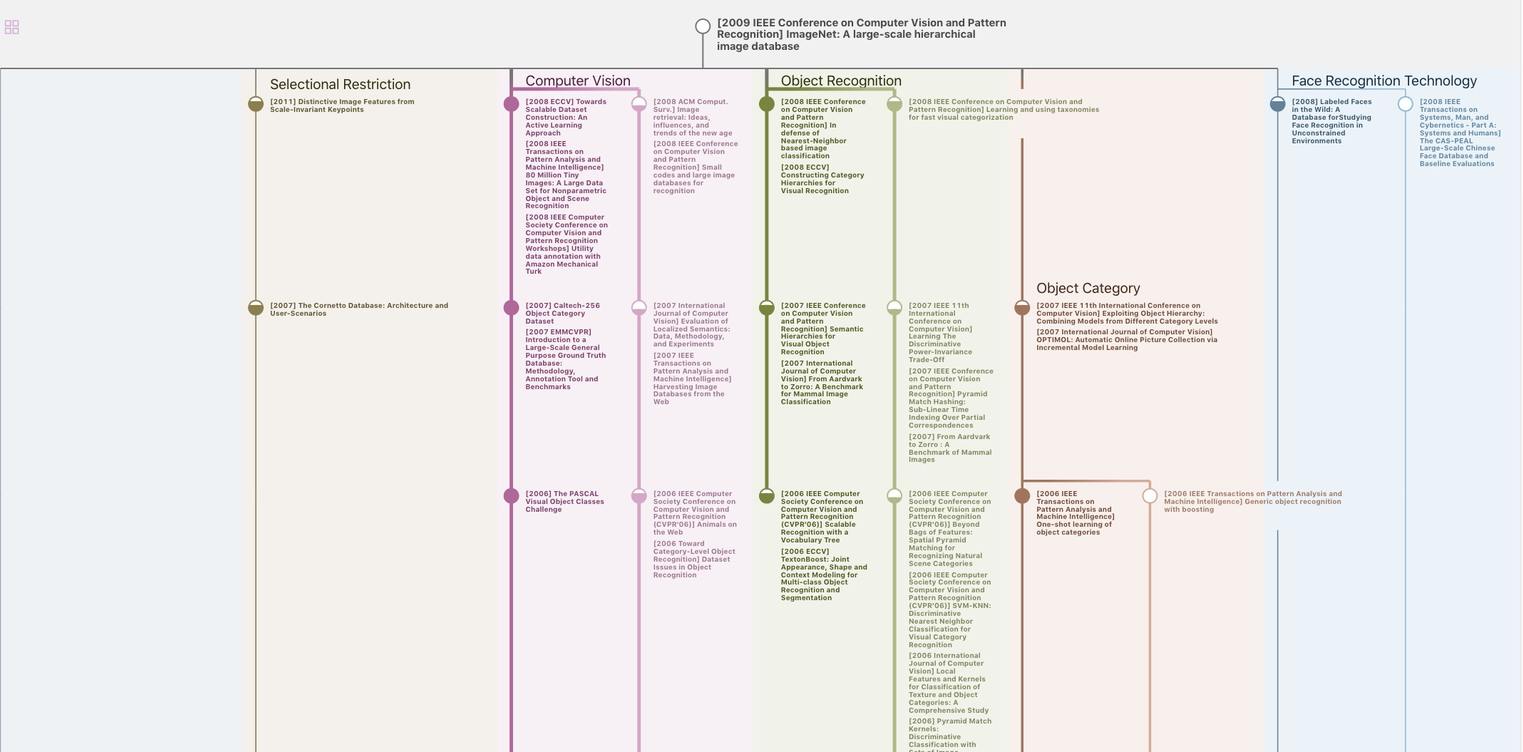
生成溯源树,研究论文发展脉络
Chat Paper
正在生成论文摘要