Fine-grained Lesion Classification Framework for Early Auxiliary Diagnosis.
IEEE/ACM transactions on computational biology and bioinformatics(2023)
摘要
The deep neural networks are envisaged for the early disease diagnosis from medical images. However, in the early stage of the disease, the medical images of patients and healthy people have only subtle visual differences. Distinguishing the medical images for early diagnosis belongs to the Fine-Grained Visual Classification (FGVC) task. Many recent works are based on a standard FGVC learning paradigm: locate the discriminative regions first and then classify by fusing the information of these regions. However, it is still not enough for medical images. Because the shape and size of the lesions are variable, and the relationship between lesions and the background is complex. In order to solve these problems, we propose a fine-grained lesion classification framework for early auxiliary diagnosis. We first locate and extract multiple lesions with different sizes and shapes from the original image and then fuse the feature of lesion and background based on attention mechanism. As shown by experiment results in two real-world clinical data sets, our model can locate accurately and perform better.
更多查看译文
关键词
Fine-grained visual classification task,Attention mechanism
AI 理解论文
溯源树
样例
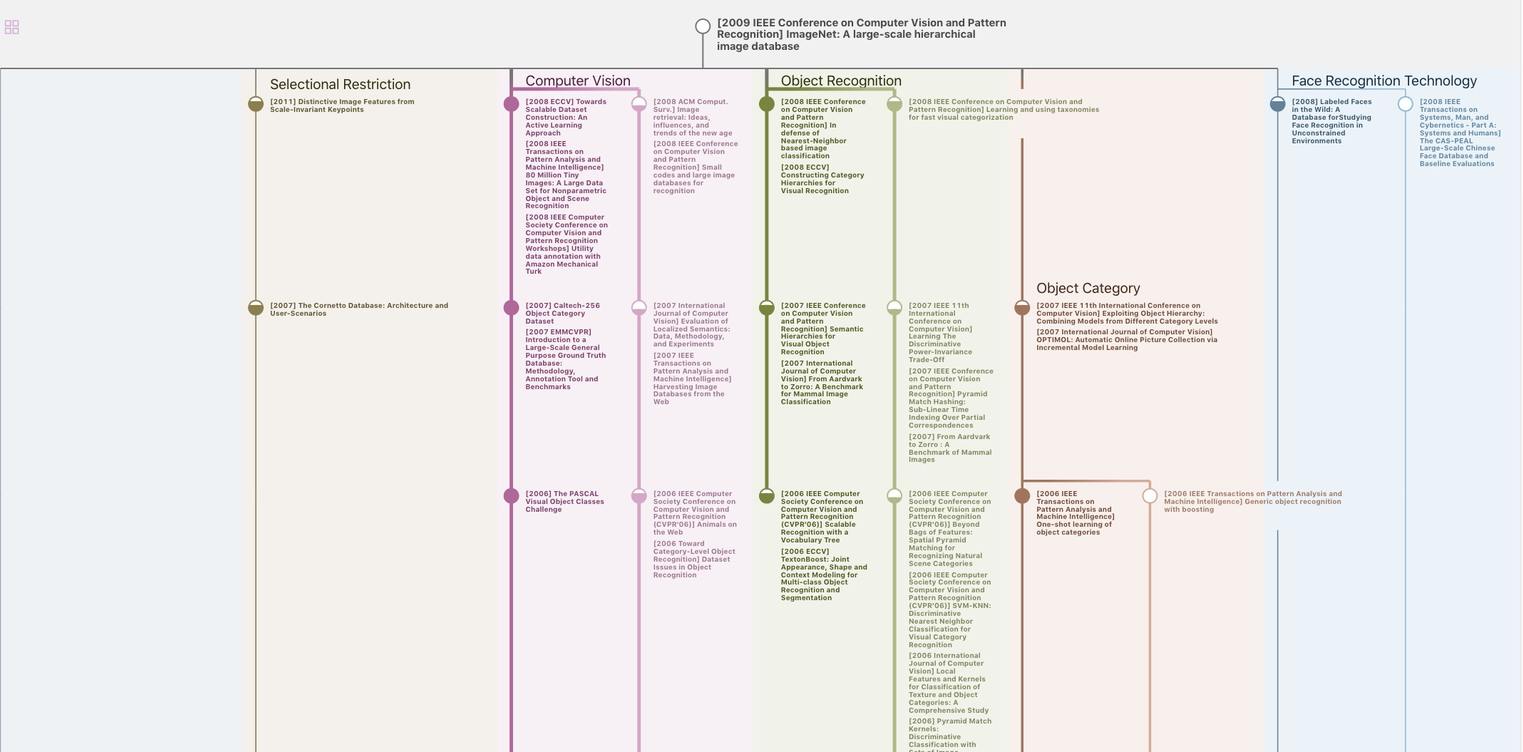
生成溯源树,研究论文发展脉络
Chat Paper
正在生成论文摘要