Class-Incremental Learning Method With Fast Update and High Retainability Based on Broad Learning System.
IEEE transactions on neural networks and learning systems(2023)
摘要
Machine learning aims to generate a predictive model from a training dataset of a fixed number of known classes. However, many real-world applications (such as health monitoring and elderly care) are data streams in which new data arrive continually in a short time. Such new data may even belong to previously unknown classes. Hence, class-incremental learning (CIL) is necessary, which incrementally and rapidly updates an existing model with the data of new classes while retaining the existing knowledge of old classes. However, most current CIL methods are designed based on deep models that require a computationally expensive training and update process. In addition, deep learning based CIL (DCIL) methods typically employ stochastic gradient descent (SGD) as an optimizer that forgets the old knowledge to a certain extent. In this article, a broad learning system-based CIL (BLS-CIL) method with fast update and high retainability of old class knowledge is proposed. Traditional BLS is a fast and effective shallow neural network, but it does not work well on CIL tasks. However, our proposed BLS-CIL can overcome these issues and provide the following: 1) high accuracy due to our novel class-correlation loss function that considers the correlations between old and new classes; 2) significantly short training/update time due to the newly derived closed-form solution for our class-correlation loss without iterative optimization; and 3) high retainability of old class knowledge due to our newly derived recursive update rule for CIL (RULL) that does not replay the exemplars of all old classes, as contrasted to the exemplars-replaying methods with the SGD optimizer. The proposed BLS-CIL has been evaluated over 12 real-world datasets, including seven tabular/numerical datasets and six image datasets, and the compared methods include one shallow network and seven classical or state-of-the-art DCIL methods. Experimental results show that our BIL-CIL can significantly improve the classification performance over a shallow network by a large margin (8.80%-48.42%). It also achieves comparable or even higher accuracy than DCIL methods, but greatly reduces the training time from hours to minutes and the update time from minutes to seconds.
更多查看译文
关键词
Training,Task analysis,Learning systems,Data models,Predictive models,Correlation,Support vector machines,Broad learning system (BLS),catastrophic forgetting,class correlations,class-incremental learning (CIL),recursive update rule
AI 理解论文
溯源树
样例
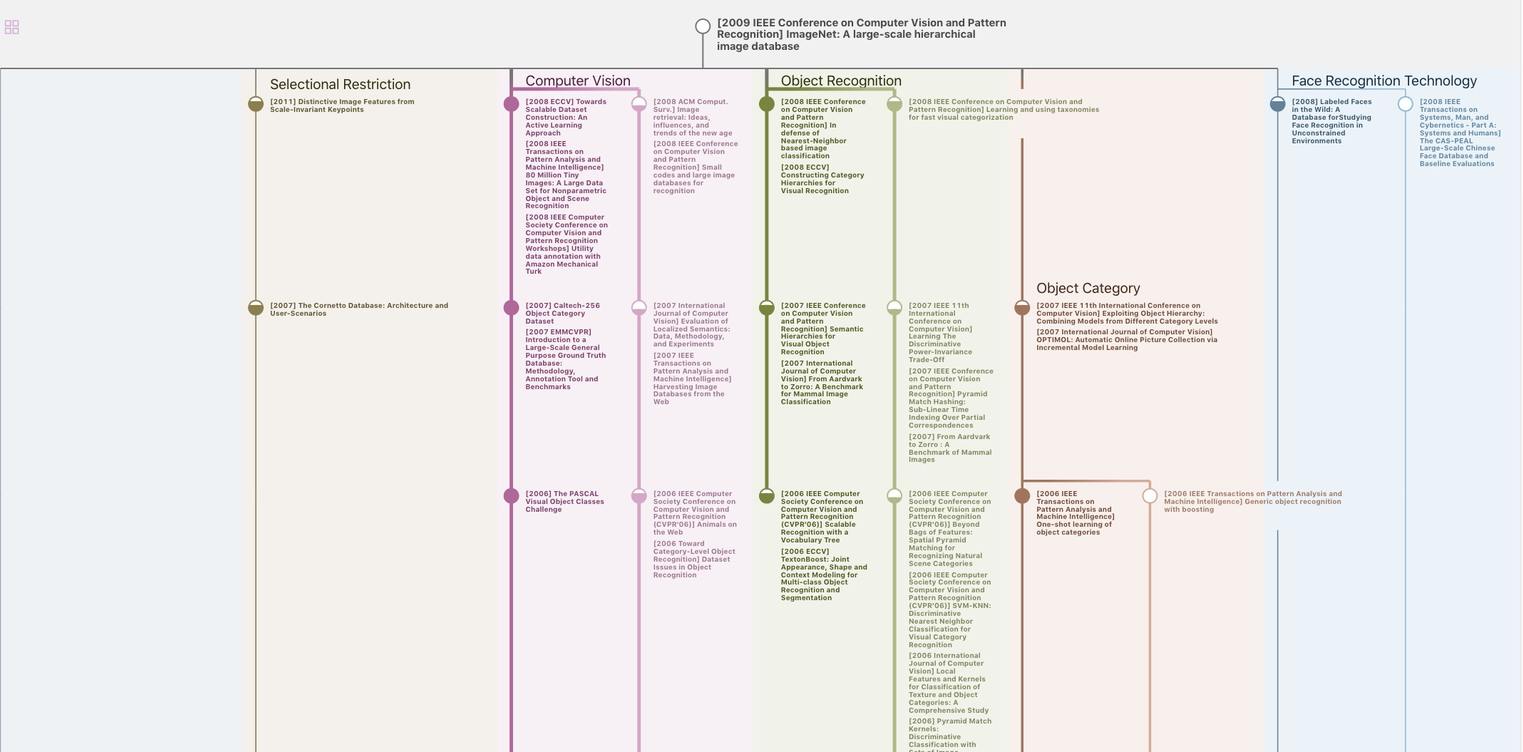
生成溯源树,研究论文发展脉络
Chat Paper
正在生成论文摘要