RMAU-Net: Residual Multi-Scale Attention U-Net For liver and tumor segmentation in CT images.
Computers in biology and medicine(2023)
摘要
Liver cancer is one of the leading causes of cancer-related deaths worldwide. Automatic liver and tumor segmentation are of great value in clinical practice as they can reduce surgeons' workload and increase the probability of success in surgery. Liver and tumor segmentation is a challenging task because of the different sizes, shapes, blurred boundaries of livers and lesions, and low-intensity contrast between organs within patients. To address the problem of fuzzy livers and small tumors, we propose a novel Residual Multi-scale Attention U-Net (RMAU-Net) for liver and tumor segmentation by introducing two modules, i.e., Res-SE-Block and MAB. The Res-SE-Block can mitigate the problem of gradient disappearance by residual connection and enhance the quality of representations by explicitly modeling the interdependencies and feature recalibration between the channels of features. The MAB can exploit rich multi-scale feature information and capture inter-channel and inter-spatial relationships of features simultaneously. In addition, a hybrid loss function, that combines focal loss and dice loss, is designed to improve segmentation accuracy and speed up convergence. We evaluated the proposed method on two publicly available datasets, i.e., LiTS and 3D-IRCADb. Our proposed method achieved better performance than the other state-of-the-art methods, with dice scores of 0.9552 and 0.9697 for LiTS and 3D-IRCABb liver segmentation, and dice scores of 0.7616 and 0.8307 for LiTS and 3D-IRCABb liver tumor segmentation.
更多查看译文
关键词
Attention mechanism,Deep learning,Liver and tumor segmentation,Medical imaging,Multi-scale feature
AI 理解论文
溯源树
样例
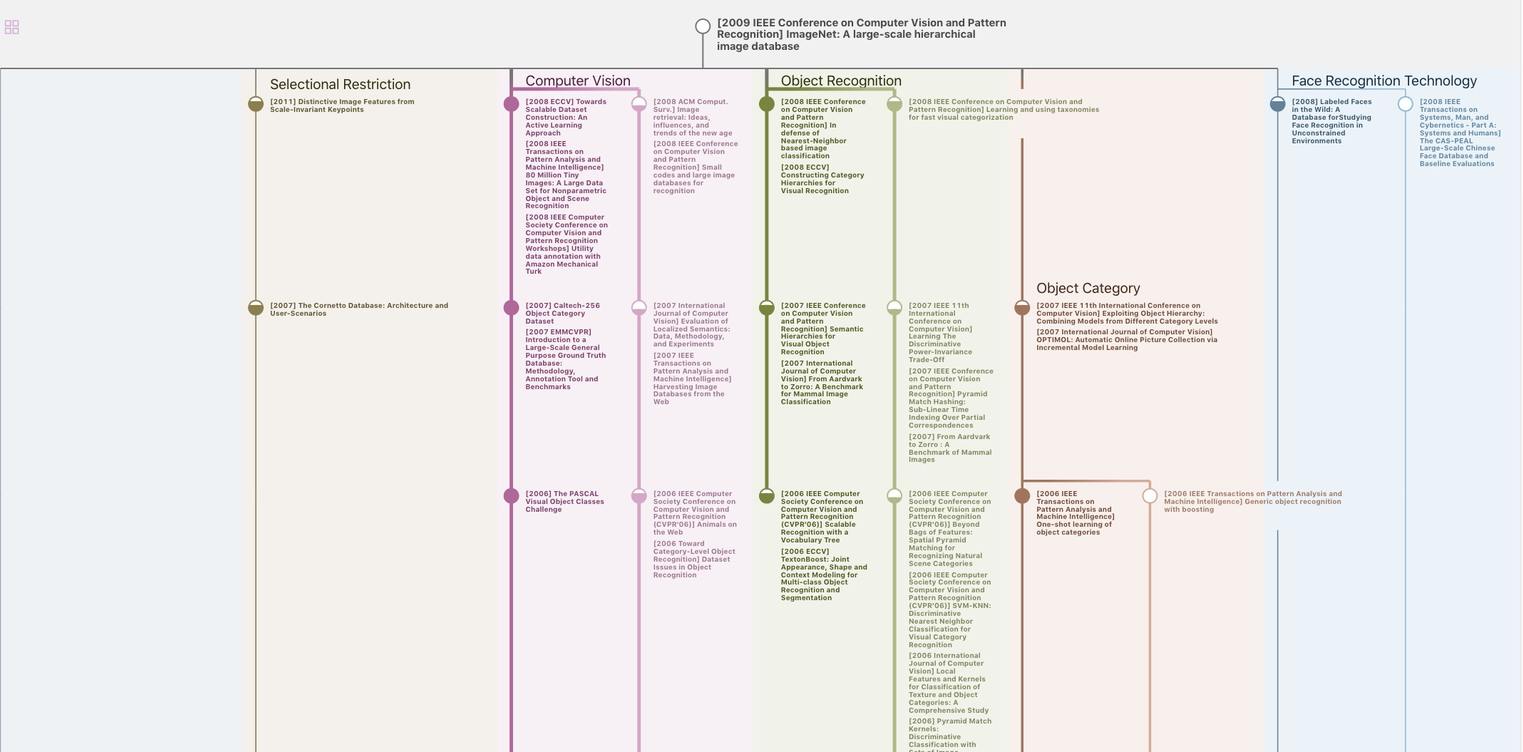
生成溯源树,研究论文发展脉络
Chat Paper
正在生成论文摘要