A Novel Regularization Paradigm for the Extreme Learning Machine
Neural Processing Letters(2023)
摘要
Due to its fast training speed and powerful approximation capabilities, the extreme learning machine (ELM) has generated a lot of attention in recent years. However, the basic ELM still has some drawbacks, such as the tendency to over-fitting and the susceptibility to noisy data. By adding a regularization term to the basic ELM, the regularized extreme learning machine (R-ELM) can dramatically improve its generalization and stability. In the R-ELM, choosing an appropriate regularization parameter is critical since it can regulate the fitting and generalization capabilities of the model. In this paper, we propose the regularized functional extreme learning machine (RF-ELM), which employs the regularization functional instead of a preset regularization parameter for adaptively choosing appropriate regularization parameters. The regularization functional is defined according to output weights, and the successive approximation iterative algorithm is utilized to solve the output weights so that we can get their values simultaneously at each iteration step. We also developed a parallel version of RF-ELM (PRF-ELM) to deal with big data tasks. Furthermore, the analysis of convexity and convergence ensures the validity of the model training. Finally, the experiments on the function approximation and the UCI repository with or without noise data demonstrate the superiority and competitiveness of our proposed models.
更多查看译文
关键词
Extreme learning machine (ELM),Robustness,Generalization,Convexity,Convergence analysis
AI 理解论文
溯源树
样例
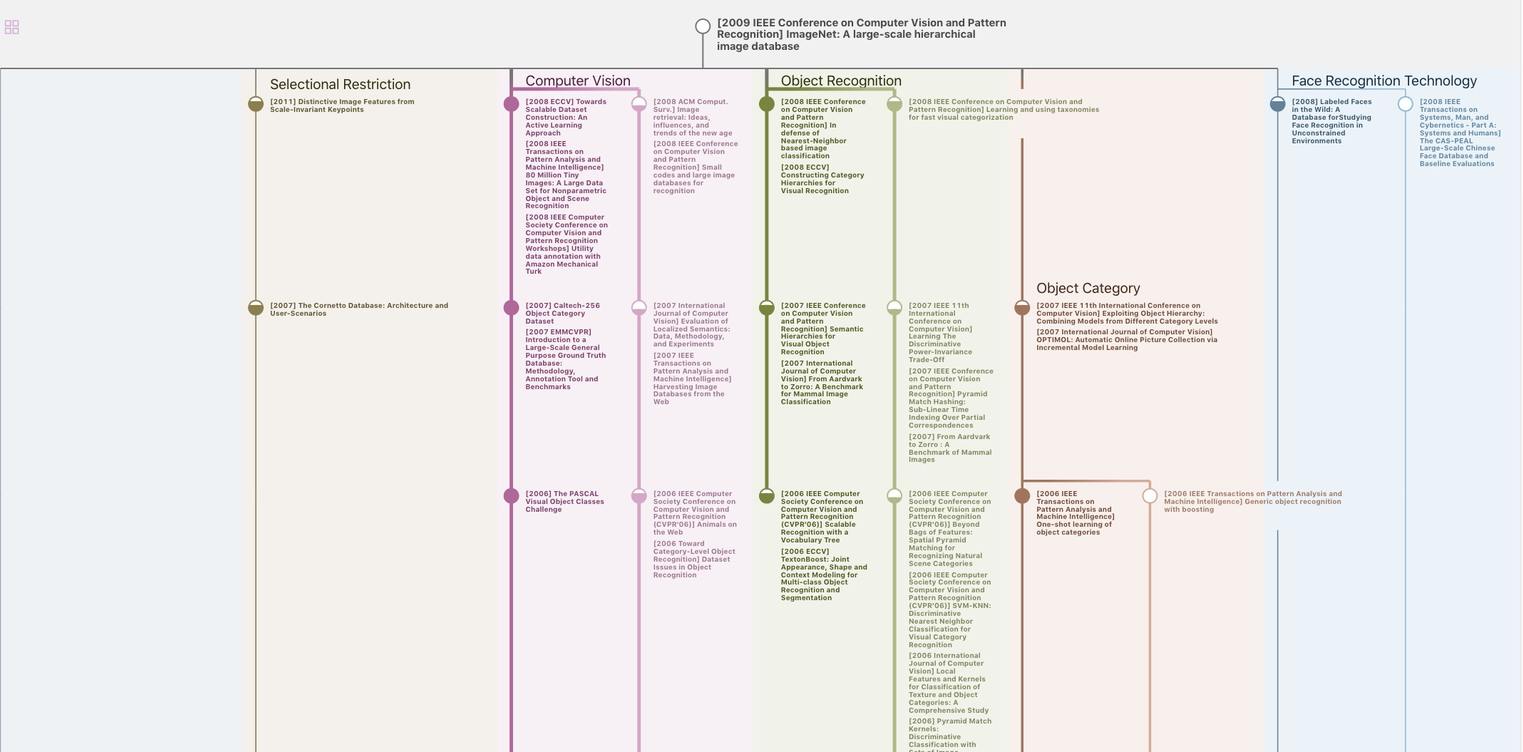
生成溯源树,研究论文发展脉络
Chat Paper
正在生成论文摘要