Online Learning for Scheduling MIP Heuristics
CoRR(2023)
摘要
Mixed Integer Programming (MIP) is NP-hard, and yet modern solvers often solve large real-world problems within minutes. This success can partially be attributed to heuristics. Since their behavior is highly instance-dependent, relying on hard-coded rules derived from empirical testing on a large heterogeneous corpora of benchmark instances might lead to sub-optimal performance. In this work, we propose an online learning approach that adapts the application of heuristics towards the single instance at hand. We replace the commonly used static heuristic handling with an adaptive framework exploiting past observations about the heuristic's behavior to make future decisions. In particular, we model the problem of controlling Large Neighborhood Search and Diving - two broad and complex classes of heuristics - as a multi-armed bandit problem. Going beyond existing work in the literature, we control two different classes of heuristics simultaneously by a single learning agent. We verify our approach numerically and show consistent node reductions over the MIPLIB 2017 Benchmark set. For harder instances that take at least 1000 seconds to solve, we observe a speedup of 4%.
更多查看译文
关键词
scheduling mip heuristics,online learning
AI 理解论文
溯源树
样例
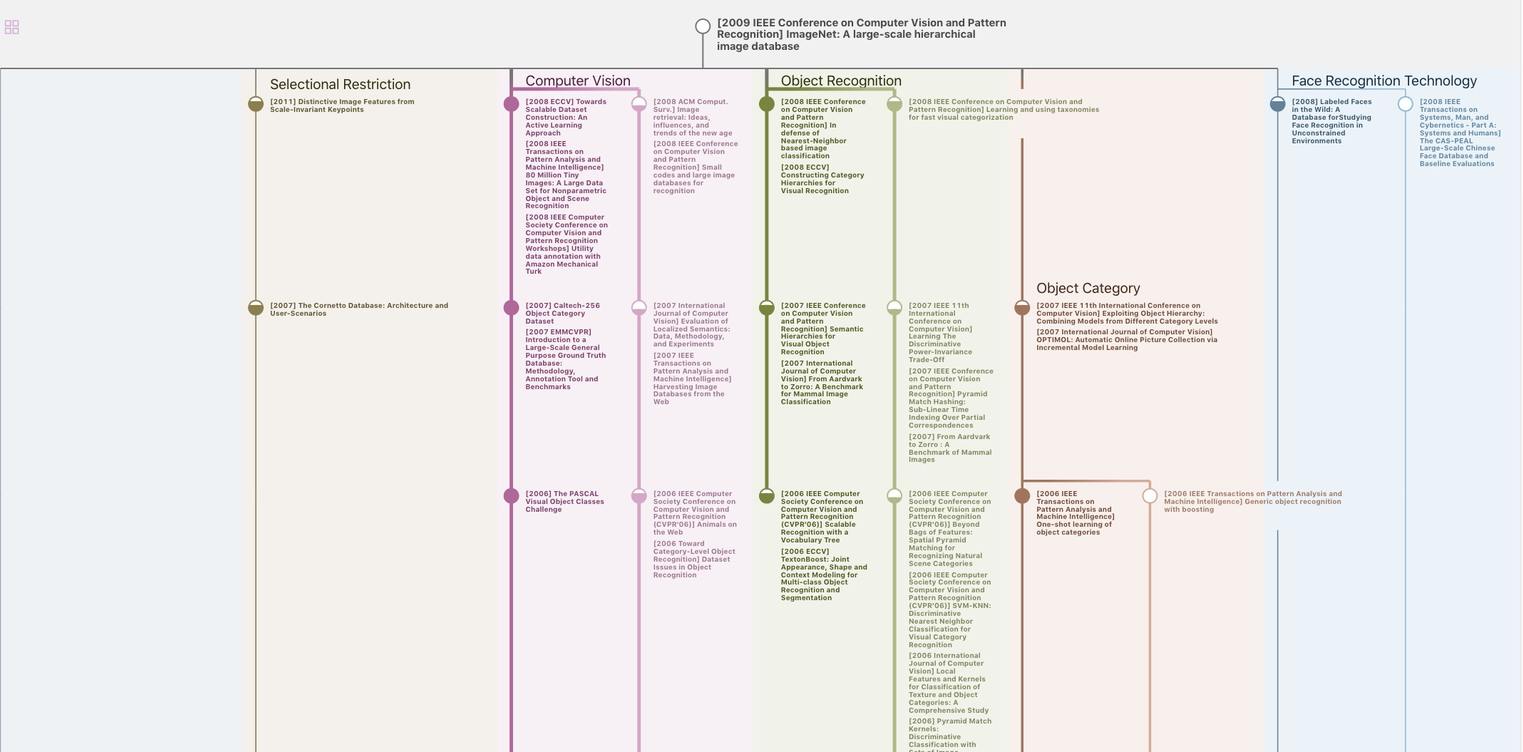
生成溯源树,研究论文发展脉络
Chat Paper
正在生成论文摘要