A physics-informed neural network framework for modeling obstacle-related equations
CoRR(2023)
摘要
Deep learning has been highly successful in some applications. Nevertheless, its use for solving partial differential equations (PDEs) has only been of recent interest with current state-of-the-art machine learning libraries, e.g., TensorFlow or PyTorch. Physics-informed neural networks (PINNs) are an attractive tool for solving partial differential equations based on sparse and noisy data. Here extend PINNs to solve obstacle-related PDEs which present a great computational challenge because they necessitate numerical methods that can yield an accurate approximation of the solution that lies above a given obstacle. The performance of the proposed PINNs is demonstrated in multiple scenarios for linear and nonlinear PDEs subject to regular and irregular obstacles.
更多查看译文
关键词
neural network framework,neural network,modeling
AI 理解论文
溯源树
样例
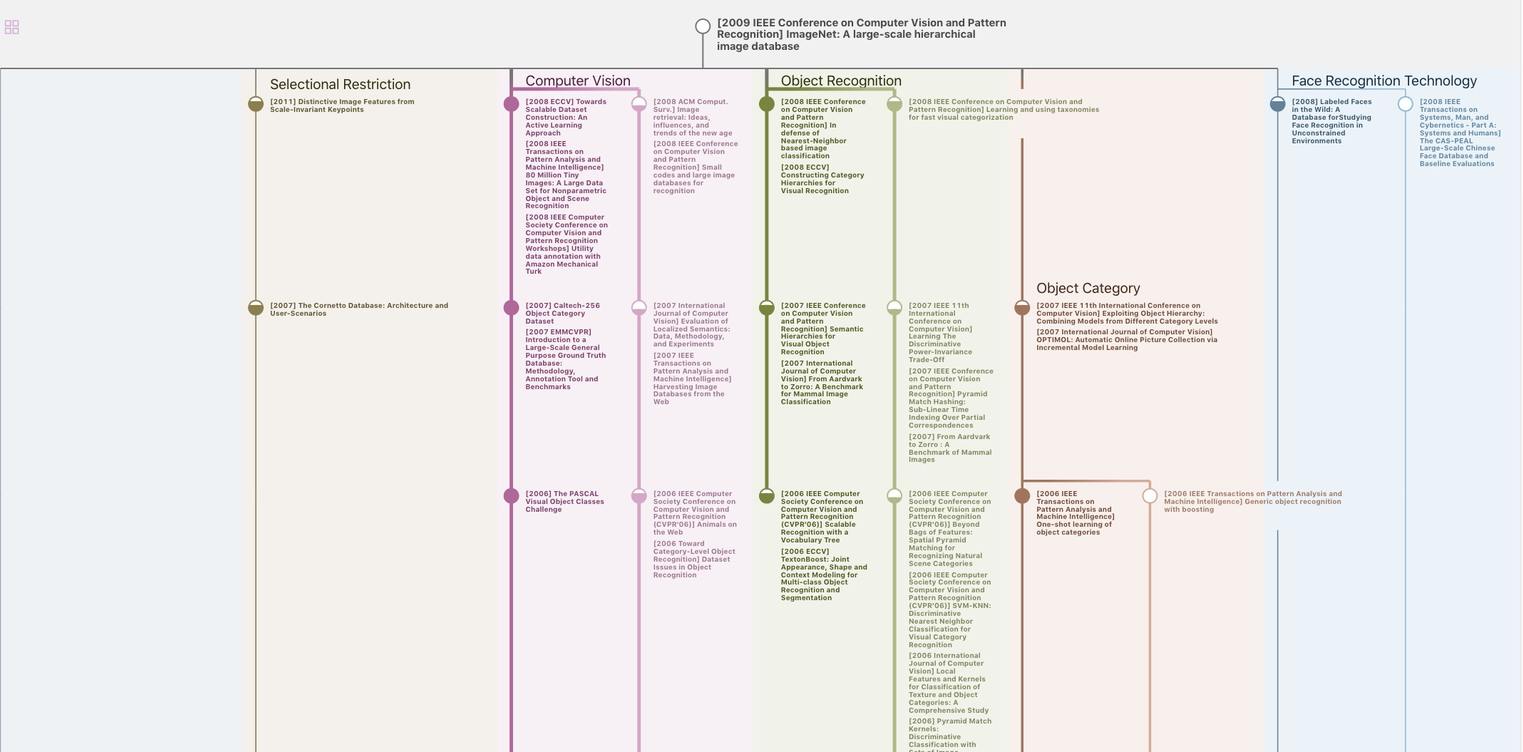
生成溯源树,研究论文发展脉络
Chat Paper
正在生成论文摘要