A Riemannian Framework for Structurally Curated Functional Clustering of Brain White Matter Fibers
IEEE transactions on medical imaging(2023)
摘要
White matter (WM) consists of fibers that transmit information from one brain region to another, and functional fiber clustering that combines diffusion and functional MRI provides a novel perspective for exploring the functional architecture of axonal fibers. However, existing methods only concern functional signals in gray matter (GM), whereas the connecting fibers may not transmit relevant functional signals. There has been growing evidence that neural activity is encoded in WM BOLD signals as well, which provides rich multimodal information for fiber clustering. In this paper, we develop a comprehensive Riemannian framework for functional fiber clustering using WM BOLD signals along fibers. Specifically, we derive a novel metric that is highly discriminative of different functional classes while reducing the variability within classes and, in the meantime, enables low-dimensional coding of high-dimensional data. Our in vivo experiments show that the proposed framework is able to achieve clustering results with inter-subject consistency and functional homogeneity. In addition, we develop an atlas of WM functional architecture for standardizable yet flexible use and exemplify a machine-learning-based application for the classification of autism spectrum disorders, which further demonstrates the great potential of our approach in practical applications.
更多查看译文
关键词
Functional clustering,WM bold signal,riemannian framework,tract-based analysis,autism
AI 理解论文
溯源树
样例
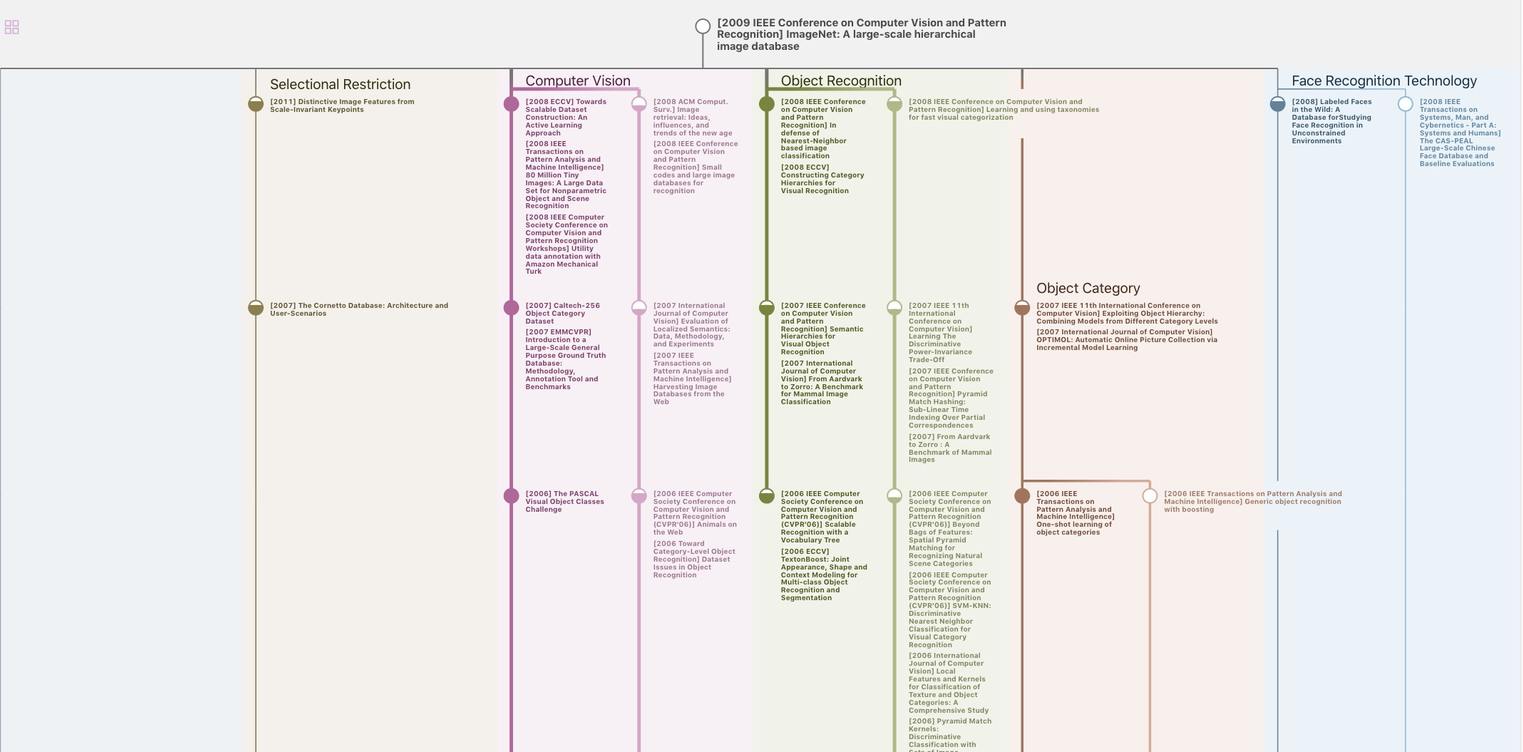
生成溯源树,研究论文发展脉络
Chat Paper
正在生成论文摘要