Multiagent Reinforcement Learning With Graphical Mutual Information Maximization.
IEEE transactions on neural networks and learning systems(2023)
Abstract
Communication learning is an important research direction in the multiagent reinforcement learning (MARL) domain. Graph neural networks (GNNs) can aggregate the information of neighbor nodes for representation learning. In recent years, several MARL methods leverage GNN to model information interactions between agents to coordinate actions and complete cooperative tasks. However, simply aggregating the information of neighboring agents through GNNs may not extract enough useful information, and the topological relationship information is ignored. To tackle this difficulty, we investigate how to efficiently extract and utilize the rich information of neighbor agents as much as possible in the graph structure, so as to obtain high-quality expressive feature representation to complete the cooperation task. To this end, we present a novel GNN-based MARL method with graphical mutual information (MI) maximization to maximize the correlation between input feature information of neighbor agents and output high-level hidden feature representations. The proposed method extends the traditional idea of MI optimization from graph domain to multiagent system, in which the MI is measured from two aspects: agent features information and agent topological relationships. The proposed method is agnostic to specific MARL methods and can be flexibly integrated with various value function decomposition methods. Considerable experiments on various benchmarks demonstrate that the performance of our proposed method is superior to the existing MARL methods.
MoreTranslated text
Key words
Communication learning,graph neural net-work (GNN),multiagent reinforcement learning (MARL),mutual information (MI)
AI Read Science
Must-Reading Tree
Example
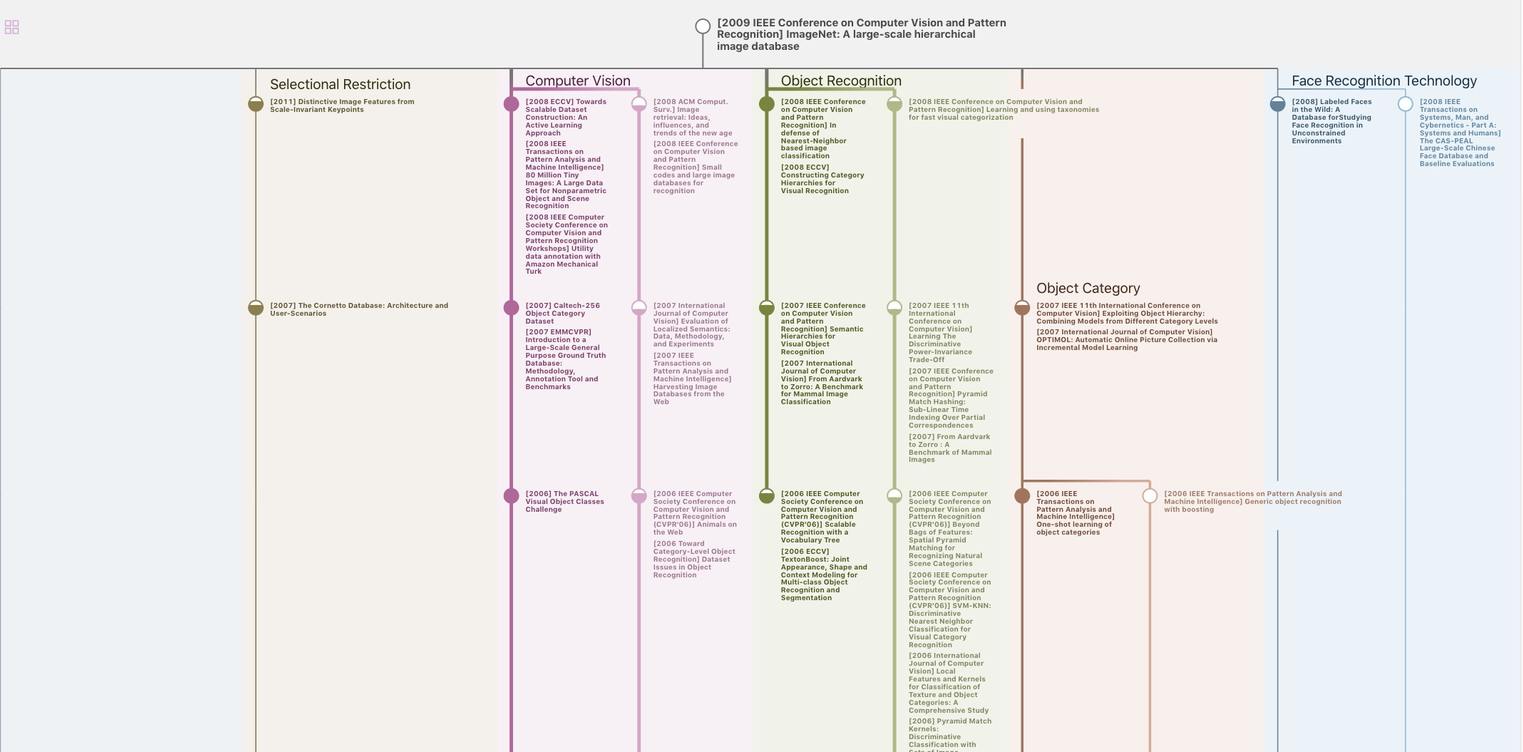
Generate MRT to find the research sequence of this paper
Chat Paper
Summary is being generated by the instructions you defined