Continuous-Time Reinforcement Learning Control: A Review of Theoretical Results, Insights on Performance, and Needs for New Designs
IEEE transactions on neural networks and learning systems(2023)
摘要
This exposition discusses continuous-time reinforcement learning (CT-RL) for the control of affine nonlinear systems. We review four seminal methods that are the centerpieces of the most recent results on CT-RL control. We survey the theoretical results of the four methods, highlighting their fundamental importance and successes by including discussions on problem formulation, key assumptions, algorithm procedures, and theoretical guarantees. Subsequently, we evaluate the performance of the control designs to provide analyses and insights on the feasibility of these design methods for applications from a control designer's point of view. Through systematic evaluations, we point out when theory diverges from practical controller synthesis. We, furthermore, introduce a new quantitative analytical framework to diagnose the observed discrepancies. Based on the analyses and the insights gained through quantitative evaluations, we point out potential future research directions to unleash the potential of CT-RL control algorithms in addressing the identified challenges.
更多查看译文
关键词
Optimal control,Mathematical models,Heuristic algorithms,Convergence,Tuning,Recurrent neural networks,Power system stability,Adaptive,approximate dynamic programming (ADP),continuous-time (CT),optimal control,policy iteration (PI),reinforcement learning (RL),value iteration (VI)
AI 理解论文
溯源树
样例
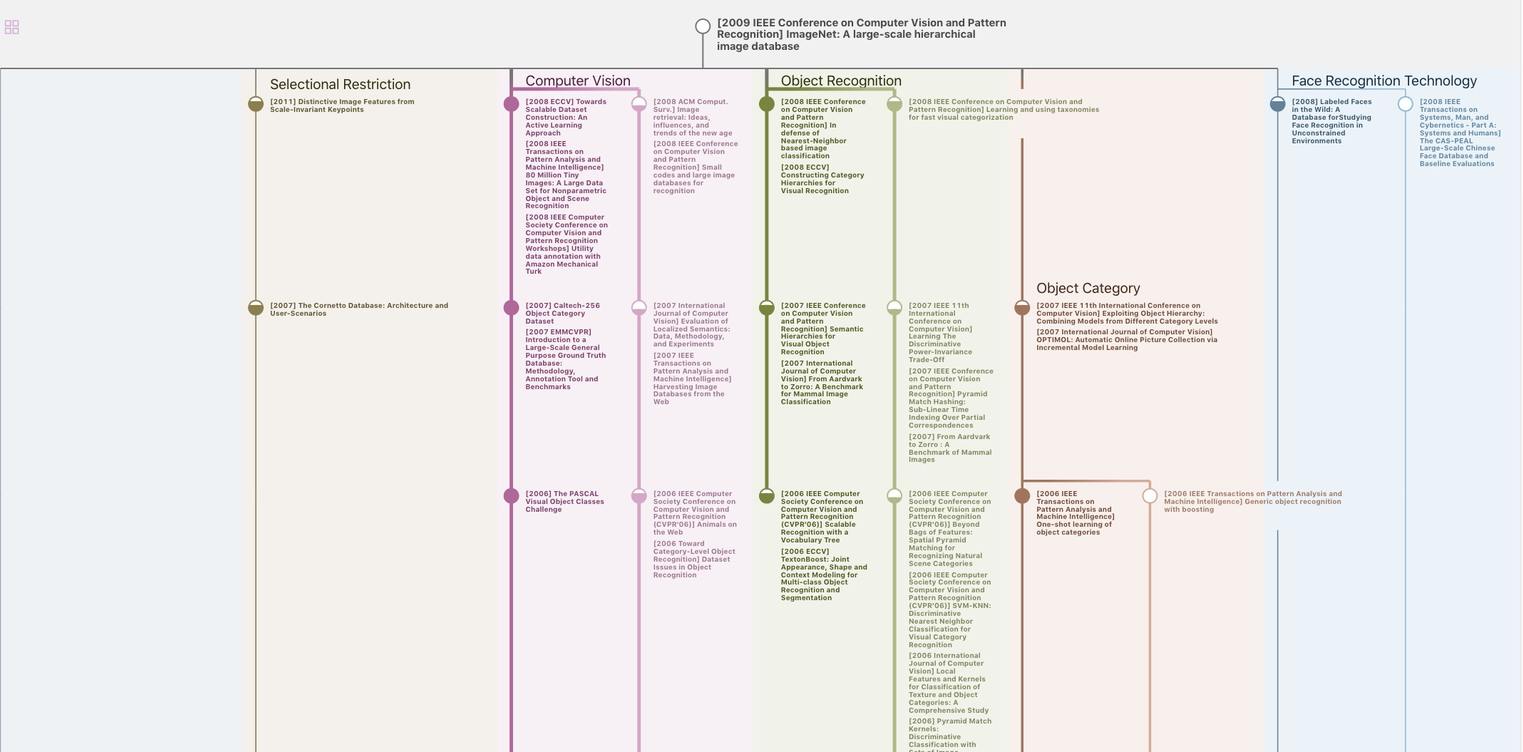
生成溯源树,研究论文发展脉络
Chat Paper
正在生成论文摘要