Predictive power of non-identifiable models
bioRxiv (Cold Spring Harbor Laboratory)(2023)
摘要
Resolving practical nonidentifiability of computational models typically requires either additional data or non-algorithmic model reduction, which frequently results in models containing parameters lacking direct interpretation. Here, instead of reducing models, we explore an alternative, Bayesian approach, and quantify predictive power of non-identifiable models. Considering an example biochemical signalling cascade model as well as its mechanical analog, we demonstrate that by measuring a single variable in response to a properly chosen stimulation protocol, the dimensionality of the parameter space is reduced, which allows for prediction of its trajectory in response to different stimulation protocols even if all model parameters remain unidentified. Successive measurements of remaining variables further constrain model parameters and enable more predictions. We analyse potential pitfalls of the proposed approach that can arise when the investigated model is oversimplified, incorrect, or when the training protocol is inadequate. The main advantage of the suggested iterative approach is that the predictive power of the model can be assessed and practically utilised at each step.
更多查看译文
关键词
predictive power,models,non-identifiable
AI 理解论文
溯源树
样例
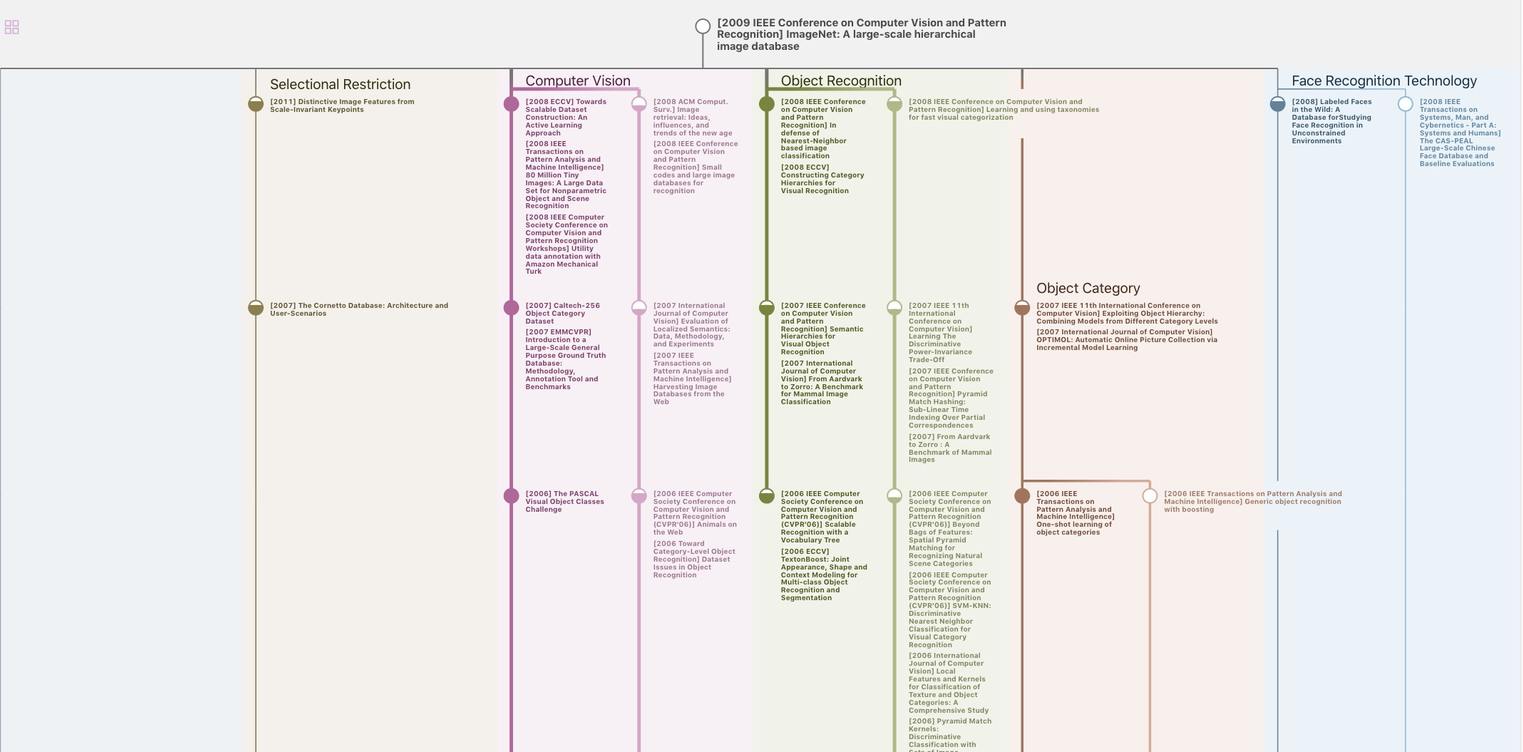
生成溯源树,研究论文发展脉络
Chat Paper
正在生成论文摘要