scENT for Revealing Gene Clusters From Single-Cell RNA-Seq Data
IEEE/ACM Transactions on Computational Biology and Bioinformatics(2023)
摘要
Recently, the fast development of single-cell RNA-seq (scRNA-seq) techniques has enabled high-resolution transcriptomic statistical analysis of individual cells in heterogeneous tissues, which can help researchers to explore the relationship between genes and human diseases. The emerging scRNA-seq data results in new analysis methods aiming to identify cell-level clustering and annotations. However, there are few methods developed to gain insights into the gene-level clusters with biological significance. This study proposes a new deep learning-based framework, scENT (single cell gENe clusTer), to identify significant gene clusters from single-cell RNA-seq data. We started with clustering the scRNA-seq data into multiple optimal groups, followed by a gene set enrichment analysis to identify classes of over-represented genes. Considering high-dimensional data with extensive zeros and dropout issues, scENT integrates perturbation in the learning process of clustering scRNA-seq data to improve its robustness and performance. Experimental results show that scENT outperformed other benchmarking methods on simulation data. To validate the biological insights of scENT, we applied it to the public experimental scRNA-seq data profiled from patients with Alzheimer's disease and brain metastasis. scENT successfully identified novel functional gene clusters and associated functions, facilitating the discovery of prospective mechanisms and the understanding of related diseases.
更多查看译文
关键词
Alzheimer's disease,clustering,deep learning,gene set enrichment analysis,scRNA-seq data
AI 理解论文
溯源树
样例
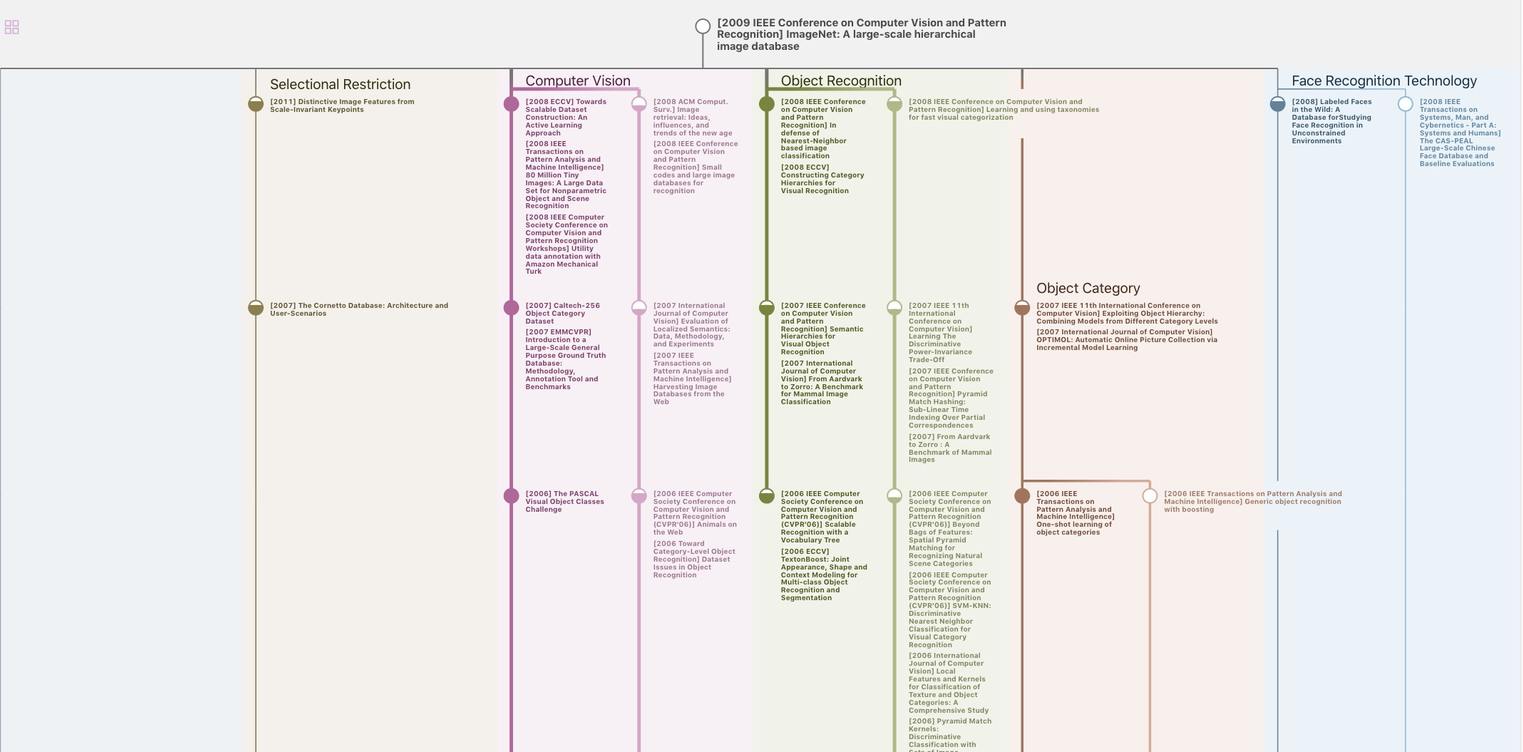
生成溯源树,研究论文发展脉络
Chat Paper
正在生成论文摘要