mFILS: Tri-Selection via Convex and Nonconvex Regularizations.
IEEE transactions on neural networks and learning systems(2023)
摘要
In many real-world applications, data are represented by multiple instances and simultaneously associated with multiple labels. These data are always redundant and generally contaminated by different noise levels. As a result, several machine learning models fail to achieve good classification and find an optimal mapping. Feature selection, instance selection, and label selection are three effective dimensionality reduction techniques. Nevertheless, the literature was limited to feature and/or instance selection but has, to some extent, neglected label selection, which also plays an essential role in the preprocessing step, as label noises can adversely affect the performance of the underlying learning algorithms. In this article, we propose a novel framework termed multilabel Feature Instance Label Selection (mFILS) that simultaneously performs feature, instance, and label selections in both convex and nonconvex scenarios. To the best of our knowledge, this article offers, for the first time ever, a study using the triple and simultaneous selection of features, instances, and labels based on convex and nonconvex penalties in a multilabel scenario. Experimental results are built on some known benchmark datasets to validate the effectiveness of the proposed mFILS.
更多查看译文
关键词
convex,mfils,tri-selection
AI 理解论文
溯源树
样例
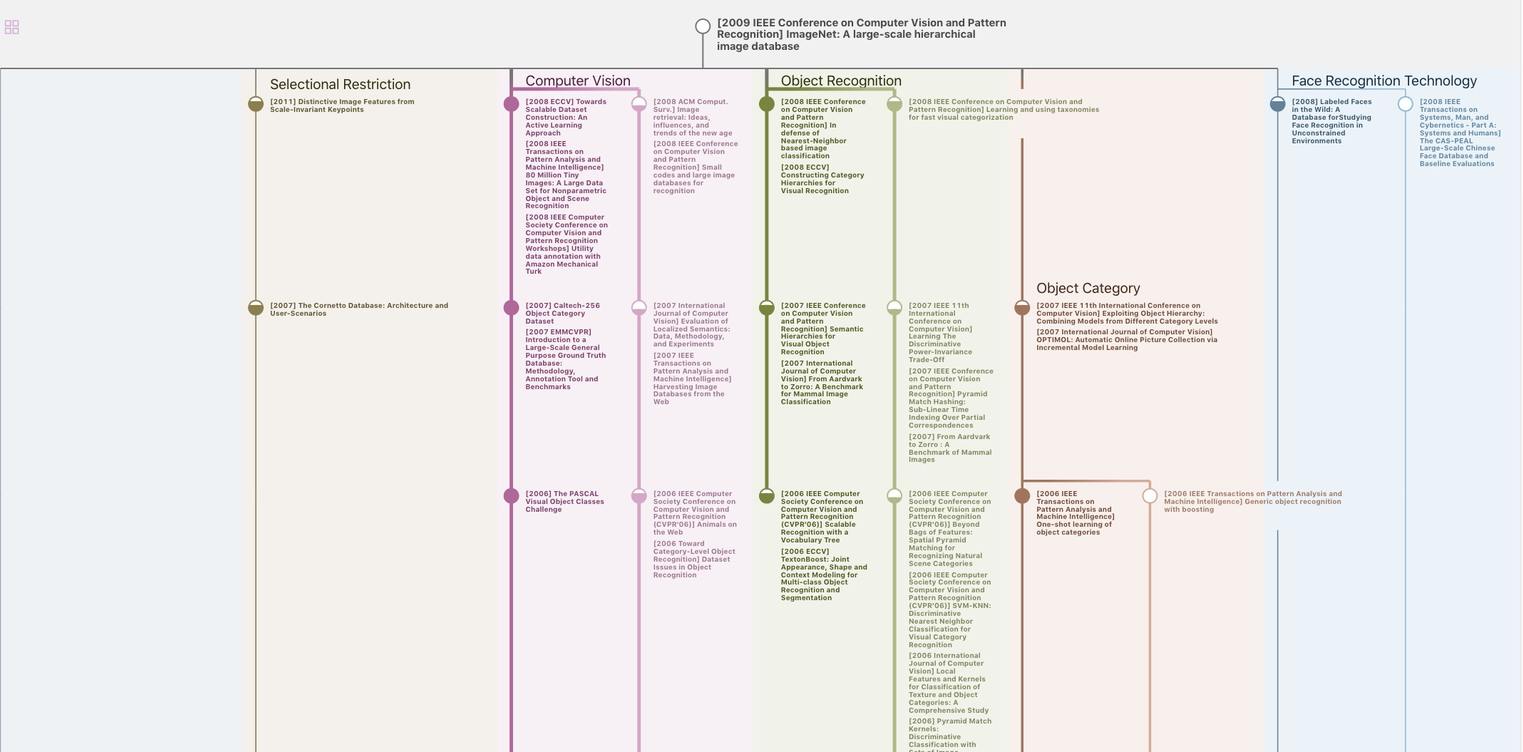
生成溯源树,研究论文发展脉络
Chat Paper
正在生成论文摘要