Synthetic Hard Negative Samples for Contrastive Learning
Neural Processing Letters(2024)
摘要
Contrastive learning has emerged as an essential approach in self-supervised visual representation learning. Its main goal is to maximize the similarities between augmented versions of the same image (positive pairs), while minimizing the similarities between different images (negative pairs). Recent studies have demonstrated that harder negative samples, i.e., those that are more challenging to differentiate from the anchor sample perform a more crucial function in contrastive learning. However, many existing contrastive learning methods ignore the role of hard negative samples. In order to provide harder negative samples for the network model more efficiently. This paper proposes a novel feature-level sample sampling method, namely sampling synthetic hard negative samples for contrastive learning (SSCL). Specifically, we generate more and harder negative samples by mixing them through linear combination and ensure their reliability by debiasing. Finally, we execute weighted sampling of these negative samples. Compared to state-of-the-art methods, our method can provide more high-quality negative samples. Experiments show that SSCL improves the classification performance on different image datasets and can be readily integrated into existing methods.
更多查看译文
关键词
Self-supervised learning,Contrastive learning,Sampling negative samples,Synthetic hard negative samples
AI 理解论文
溯源树
样例
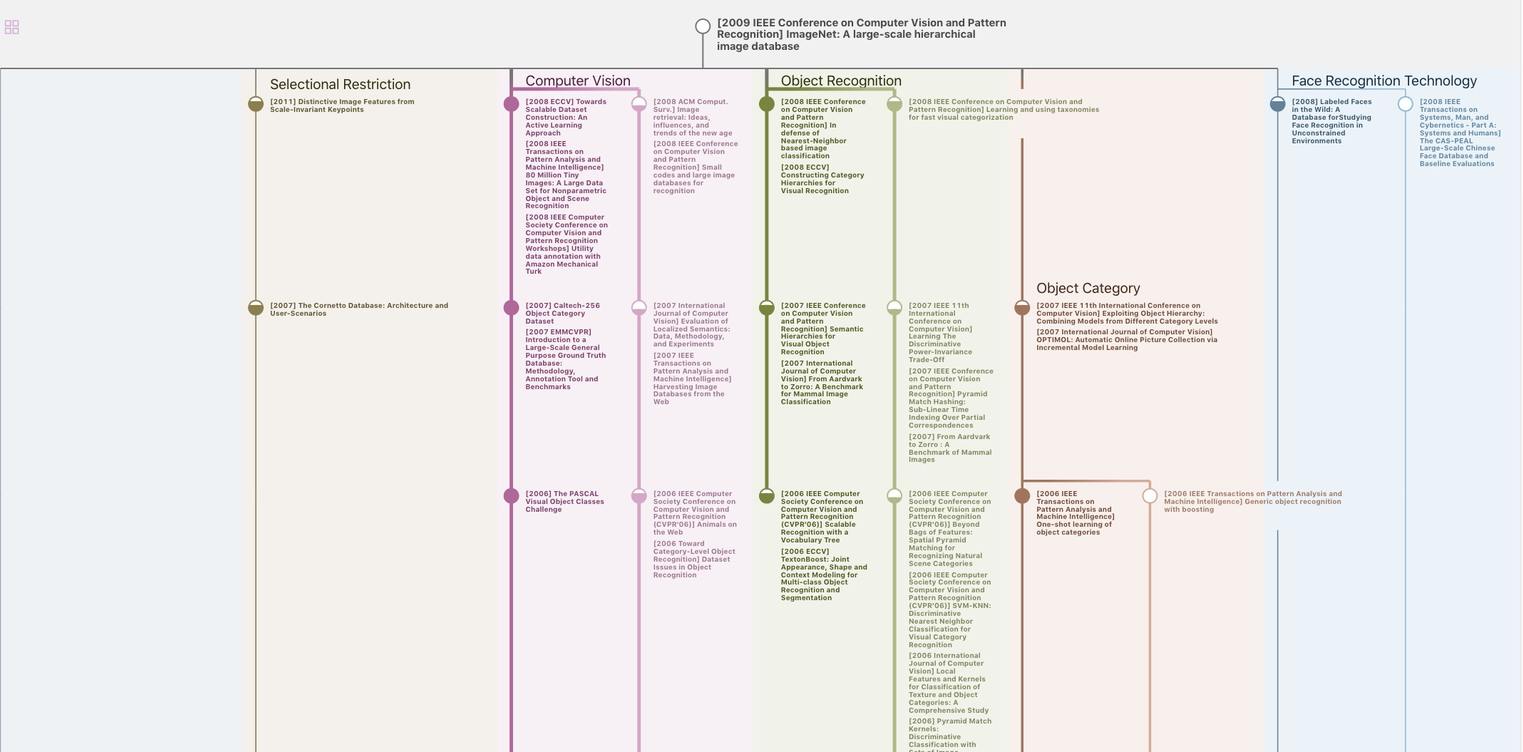
生成溯源树,研究论文发展脉络
Chat Paper
正在生成论文摘要