Position-Aware Relational Transformer for Knowledge Graph Embedding
IEEE TRANSACTIONS ON NEURAL NETWORKS AND LEARNING SYSTEMS(2023)
摘要
Although Transformer has achieved success in language and vision tasks, its capacity for knowledge graph (KG) embedding has not been fully exploited. Using the self-attention (SA) mechanism in Transformer to model the subject-relation-object triples in KGs suffers from training inconsistency as SA is invariant to the order of input tokens. As a result, it is unable to distinguish a (real) relation triple from its shuffled (fake) variants (e.g., object-relation-subject) and, thus, fails to capture the correct semantics. To cope with this issue, we propose a novel Transformer architecture, namely, for KG embedding. It incorporates relational compositions in entity representations to explicitly inject semantics and capture the role of an entity based on its position (subject or object) in a relation triple. The relational composition for a subject (or object) entity of a relation triple refers to an operator on the relation and the object (or subject). We borrow ideas from the typical translational and semantic-matching embedding techniques to design relational compositions. We carefully design a residual block to integrate relational compositions into SA and efficiently propagate the composed relational semantics layer by layer. We formally prove that the SA with relational compositions is able to distinguish the entity roles in different positions and correctly capture relational semantics. Extensive experiments and analyses on six benchmark datasets show that achieves state-of-the-art performance on both link prediction and entity alignment.
更多查看译文
关键词
Entity alignment,knowledge graph (KG) embedding,link prediction,position encoding,Transformer
AI 理解论文
溯源树
样例
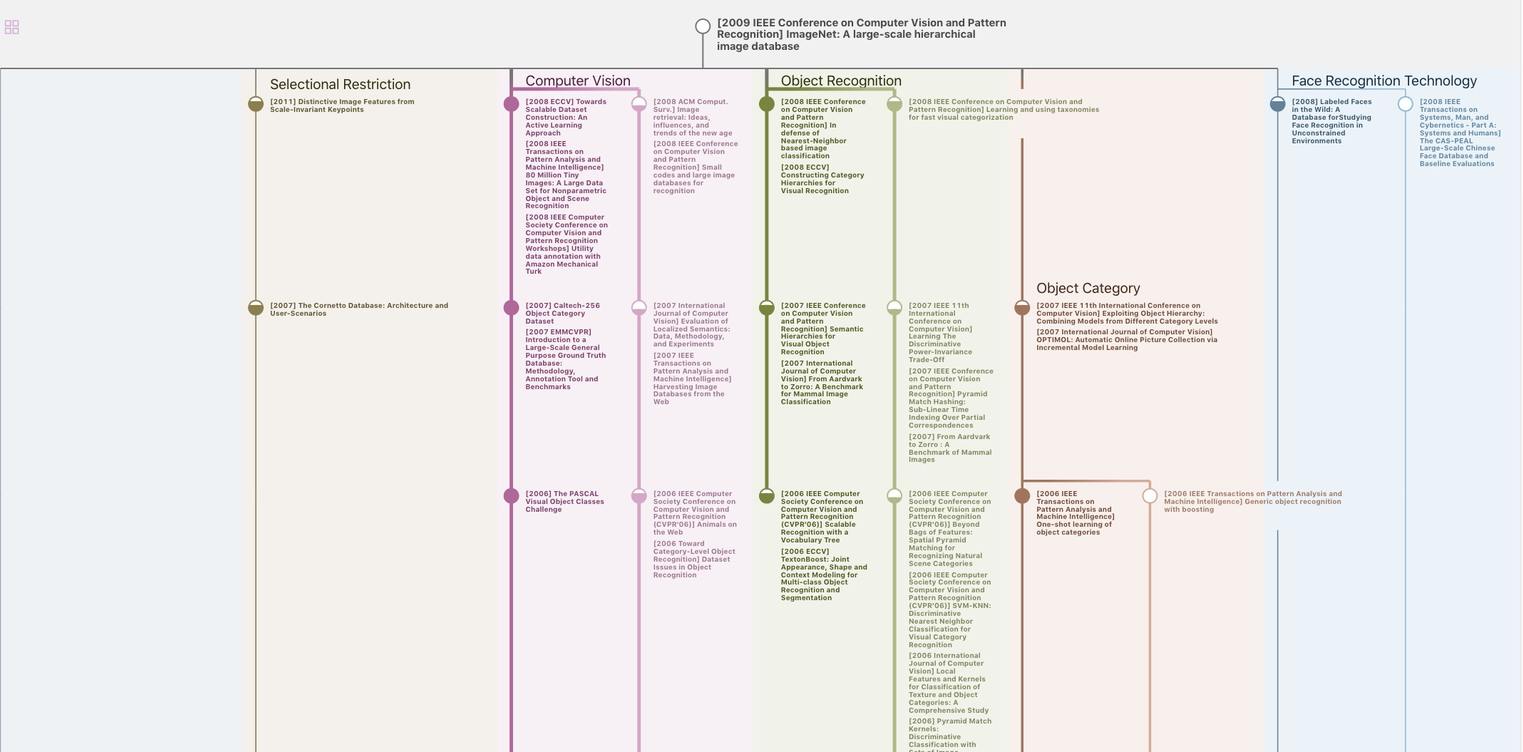
生成溯源树,研究论文发展脉络
Chat Paper
正在生成论文摘要