A sequential adaptive regularisation using cubics algorithm for solving nonlinear equality constrained optimization
Comput. Optim. Appl.(2023)
摘要
The adaptive regularisation algorithm using cubics (ARC) is initially proposed for unconstrained optimization. ARC has excellent convergence properties and complexity. In this paper, we extend ARC to solve nonlinear equality constrained optimization and propose a sequential adaptive regularisation using cubics algorithm inspired by sequential quadratic programming (SQP) methods. In each iteration of our method, the trial step is computed via composite-step approach, i.e., it is decomposed into the sum of normal step and tangential step. By means of reduced-Hessian approach, a new ARC subproblem for nonlinear equality constrained optimization is constructed to compute the tangential step, which can supply sufficient decrease required in the proposed algorithm. Once the trial step is obtained, the ratio of the penalty function reduction to the model function reduction is calculated to determine whether the trial point is accepted. The global convergence of the algorithm is investigated under some mild assumptions. Preliminary numerical experiments are reported to show the performance of the proposed algorithm.
更多查看译文
关键词
Nonlinear optimization,Constrained optimization,Adaptive regularization with cubics,Global convergence
AI 理解论文
溯源树
样例
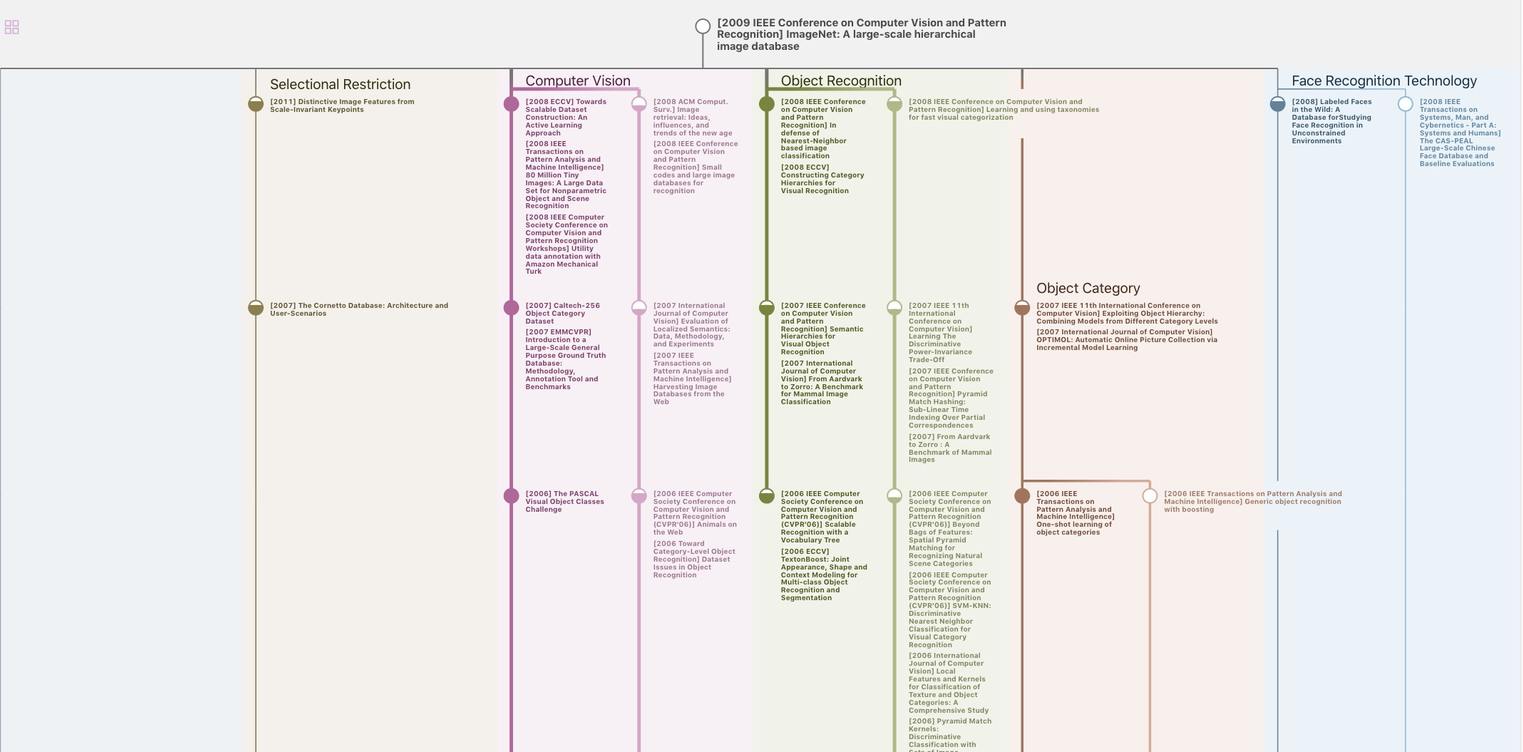
生成溯源树,研究论文发展脉络
Chat Paper
正在生成论文摘要