Classes versus Communities: Outlier Detection and Removal in Tabular Datasets via Social Network Analysis (ClaCO).
ASONAM(2022)
摘要
In this research, we introduce a model to detect inconsistent & anomalous samples in tabular labeled datasets which are used in machine learning classification tasks, frequently. Our model, abbreviated as the ClaCO (Classes vs. Communities: SNA for Outlier Detection), first converts tabular data with labels into an attributed and labeled undirected network graph. Following the enrichment of the graph, it analyses the edge structure of the individual egonets, in terms of the class and community belongings, by introducing a new SNA metric named as ‘the Consistency Score of a Node - CSoN’. Through an exhaustive analysis of the ego network of a node, CSoN tries to exhibit consistency of a node by examining the similarity of its immediate neighbors in terms of shared class and/or shared community belongings. To prove the efficiency of the proposed ClaCO, we employed it as a subsidiary method for detecting anomalous samples in the train part in the traditional ML classification task. With the help of this new consistency score, the least CSoN scored set of nodes flagged as outliers and removed from the training dataset, and remaining part fed into the ML model to see the effect on classification performance with the ‘whole’ dataset through competing outlier detection methods. We have shown this outlier detection model as an efficient method since it improves classification performance both on the whole dataset and reduced datasets with competing outlier detection methods, over several known both real-life and synthetic datasets.
更多查看译文
关键词
Social Network Analysis,supervised learning,graph-based outlier detection,structural outlier detection,downsampling of data
AI 理解论文
溯源树
样例
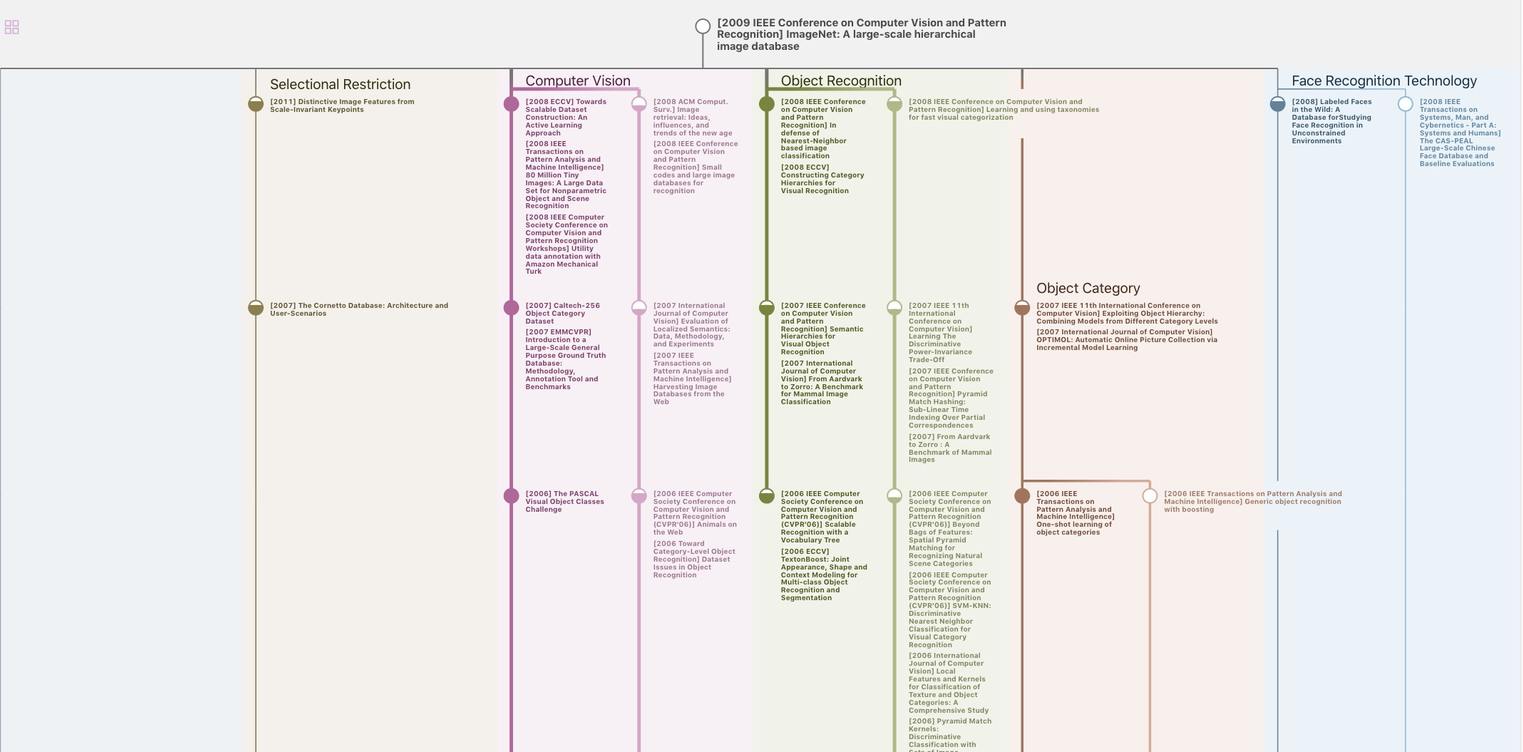
生成溯源树,研究论文发展脉络
Chat Paper
正在生成论文摘要