Breast Cancer Classification Using Equivariance Transition in Group Convolutional Neural Networks.
IEEE Access(2023)
Abstract
In computer vision, rotation equivariance and translation invariance are properties of a representation that preserve the geometric structure of a transformed input. These properties are achieved in Convolutional Neural Networks (CNNs) through data augmentation. However, achieving these properties remains a challenge. This is because CNNs are not equivariance under rotation. In this study, a novel deep neural network architecture combining a group convolutional neural network (G-CNN) built with a special Euclidean (SE2) motion group and discrete cosine transform (DCT) is proposed. The former is based on the group theory and uses SE2 to guarantee equivariance in 2D images, whereas the latter is used to encode and parameterize the model space. To restore and preserve the equivariance property of the transformed and convoluted images, the DCT was used as a rotation-invariant module. These combined techniques are employed to improve breast cancer classification and data efficiency in the CNNs processing pipeline. The developed model is tested on the rotated MNIST datasets to assess its performance. Finally, the model is applied to mammography images and achieved a high computational performance and improved inference generalization in breast cancer classification with an accuracy of 94.84%.
MoreTranslated text
Key words
Convolution, Convolutional neural networks, Breast cancer, Neural networks, Discrete cosine transforms, Computational modeling, Terminology, convolutional neural network, discrete cosine transform, group convolution, equivariance transition, image processing, mammography images
AI Read Science
Must-Reading Tree
Example
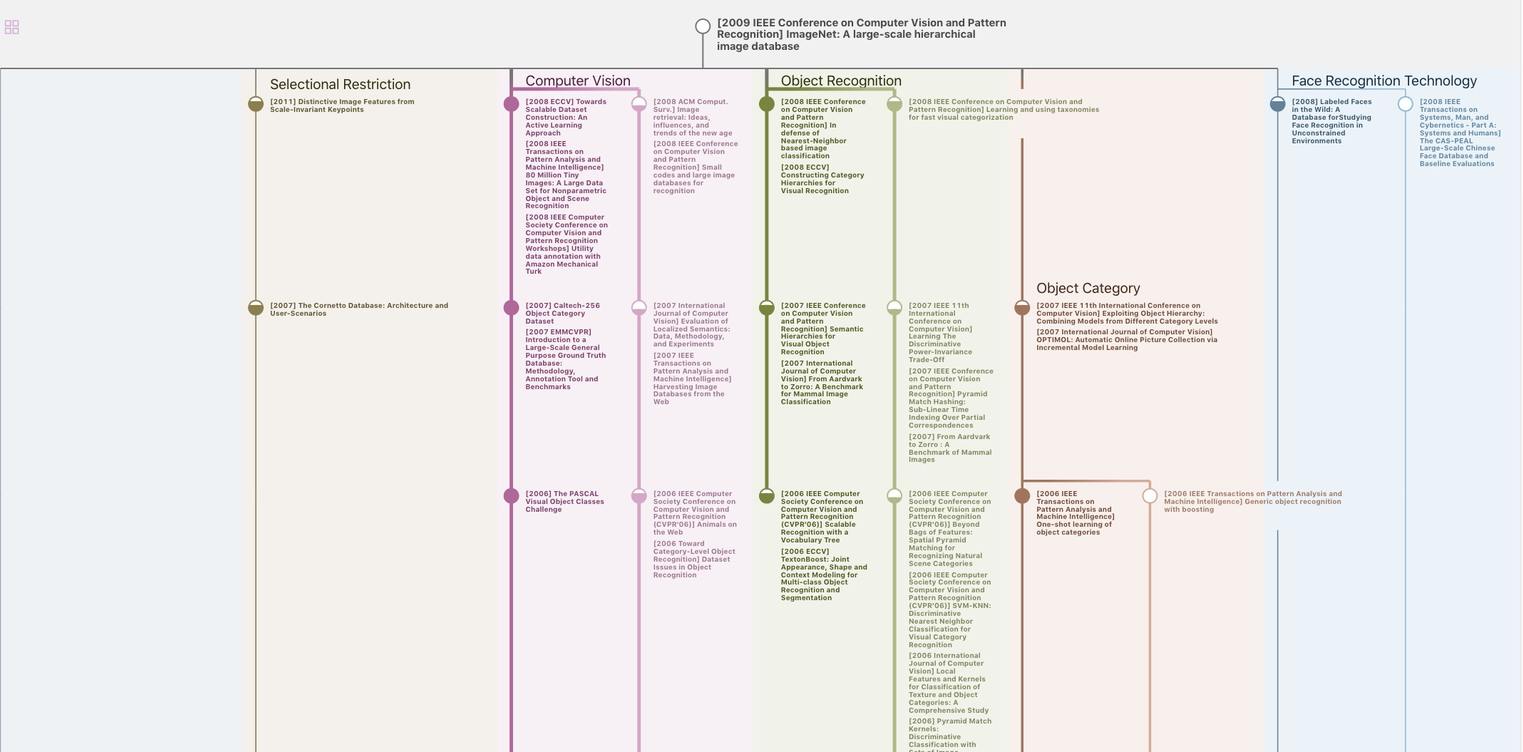
Generate MRT to find the research sequence of this paper
Chat Paper
Summary is being generated by the instructions you defined