A Self-Adaptive Approach to Exploit Topological Properties of Different GAs' Crossover Operators.
EuroGP(2023)
摘要
Evolutionary algorithms (EAs) are a family of optimization algorithms inspired by the Darwinian theory of evolution, and Genetic Algorithm (GA) is a popular technique among EAs. Similar to other EAs, common limitations of GAs have geometrical origins, like premature convergence, where the final population's convex hull might not include the global optimum. Population diversity maintenance is a central idea to tackle this problem but is often performed through methods that constantly diminish the search space's area. This work presents a self-adaptive approach, where the non-geometric crossover is strategically employed with geometric crossover to maintain diversity from a geometrical/topological perspective. To evaluate the performance of the proposed method, the experimental phase compares it against well-known diversity maintenance methods over well-known benchmarks. Experimental results clearly demonstrate the suitability of the proposed self-adaptive approach and the possibility of applying it to different types of crossover and EAs.
更多查看译文
关键词
exploit topological properties,crossover,operators,different gas,self-adaptive
AI 理解论文
溯源树
样例
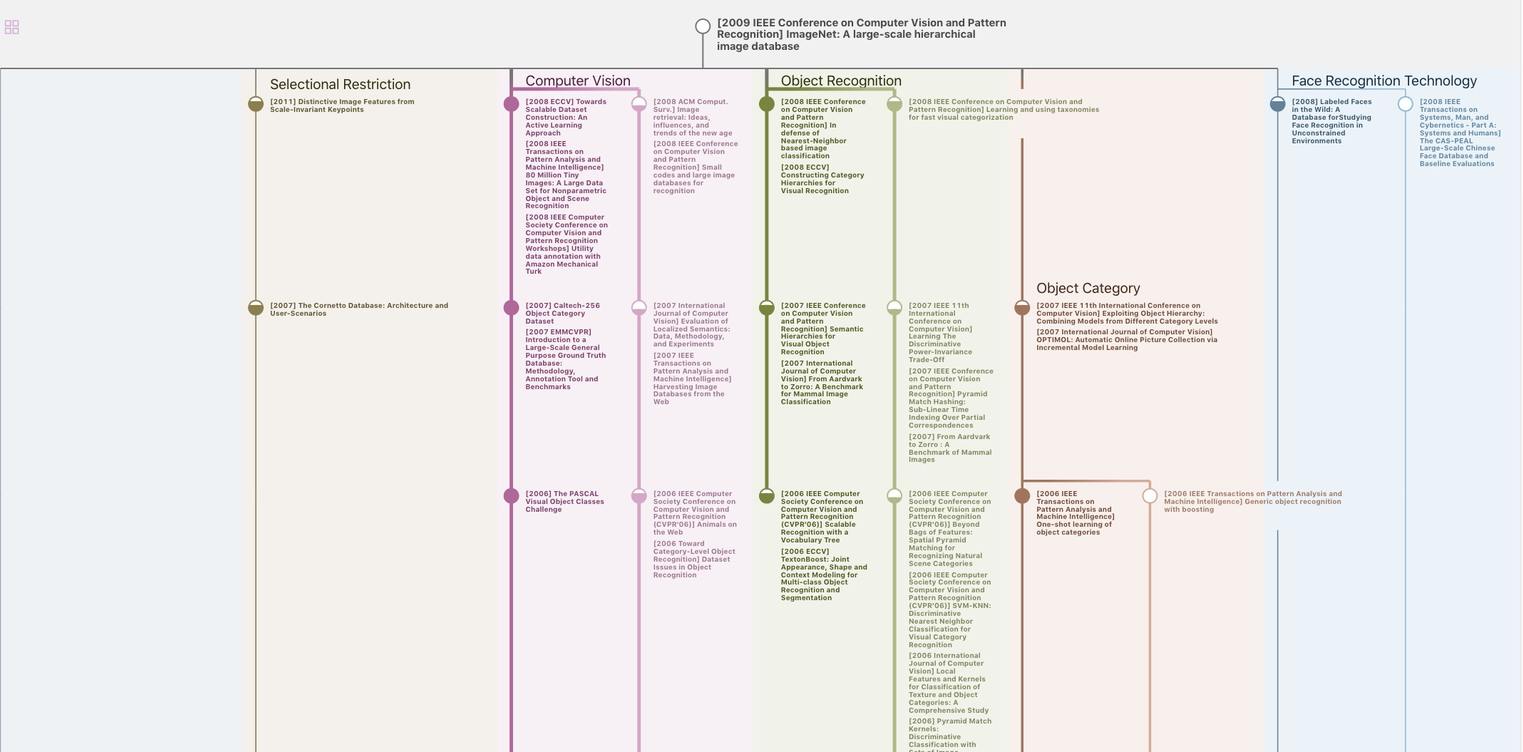
生成溯源树,研究论文发展脉络
Chat Paper
正在生成论文摘要