Ensemble-Based Data Assimilation of GPM DPR Reflectivity: Cloud Microphysics Parameter Estimation With the Nonhydrostatic Icosahedral Atmospheric Model (NICAM)
JOURNAL OF GEOPHYSICAL RESEARCH-ATMOSPHERES(2023)
摘要
Direct assimilation of Dual-frequency Precipitation Radar (DPR) data of the Global Precipitation Measurement (GPM) core satellite is challenging mainly due to its long revisiting intervals relative to the time scale of precipitation, and precipitation location errors. This study explores a method for improving precipitation forecasts using GPM DPR through model parameter estimation. We developed a 28 km mesh global atmospheric data assimilation system that integrates the Nonhydrostatic ICosahedral Atmospheric Model (NICAM) and Local Ensemble Transform Kalman Filter (LETKF) coupled with a satellite radar simulator. Using the NICAM-LETKF and GPM DPR observations, this study estimates a model cloud physics parameter corresponding to snowfall terminal velocity. To overcome the difficulties of long revisiting intervals and precipitation location errors, we propose a parameter estimation method based on a two-dimensional histogram known as the contoured frequency by temperature diagram (CFTD). Parameter estimation effectively mitigated the gap between simulated and observed CFTD, resulting in improved 6 hr precipitation forecasts.
更多查看译文
关键词
data assimilation,GPM DPR,parameter estimation,cloud microphysics,radar reflectivity
AI 理解论文
溯源树
样例
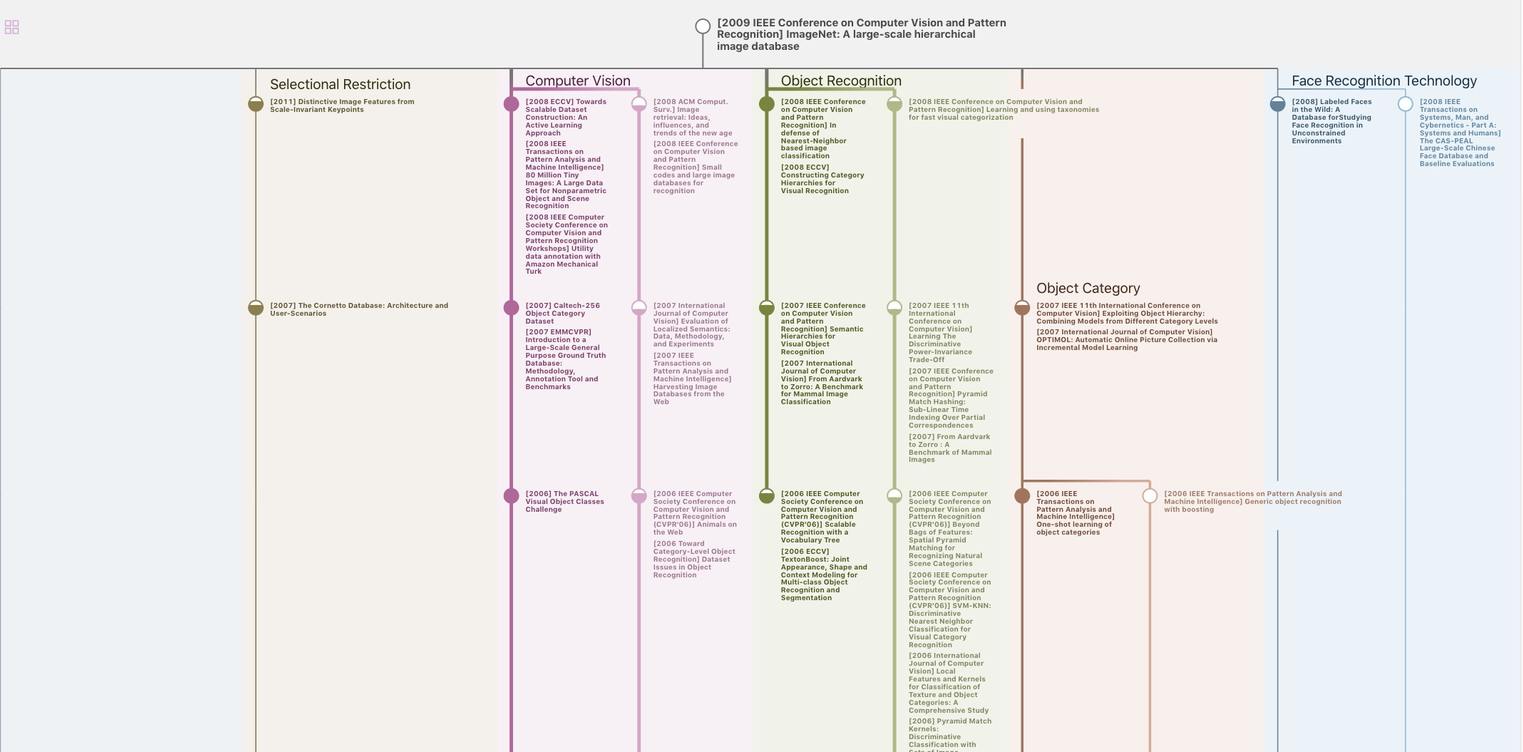
生成溯源树,研究论文发展脉络
Chat Paper
正在生成论文摘要