A vigilance estimation method for high-speed rail drivers using physiological signals with a two-level fusion framework
BIOMEDICAL SIGNAL PROCESSING AND CONTROL(2023)
摘要
The vigilance decrement of high-speed rail (HSR) drivers is one of the main factors contributing to accidents. It's necessary to develop an effective and reliable method to estimate HSR drivers' vigilance. Given that response speed is one of the most important capabilities of HSR drivers, the reaction time (RT) of signal detection would be an objective indicator of vigilance. We explored the relationship between RTs and self-reported Karolinska Sleepiness Scale (KSS) scores. And analyzed the change of electroencephalogram (EEG) and electrocardiogram (ECG) features with RTs. Then, a regression model with two-level fusion was proposed to continuously evaluate HSR drivers' vigilance by estimating their RTs. In the feature-level fusion, a convolutional neural network (CNN) module was used to fuse selected EEG and ECG. Then, EEG, ECG, and fused features were fed into three long short-term memory (LSTM) models to calculate HSR drivers' RTs in parallel. In the decision-level fusion, a threshold filter was performed on the sub-results of three long short-term memory (LSTM) models to calculate the weights, and the final result was fused with the sub-results with a linear regression (LR) model. Data of 30 HSR drivers in a simulated experiment were used to validate our proposed method. The mean absolute error (MAE), root mean square error (RMSE), and Pearson correlation coefficient (PCC) of our model were 80.13 ms, 109.42 ms, and 0.83, respectively. The results indicated that the estimation accuracy and reliability were improved significantly. This method would make some contributions to developing HSR driver vigilance monitoring systems.
更多查看译文
关键词
Vigilance,HSR driver,Information fusion,EEG,ECG,LSTM
AI 理解论文
溯源树
样例
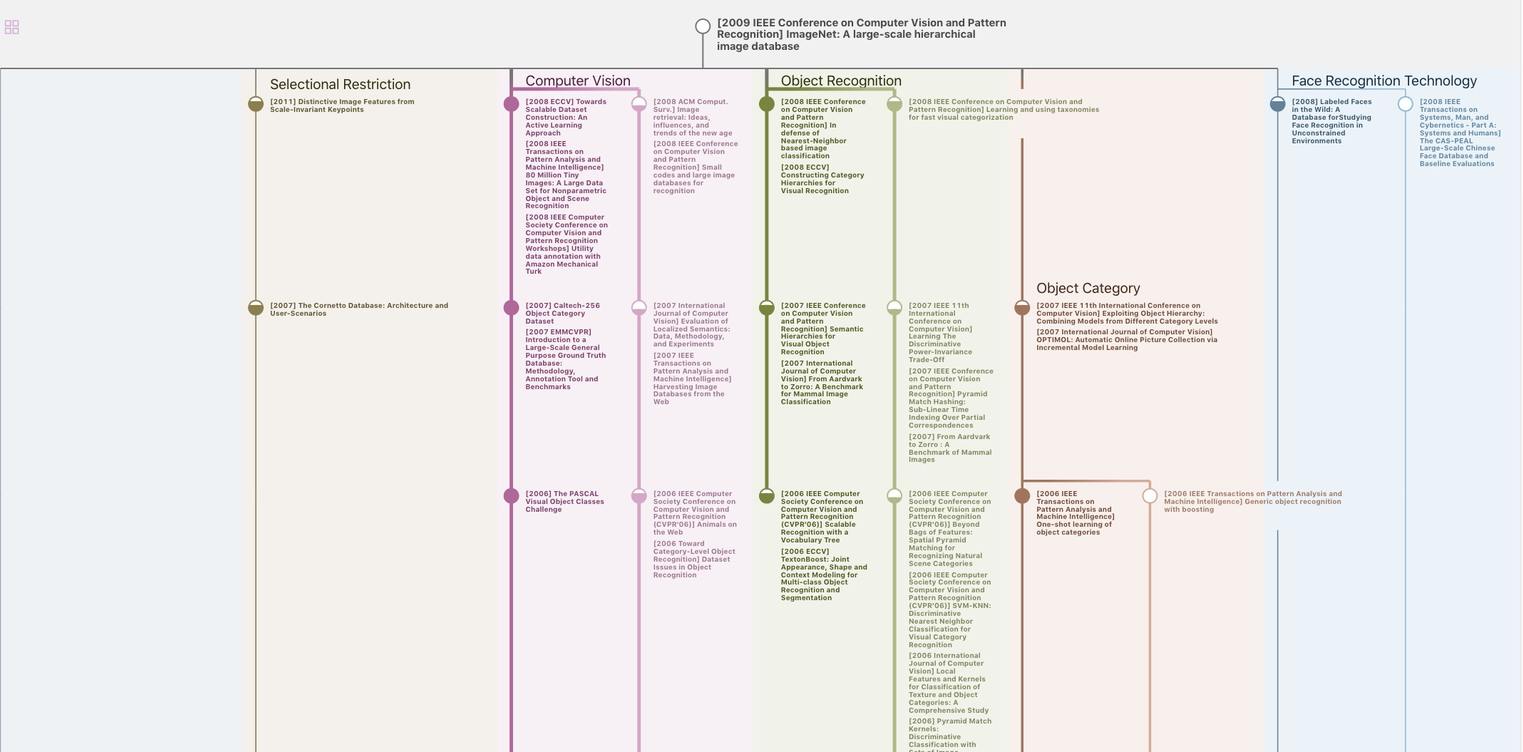
生成溯源树,研究论文发展脉络
Chat Paper
正在生成论文摘要