Optimality conditions for Tucker low-rank tensor optimization
COMPUTATIONAL OPTIMIZATION AND APPLICATIONS(2023)
摘要
Optimization problems with tensor variables are widely used in statistics, machine learning, pattern recognition, signal processing, computer vision, etc. Among these applications, the low-rankness of tensors is an intrinsic property that can help unearth potential but important structure or feature in the corresponding high-dimensional multi-way datasets, leading to the study on low-rank tensor optimization (LRTO for short). For the general framework of LRTO, little has been addressed in optimization theory. This motivates us to study the optimality conditions, with special emphasis on the Tucker low-rank constrained problems and the Tucker low-rank decomposition-based reformulations. It is noteworthy that all the involved optimization problems are nonconvex, and even discontinuous, due to the complexity of the tensor Tucker rank function or the multi-linear decomposition with the orthogonality or even group sparsity constraints imposed on factor matrices. By employing the tools in variational analysis, especially the normal cones to low-rank matrices and the properties of matrix manifolds, we propose necessary and/or sufficient optimality conditions for Tucker low-rank tensor optimization problems, which will enrich the context of the nonconvex and nonsmooth optimization.
更多查看译文
关键词
Tensor optimization,Optimality conditions,Tucker decomposition,Low-rankness
AI 理解论文
溯源树
样例
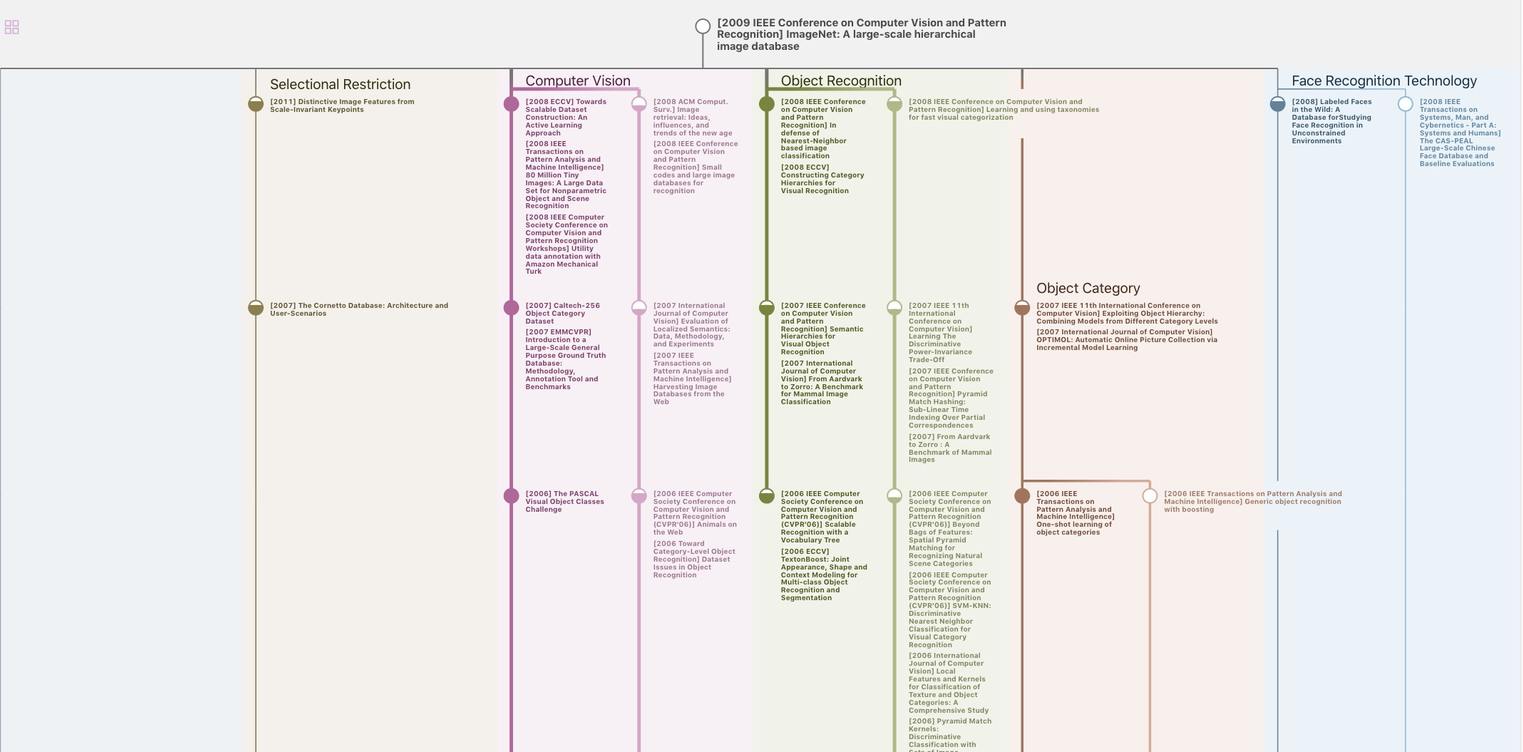
生成溯源树,研究论文发展脉络
Chat Paper
正在生成论文摘要