Cell Painting-based bioactivity prediction boosts high-throughput screening hit-rates and compound diversity
bioRxiv (Cold Spring Harbor Laboratory)(2023)
摘要
Efficiently identifying bioactive compounds towards a target of interest remains a time- and resource-intensive task in early drug discovery. The ability to accurately predict bioactivity using morphological profiles has the potential to rationalize the process, enabling smaller screens of focused compound sets. Towards this goal, we explored the application of deep learning with Cell Painting, a high-content image-based assay, for compound bioactivity prediction in early drug screening. Combining Cell Painting data and unrefined single-concentration activity readouts from high-throughput screening (HTS) assays, we investigated to what degree morphological profiles could predict compound activity across a set of 140 unique assays. We evaluated the performance of our models across different target classes, assay technologies, and disease areas. The predictive performance of the models was high, with a tendency for better predictions on cell-based assays and kinase targets. The average ROC-AUC was 0.744 with 62% of assays reaching ≥0.7, 30% reaching ≥0.8 and 7% reaching ≥0.9 average ROC-AUC, outperforming commonly used structure-based predictions in terms of predictive performance and compound structure diversity. In many cases, bioactivity prediction from Cell Painting data could be matched using brightfield images rather than multichannel fluorescence images. Experimental validation of our predictions in follow-up assays confirmed enrichment of active compounds. Our results suggest that models trained on Cell Painting data can predict compound activity in a range of high-throughput screening assays robustly, even with relatively noisy HTS assay data. With our approach, enriched screening sets with higher hit rates and higher hit diversity can be selected, which could reduce the size of HTS campaigns and enable primary screening with more complex assays.
更多查看译文
关键词
bioactivity prediction,compound diversity,painting-based,high-throughput,hit-rates
AI 理解论文
溯源树
样例
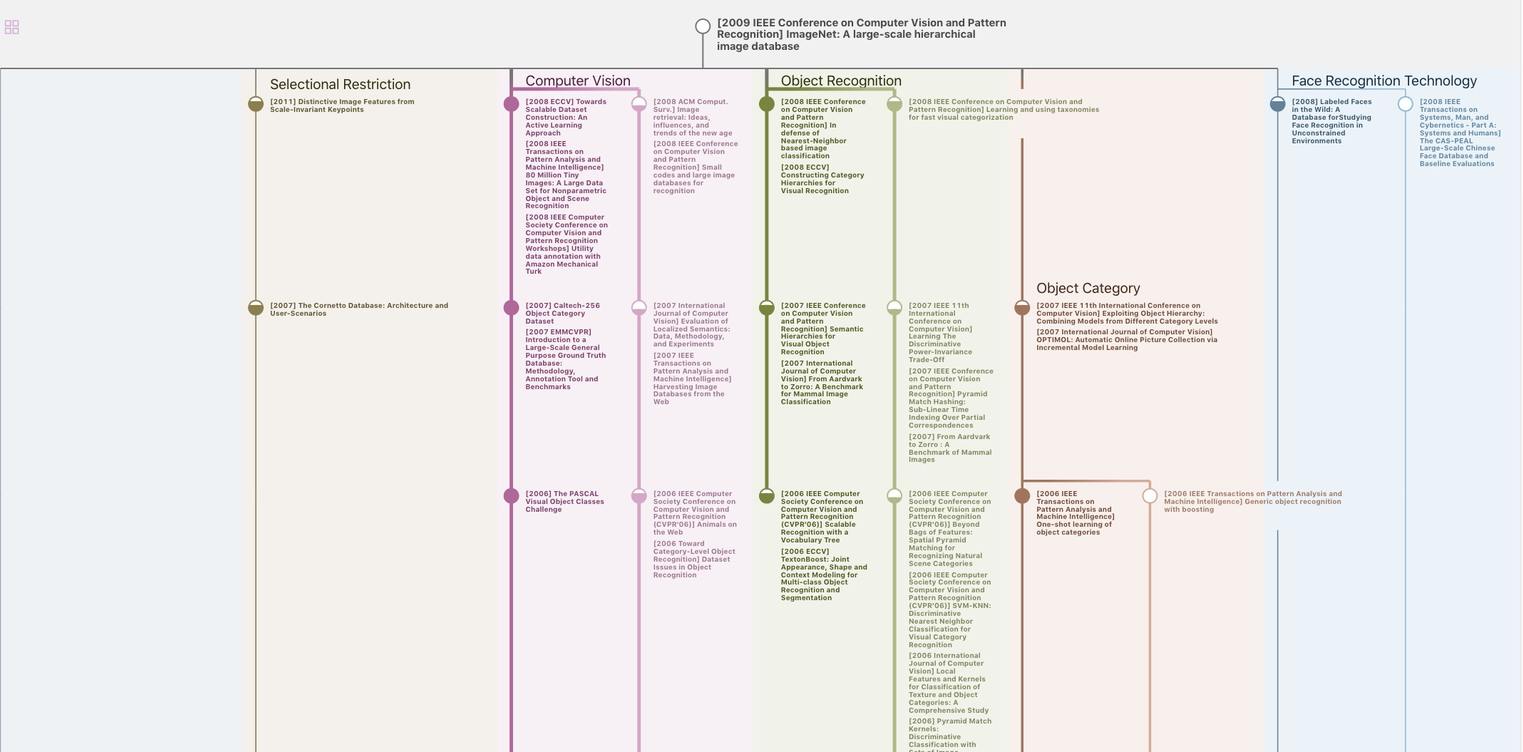
生成溯源树,研究论文发展脉络
Chat Paper
正在生成论文摘要