Unsupervised generalization of correlated quantum dynamics on disordered lattices
PHYSICAL REVIEW A(2023)
摘要
The application of unsupervised machine learning to the study of physical problems is an emerging field aimed at leveraging their outstanding capabilities to distill essential information from complex data sets. Here we use Generative Adversarial Networks (GANs), deep learning algorithms mainly used to synthesize realistic images, for the analysis of a physical problem: the propagation of interacting quantum particles on disordered chains, characterized by the emergence of complex quantum correlations. We find that GANs can learn these correlations and identify the physical control parameters in a completely unsupervised manner. After training on a data set of unlabeled examples, the algorithm can generate, without further calculations, a much larger number of unseen yet physically correct new instances. Most notably, the knowledge distilled in the algorithm's latent space identifies the relevant control parameter, the level of disorder in our case, even though this was not highlighted in the training set. This allows tuning the disorder level in the generated samples to values the algorithm was not explicitly trained on, thereby uncovering a fundamental physical phenomenon-the crossover from nearly free propagation to localization. These results demonstrate the ability of deep neural networks to learn and generalize physical rules by scanning examples and suggest their use in physical analysis and discovery.
更多查看译文
关键词
quantum dynamics,unsupervised generalization
AI 理解论文
溯源树
样例
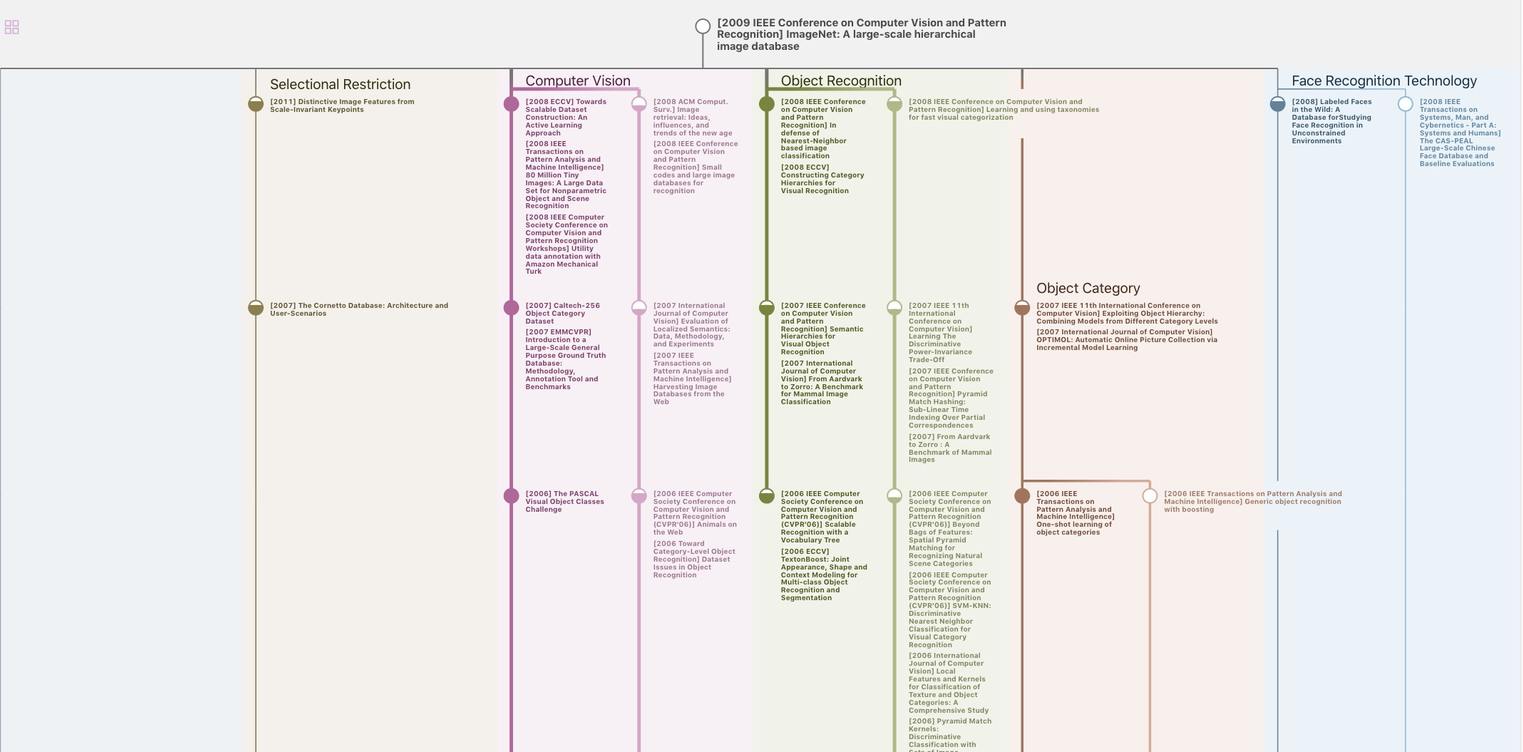
生成溯源树,研究论文发展脉络
Chat Paper
正在生成论文摘要