A graph-powered large-scale fraud detection system
International Journal of Machine Learning and Cybernetics(2024)
Abstract
Graph-powered fraud detection is a common issue in various areas, such as e-commerce, banking, insurance and social networks, where data can be naturally formulated as graph structure. Especially in e-commerce, due to its large scale and enormous amount of real-time transactions over millions of merchandises, fraud detection has become an important and serious problem. The challenges lie in three aspects: sparse fraud samples, complex features in online transactions and extra-large scale of e-commerce data. To deal with above issues, in this paper, we propose an efficient graph-powered large-scale fraud detection framework. Concretely, we first present a heterogeneous label propagation algorithm to recall more potentially fraudulent samples for further model training; then, we design a novel multi-view heterogeneous graph neural network model to obtain more accurate fraud predictions; finally, a fraud pattern analysis approach is presented to discover hidden fraud groups. In addition, in order to improve the efficiency and scalability of our proposed fraud detection framework, we present a large-scale fraud detection system deployed on a general graph computing engine. We conduct experiments on two real-world datasets. Results show that the proposed graph-powered fraud detection framework achieves high accuracy and superior scalability on large-scale graph data.
MoreTranslated text
Key words
Fraud detection,E-commerce,Scalable computing,Label propagation,Graph neural network
AI Read Science
Must-Reading Tree
Example
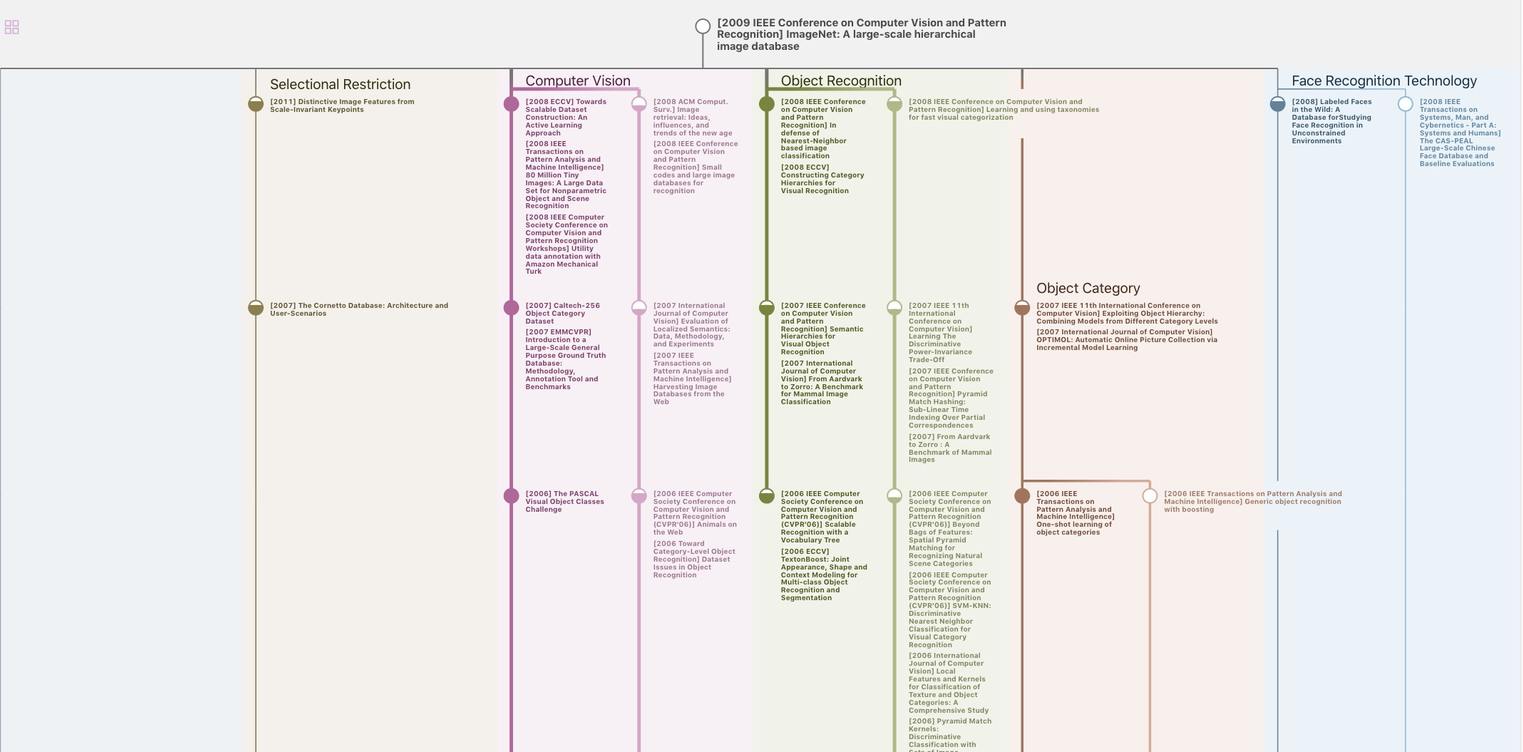
Generate MRT to find the research sequence of this paper
Chat Paper
Summary is being generated by the instructions you defined