Explainable Models for Multivariate Time-series Defect Classification of Arc Stud Welding
INTERNATIONAL JOURNAL OF PROGNOSTICS AND HEALTH MANAGEMENT(2023)
摘要
Arc Stud Welding (ASW) is widely used in many indus-tries such as automotive and shipbuilding and is employed in building and jointing large-scale structures. While defec-tive or imperfect welds rarely occur in production, even a single low-quality stud weld is the reason for scrapping the entire structure, financial loss, and wasting time. Preventive machine learning-based solutions can be leveraged to mini-mize the loss. However, these approaches only provide pre-dictions rather than demonstrating insights for characterizing defects and root cause analysis. In this work, an investigation of defect detection and classification to diagnose the possi-ble leading causes of low-quality defects is proposed. More-over, an explainable model to describe network predictions is explored. Initially, a dataset of multi-variate time series of ASW utilizing measurement sensors in an experimental en-vironment is generated. Next, a set of techniques to leverage synthetic measurements, reference, and residual signals, and generate a residue dataset, are proposed. Finally, the archi-tecture of classification models is optimized and by Bayesian black-box optimization methods to maximize their perfor-mance. Our best approach reaches an F1 score of 0.84 on the test set. Furthermore, an explainable model is employed to provide interpretations on per class feature attention of the model to extract sensor measurement contribution in detect-ing defects as well as its time attention.
更多查看译文
关键词
Time Series,Arc Stud Welding,Machine Learning,Deep Learning,Defect Classification,ICA,Explainable AI,Bayesian Optimization,Root Cause,Data-Centric AI
AI 理解论文
溯源树
样例
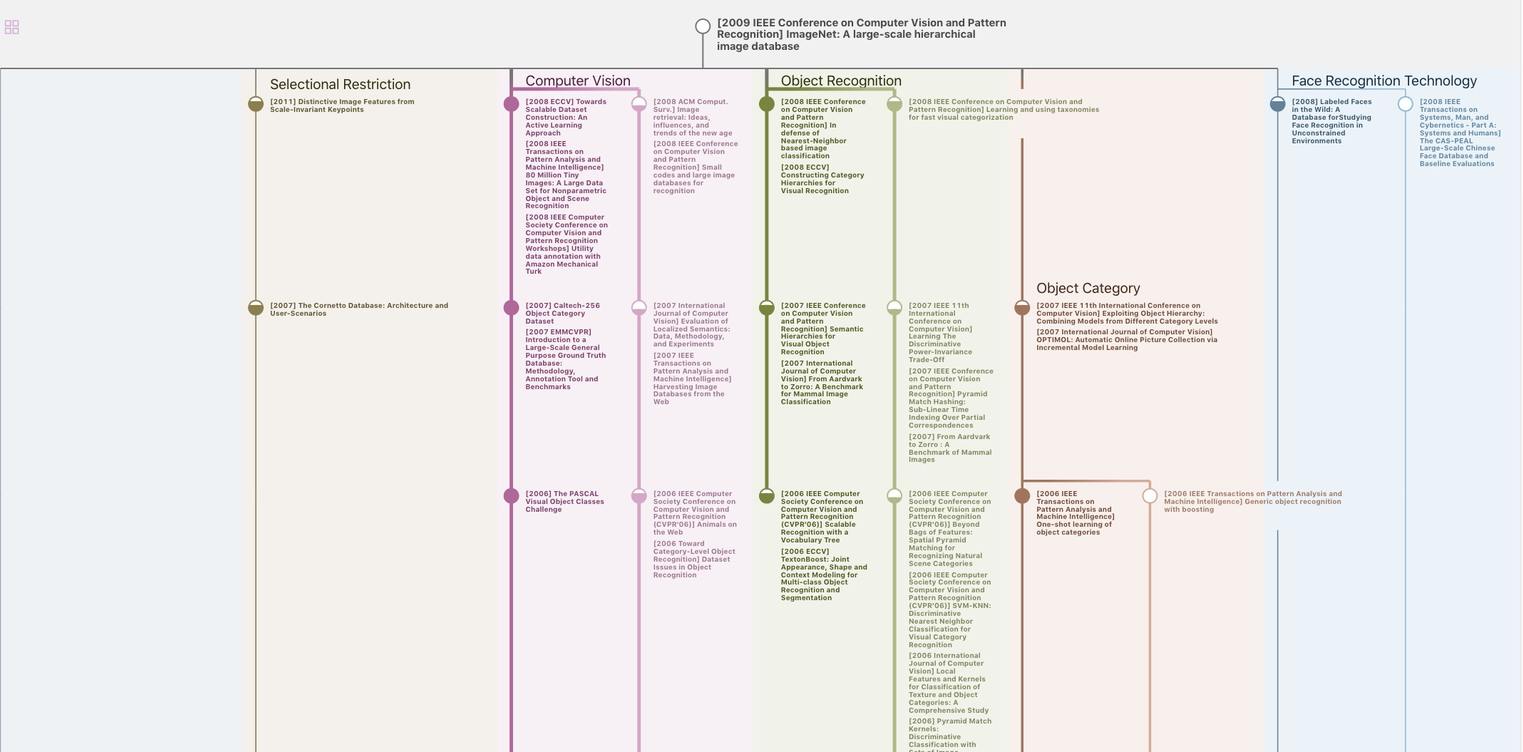
生成溯源树,研究论文发展脉络
Chat Paper
正在生成论文摘要