Multi-centers SoftMax reciprocal average precision loss for deep metric learning
NEURAL COMPUTING & APPLICATIONS(2023)
摘要
Deep metric learning (DML) aims to learn the semantic embeddings with deep convolutional neural networks (DCNNs) that the similar instances are closer than different ones. Despite the success in the past decades, one drawback of metric learning approaches is that they are mostly driven by minimizing distances. Thus they remain lacking in awareness of the importance of shifting ranking orders which is essential in rank-based metric tasks. Moreover, in real-world scenarios, images of the same class always have several local clusters rather than a single one, e.g., cars from different perspectives and birds of different postures. Therefore, this work proposes multi-centers SoftMax reciprocal average precision loss (mcSAP) to jointly supervise the learning of DCNNs by SoftMax with multi-centers and a ranking-based metric loss. Firstly, we reformulate SoftMax to learn multiple sub-centers for each class to capture more local centroids for modeling intra-class variance in real-world data. Then, we introduce mcSAP loss that enhances the capacity on improving the order of positive instances at high ranks rather than those only at low ranks. And it is meaningful for obtaining a more optimally structured feature space with higher purity around the center. Moreover, we created Cattle-2022 dataset, consisting of 10,076 images from 246 identities of Chinese Simmental and Holstein for supporting DML tasks and even cattle identification. To validate the proposed mcSAP loss, it is comprehensively compared with several well-known losses on the benchmark fine-grained datasets, as CUB-2011, Cars196, Stanford online products (SOP) and our proposed Cattle-2022 dataset. It is encouraging to find that our approach outperforms the state-of-the-art models in both image retrieval and clustering benchmarks.
更多查看译文
关键词
Deep metric learning (DML),Deep convolutional neural networks (DCNNs),Fine-grained classification,Average precision
AI 理解论文
溯源树
样例
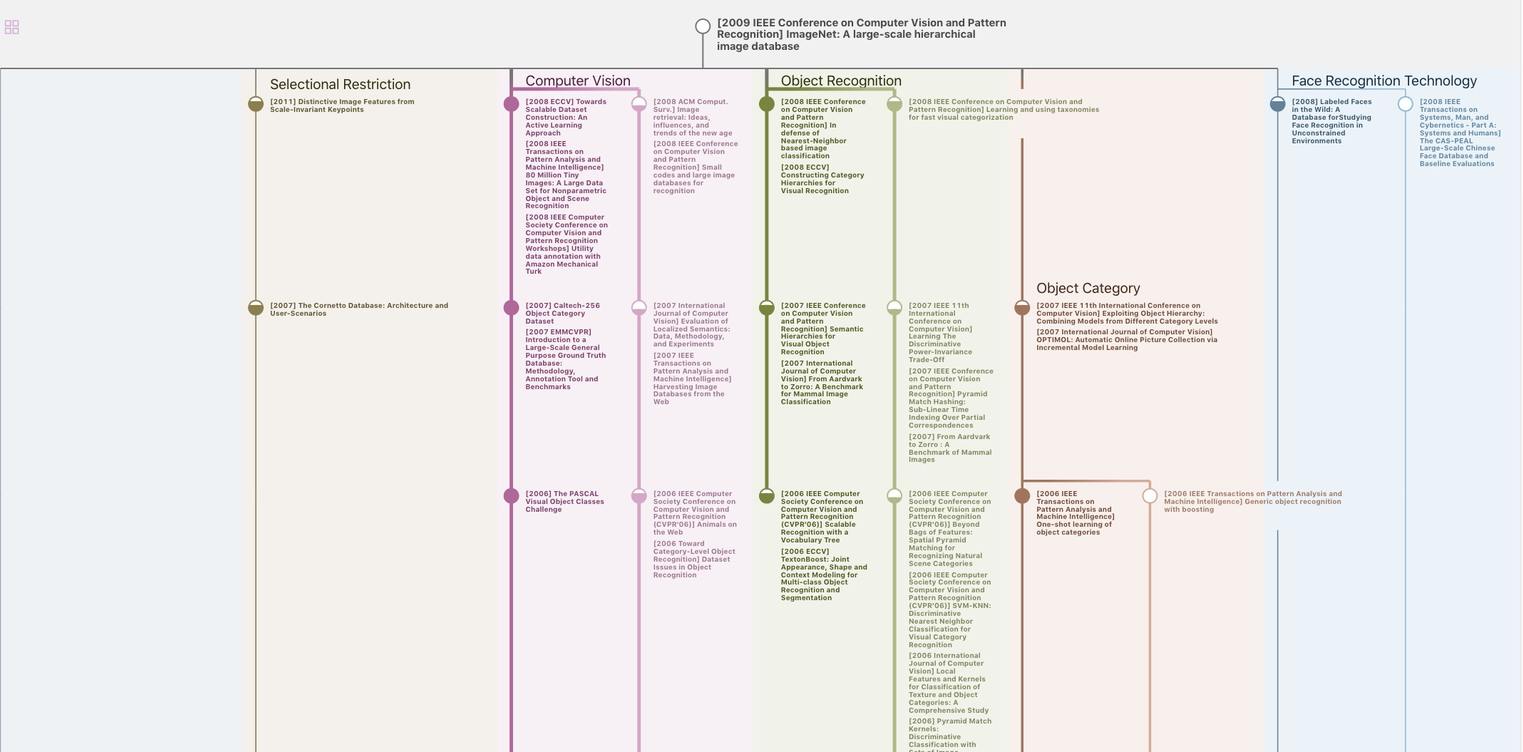
生成溯源树,研究论文发展脉络
Chat Paper
正在生成论文摘要