Detection and Correction of Abnormal IoT Data from Tea Plantations Based on Deep Learning
AGRICULTURE-BASEL(2023)
摘要
This paper proposes a data anomaly detection and correction algorithm for the tea plantation IoT system based on deep learning, aiming at the multi-cause and multi-feature characteristics of abnormal data. The algorithm is based on the Z-score standardization of the original data and the determination of sliding window size according to the sampling frequency. First, we construct a convolutional neural network (CNN) model to extract abnormal data. Second, based on the support vector machine (SVM) algorithm, the Gaussian radial basis function (RBF) and one-to-one (OVO) multiclassification method are used to classify the abnormal data. Then, after extracting the time points of abnormal data, a long short-term memory network is established for prediction with multifactor historical data. The predicted values are used to replace and correct the abnormal data. When multiple consecutive abnormal values are detected, a faulty sensor judgment is given, and the specific faulty sensor location is output. The results show that the accuracy rate and micro-specificity of abnormal data detection for the CNN-SVM model are 3-4% and 20-30% higher than those of the traditional CNN model, respectively. The anomaly detection and correction algorithm for tea plantation data established in this paper provides accurate performance.
更多查看译文
关键词
tea plantation,deep learning,data feature extraction,data correction
AI 理解论文
溯源树
样例
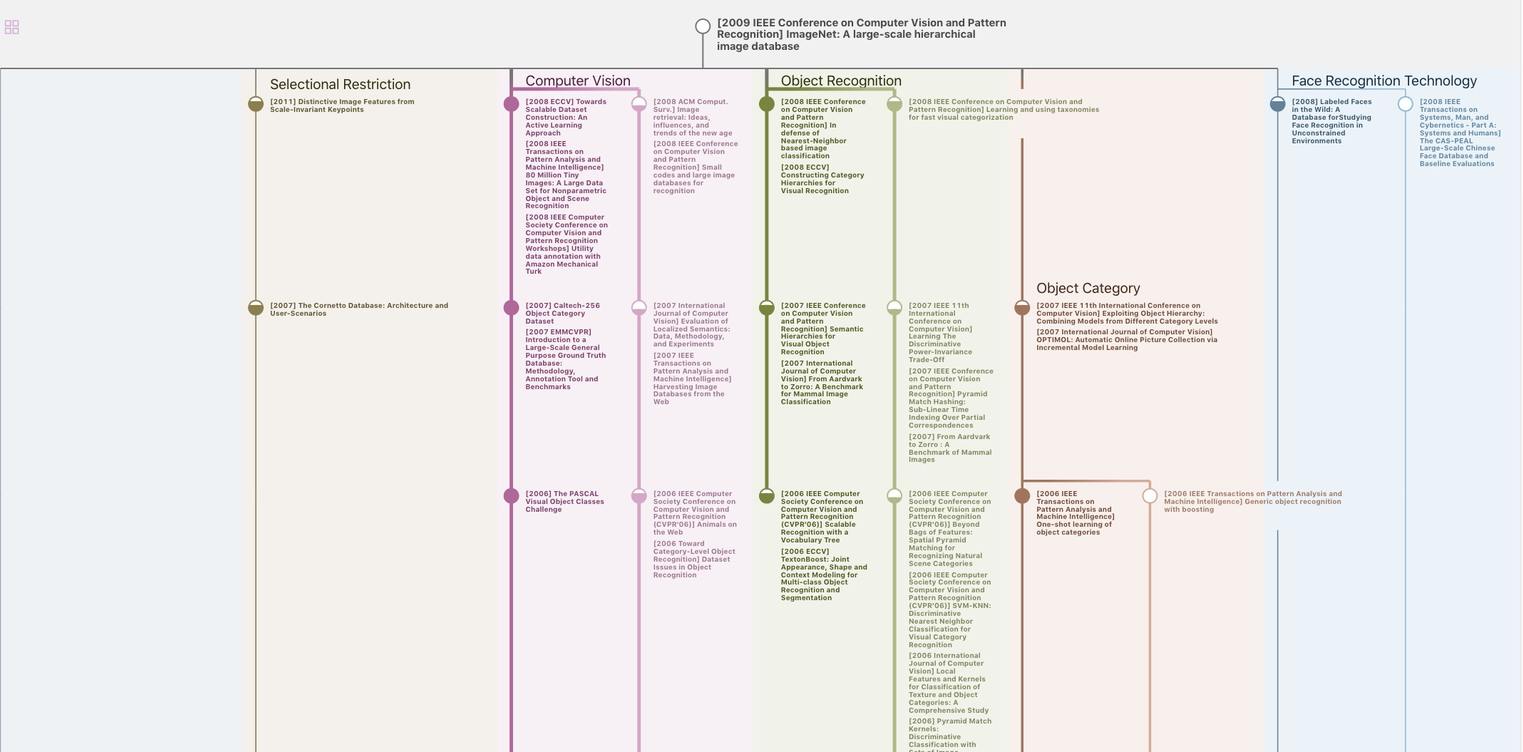
生成溯源树,研究论文发展脉络
Chat Paper
正在生成论文摘要