Spatial Autocorrelation of Global Stock Exchanges Using Functional Areal Spatial Principal Component Analysis
MATHEMATICS(2023)
摘要
This work focuses on functional data presenting spatial dependence. The spatial autocorrelation of stock exchange returns for 71 stock exchanges from 69 countries was investigated using the functional Moran's I statistic, classical principal component analysis (PCA) and functional areal spatial principal component analysis (FASPCA). This work focuses on the period where the 2015-2016 global market sell-off occurred and proved the existence of spatial autocorrelation among the stock exchanges studied. The stock exchange return data were converted into functional data before performing the classical PCA and FASPCA. Results from the Monte Carlo test of the functional Moran's I statistics show that the 2015-2016 global market sell-off had a great impact on the spatial autocorrelation of stock exchanges. Principal components from FASPCA show positive spatial autocorrelation in the stock exchanges. Regional clusters were formed before, after and during the 2015-2016 global market sell-off period. This work explored the existence of positive spatial autocorrelation in global stock exchanges and showed that FASPCA is a useful tool in exploring spatial dependency in complex spatial data.
更多查看译文
关键词
functional data analysis,spatial autocorrelation,principal component analysis,stock market
AI 理解论文
溯源树
样例
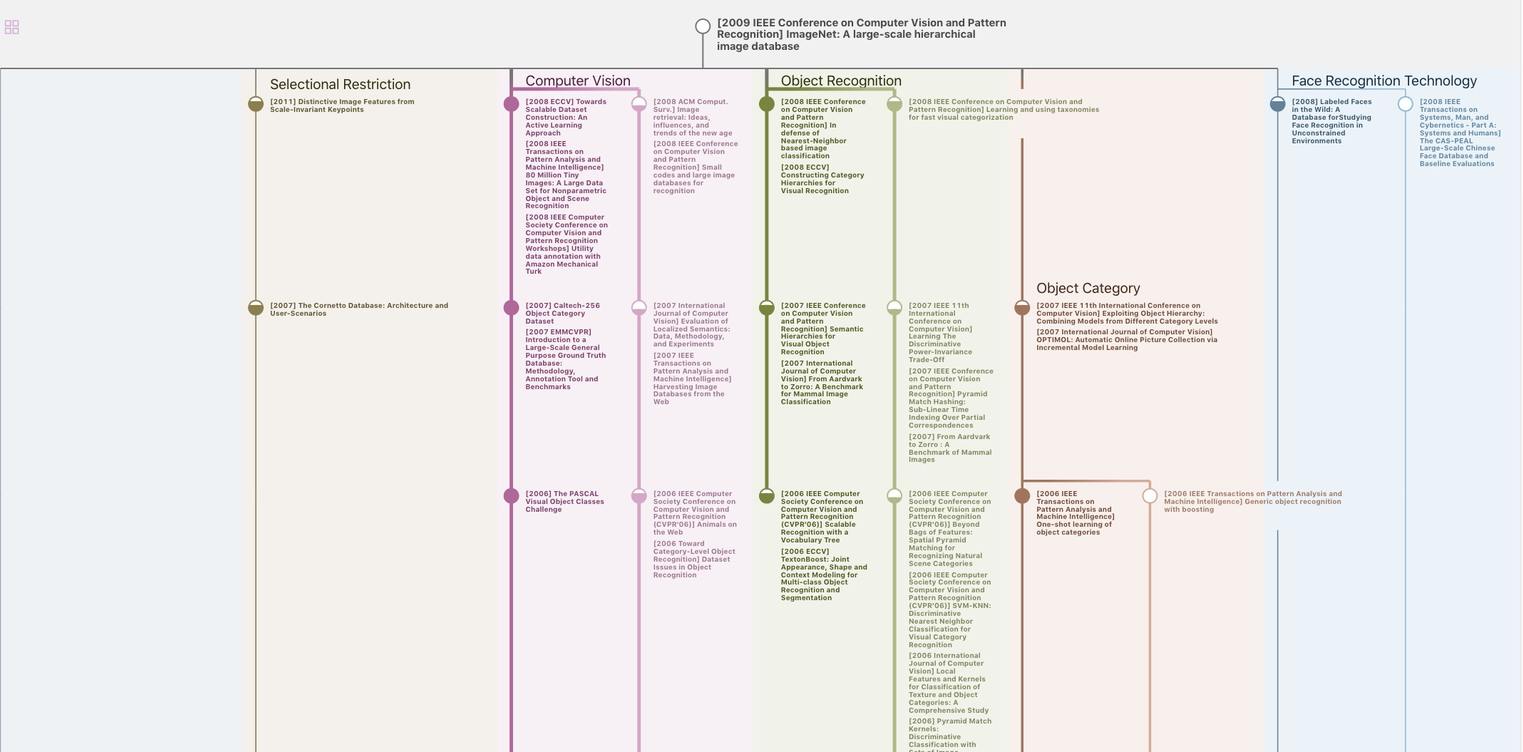
生成溯源树,研究论文发展脉络
Chat Paper
正在生成论文摘要