Next-night disrupted sleep prediction from clinician notes using weakly supervised learning models
CRITICAL CARE MEDICINE(2023)
Abstract
Introduction: Disrupted sleep frequently occurs in ICU adults but is poorly recognized and documented. Earlier prediction of disrupted sleep may facilitate timely sleep improvement efforts. We developed and evaluated weakly-supervised learning models to automatically predict next-night disrupted sleep in ICU adults using notes from the prior night. Methods: The dataset included ICU night-2 EHR notes (RN 21-8h; MD 21-11h) for adults admitted ≥4 days to 1 of 13 ICUs between 1/17 and 6/21 at 2 academic centers. Subsequent ICU admissions were excluded. A sample of 30 ICU RNs and MDs were surveyed to identify the terms they most often use to document disrupted (poor) and non-disrupted (good) ICU sleep in the EHR. Sleep quality pseudo-labels for ICU night-3, a night where ICU disrupted sleep prevalence is likely greater than nights-1 or 2, were created by searching for disrupted/non-disrupted sleep terms in the RN/MD EHR notes. Pseudo-label agreement and learning model performance were validated against a manual review of 100 night-3 clinical notes and data by a trained ICU researcher. In the absence of a disrupted sleep note, disrupted sleep was deemed to be present when ≥1 RASS score ≥0 was documented between 24-6h that was not found to be related to a bedside procedure/assessment. The absence of both a note and a 24-6h RASS score was documented as non-disrupted sleep. We trained models to predict ICU night-3 disrupted sleep based on notes from ICU night-2 using the night-3 pseudo-labels. Results: Among the 5953 patients (60.3% medical; 50.6% intubated), 56.6% had a total of 5415 night-3 disrupted sleep notes [MD 79.4%; RN 20.6%]. The night-3 manual validation identified disrupted sleep in 43/100 patients. The night-3 pseudo-labels achieved a precision of 0.53, a recall of 0.70, and an F1-score of 0.60. The night-2 to night-3 disrupted sleep prediction model achieved a precision of 0.45, a recall of 0.88, an F1-score of 0.59 and an AUC of 0.56. Conclusions: Although clinicians documented disrupted sleep in more than half the patients, our preliminary model was unable to reliably predict the onset of disrupted sleep. Promising avenues for future research include the use of strategies (e.g., RASS scores, formalized sleep assessment methods) to better train prediction models.
MoreTranslated text
Key words
sleep prediction,weakly supervised learning models,clinician notes,next-night
AI Read Science
Must-Reading Tree
Example
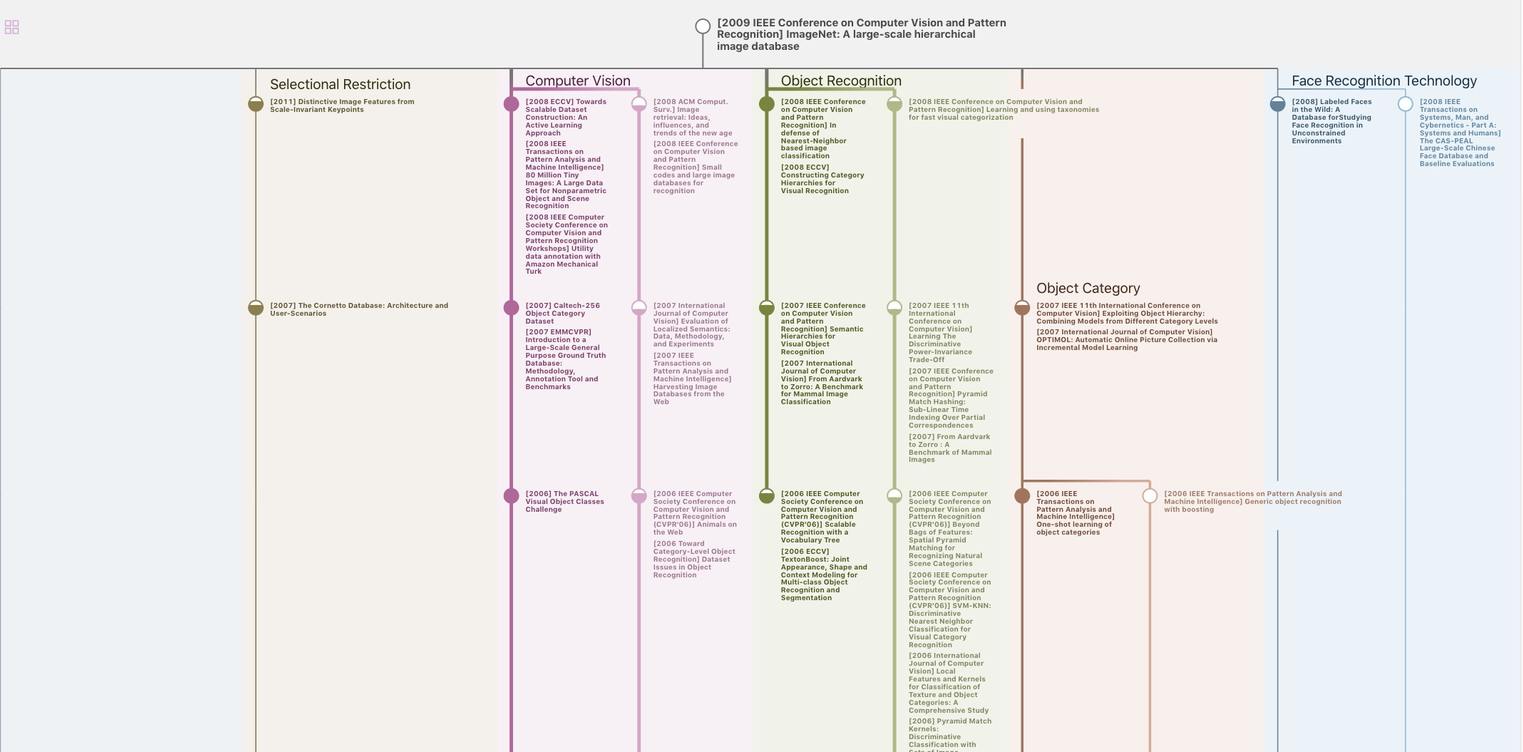
Generate MRT to find the research sequence of this paper
Chat Paper
Summary is being generated by the instructions you defined