Semantic Segmentation of In-Vehicle Point Cloud With Improved RangeNet plus plus Loss Function
IEEE ACCESS(2023)
Abstract
To solve the problem of inaccurate object segmentation caused by unbalanced samples for in-vehicle point cloud, an improved semantic segmentation network RangeNet++ based on asymmetric loss function (AsL-RangeNet++) is proposed, which uses asymmetric loss (AsL) function and Adam optimizer to calculate and adjust object weights, achieve optimal point cloud segmentation. AsL-RangeNet++ can solve the problem of unbalance between positive and negative samples and label error in multi-label classification by calculating the weights of positive and negative samples respectively and more accurately segments the point cloud of small targets. A large number of experiments on the widely used SemanticKITTI dataset show that the proposed method has higher segmentation accuracy and better adaptability than the current mainstream methods.
MoreTranslated text
Key words
Semantic segmentation,Neural networks,rangenet plus plus,asymmetric loss function,in-vehicle point cloud
AI Read Science
Must-Reading Tree
Example
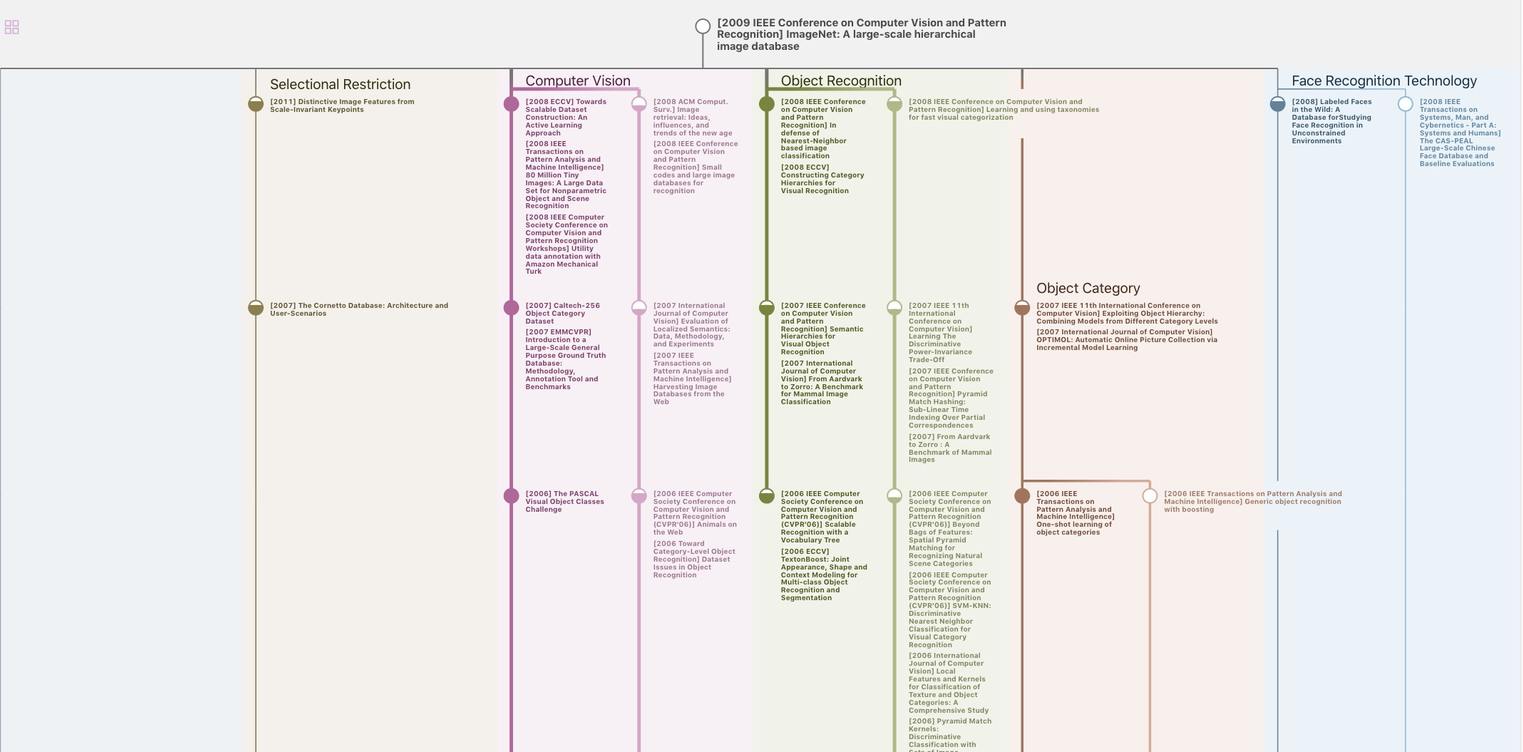
Generate MRT to find the research sequence of this paper
Chat Paper
Summary is being generated by the instructions you defined