Low-Illumination Image Enhancement Based on Deep Learning Techniques: A Brief Review
PHOTONICS(2023)
摘要
As a critical preprocessing technique, low-illumination image enhancement has a wide range of practical applications. It aims to improve the visual perception of a given image captured without sufficient illumination. Conventional low-illumination image enhancement methods are typically implemented by improving image brightness, enhancing image contrast, and suppressing image noise simultaneously. Nevertheless, recent advances in this area are dominated by deep-learning-based solutions, and consequently, various deep neural networks have been proposed and applied to this field. Therefore, this paper briefly reviews the latest low-illumination image enhancement, ranging from its related algorithms to its unsolved open issues. Specifically, current low-illumination image enhancement methods based on deep learning are first sorted out and divided into four categories: supervised learning methods, unsupervised learning methods, semi-supervised learning methods, and zero-shot learning methods. Then, existing low-light image datasets are summarized and analyzed. In addition, various quality assessment indices for low-light image enhancement are introduced in detail. We also compare 14 representative algorithms in terms of both objective evaluation and subjective evaluation. Finally, the future development trend of low-illumination image enhancement and its open issues are summarized and prospected.
更多查看译文
关键词
deep learning,low-illumination image enhancement,Retinex theory,quality evaluation index,image dataset
AI 理解论文
溯源树
样例
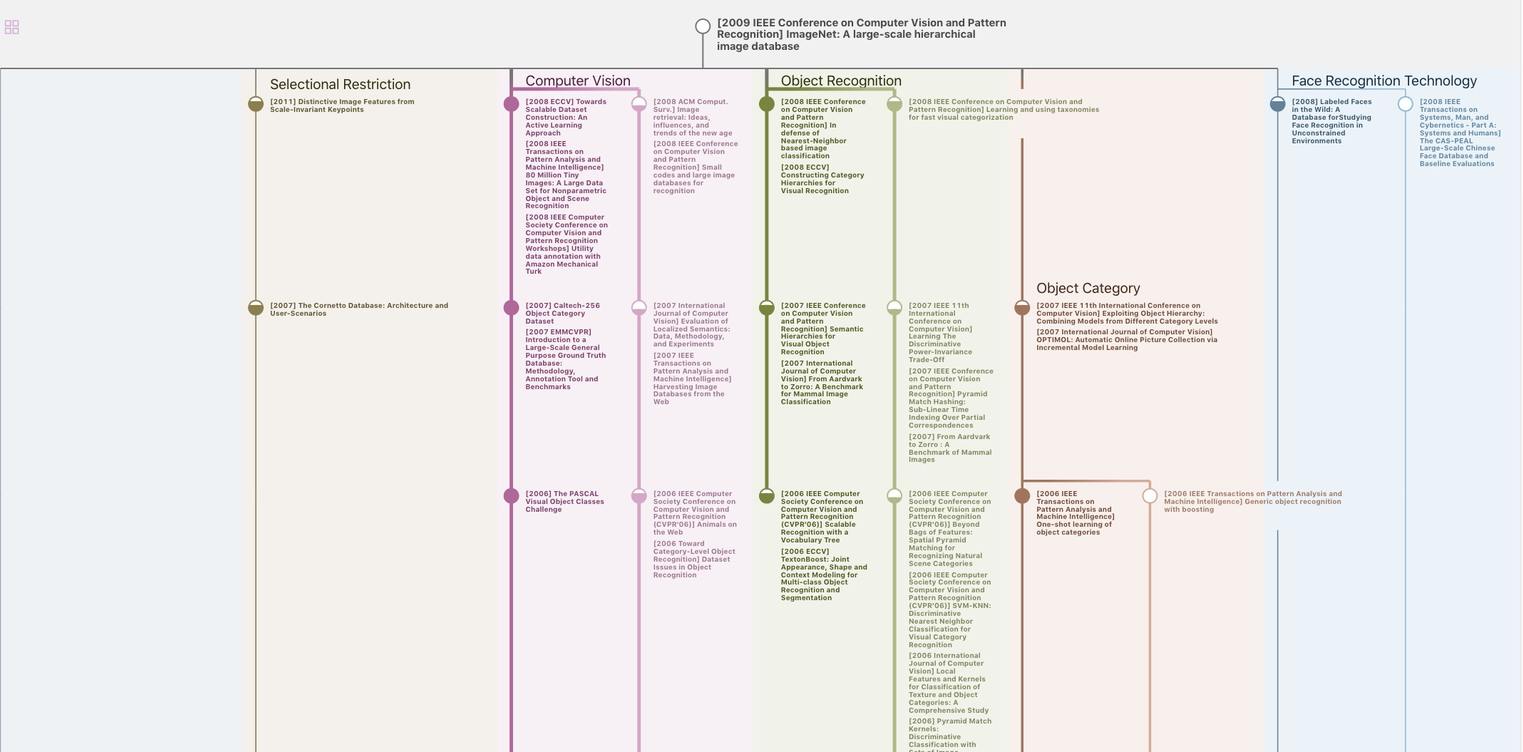
生成溯源树,研究论文发展脉络
Chat Paper
正在生成论文摘要