A serial attention module-based deep convolutional neural network for mixed Gaussian-impulse removal
IET IMAGE PROCESSING(2023)
摘要
The removal of mixed noise is a challenging task because the attenuation of the noise distribution cannot be described precisely. The coupling of additive white Gaussian noise and impulse noise (IN) is a typical case. At present, most methods use a two-phase strategy, that is, IN detection coupled with additive white Gaussian noise removal, often leading to poor denoising results with an increase in the ratio of IN. In this paper, an effective convolutional neural network (CNN) model is proposed, namely a serial attention module-based CNN (SACNN), for mixed noise removal. In contrast to the existing two-phase methods, SACNN unifies the denoising process into a single CNN framework. In SACNN, residual learning and batch normalization are used to train the model, which speeds up the convergence and improves the mixed noise removal performance. Meanwhile, the serial attention module is applied to better preserve the texture details. The experimental results reveal that SACNN achieves superior quality metrics and visual appearance when compared to several leading approaches.
更多查看译文
关键词
batch normalization,convolutional neural network,serial attention module
AI 理解论文
溯源树
样例
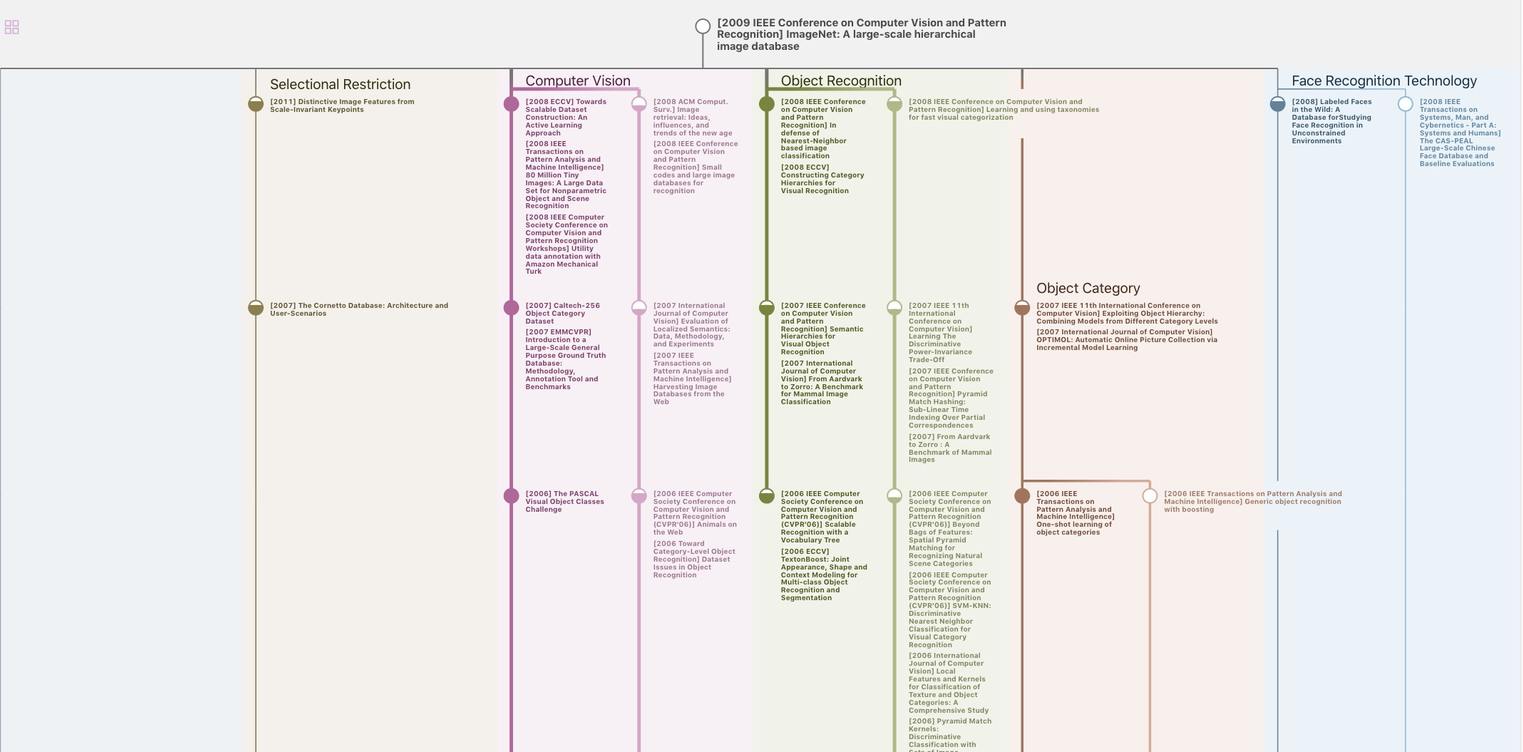
生成溯源树,研究论文发展脉络
Chat Paper
正在生成论文摘要