SkinNet-ENDO: Multiclass skin lesion recognition using deep neural network and Entropy-Normal distribution optimization algorithm with ELM
INTERNATIONAL JOURNAL OF IMAGING SYSTEMS AND TECHNOLOGY(2023)
摘要
The early diagnosis of skin cancer through clinical methods reduces the human mortality rate. The manual screening of dermoscopic images is not an efficient procedure; therefore, researchers working in the domain of computer vision employed several algorithms to classify the skin lesion. The existing computerized methods have a few drawbacks, such as low accuracy and high computational time. Therefore, in this work, we proposed a novel deep learning and Entropy-Normal Distribution Optimization Algorithm with extreme learning machine (NDOEM)-based architecture for multiclass skin lesion classification. The proposed architecture consists of five fundamental steps. In the first step, two contrast enhancement techniques including hybridization of mathematical formulation and convolutional neural network are implemented prior to data augmentation. In the second step, two pre-trained deep learning models, EfficientNetB0 and DarkNet19, are fine-tuned and retrained through the transfer learning. In the third step, features are extracted from the fine-tuned models and later the most discriminant features are selected based on novel Entropy-NDOELM algorithm. The selected features are finally fused using a parallel correlation technique in the fourth step to generate the result feature vectors. Finally, the resultant features are again down-sampled using the proposed algorithm and the resultant features are passed to the extreme learning machine (ELM) for the final classification. The simulations are conducted on three publicly available datasets as HAM10000, ISIC2018, and ISIC2019 to achieving an accuracy of 95.7%, 96.3%, and 94.8% respectively.
更多查看译文
关键词
classification,contrast enhancement,deep learning,Entropy-NDOELM,hybridization,skin cancer
AI 理解论文
溯源树
样例
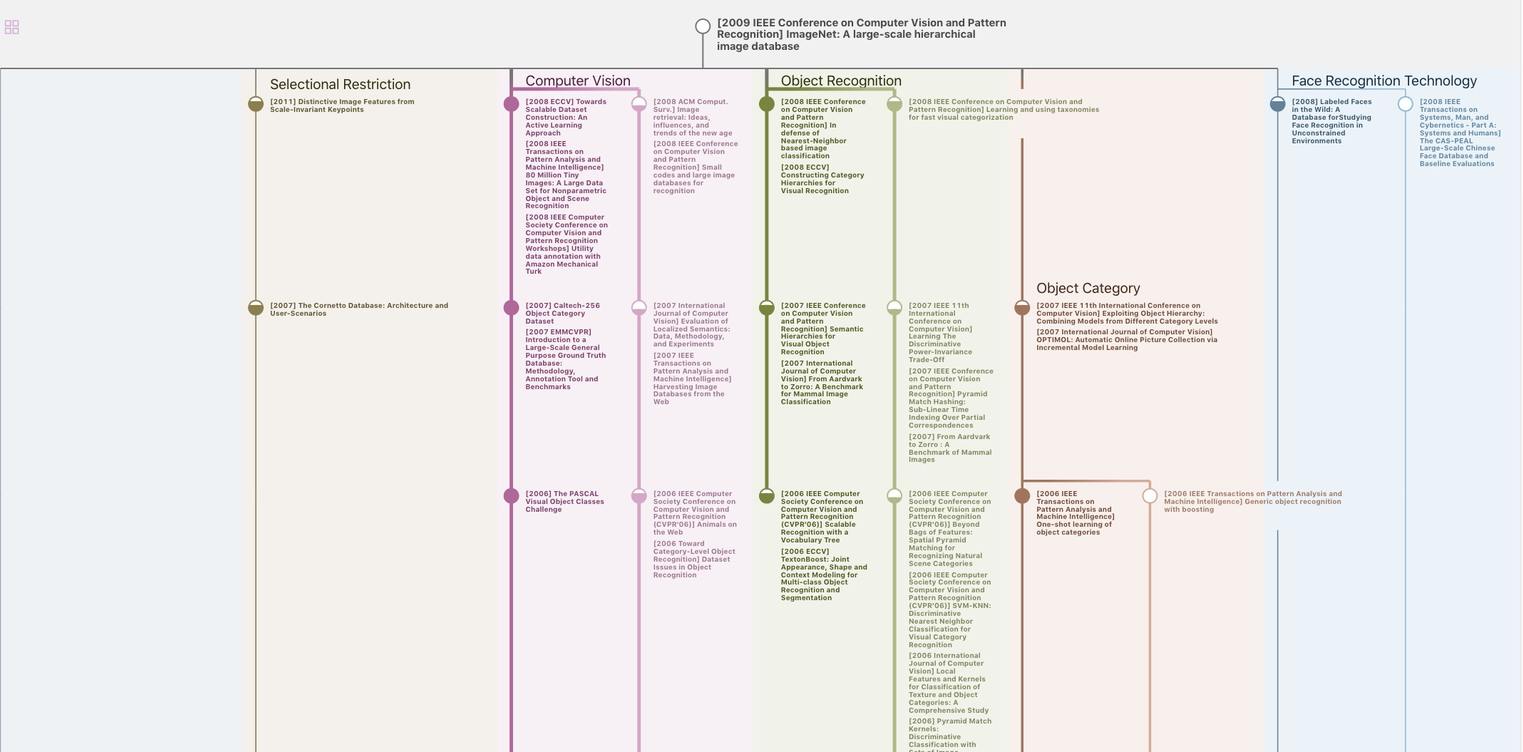
生成溯源树,研究论文发展脉络
Chat Paper
正在生成论文摘要